How do pricing models for AI ad generators affect the success of your ugc ads? As advertisers increasingly turn to these platforms, understanding diverse pricing strategies is critical. This article will explore the basics of AI ad generator pricing models, analyze popular models like freemium and subscription-based options, and compare variations across key industry players. By engaging with this content, readers will gain insights into how pricing impacts advertising revenue and how to select the right saas pricing strategy for their needs, ultimately leading to more effective and profitable advertising campaigns.
Key Takeaways
- AI ad generators streamline advertising by automating the creation and delivery of compelling content
- Subscription-based models provide predictable pricing and consistent access to advanced advertising features
- Pay-per-click strategies focus on maximizing ROI through direct user engagement and budget control
- Performance-based pricing links costs to campaign effectiveness, promoting a results-driven relationship with advertisers
- Advanced analytics enhance campaign insights, enabling adaptive strategies that align with market trends
Understanding the Basics of AI Ad Generator Pricing Models
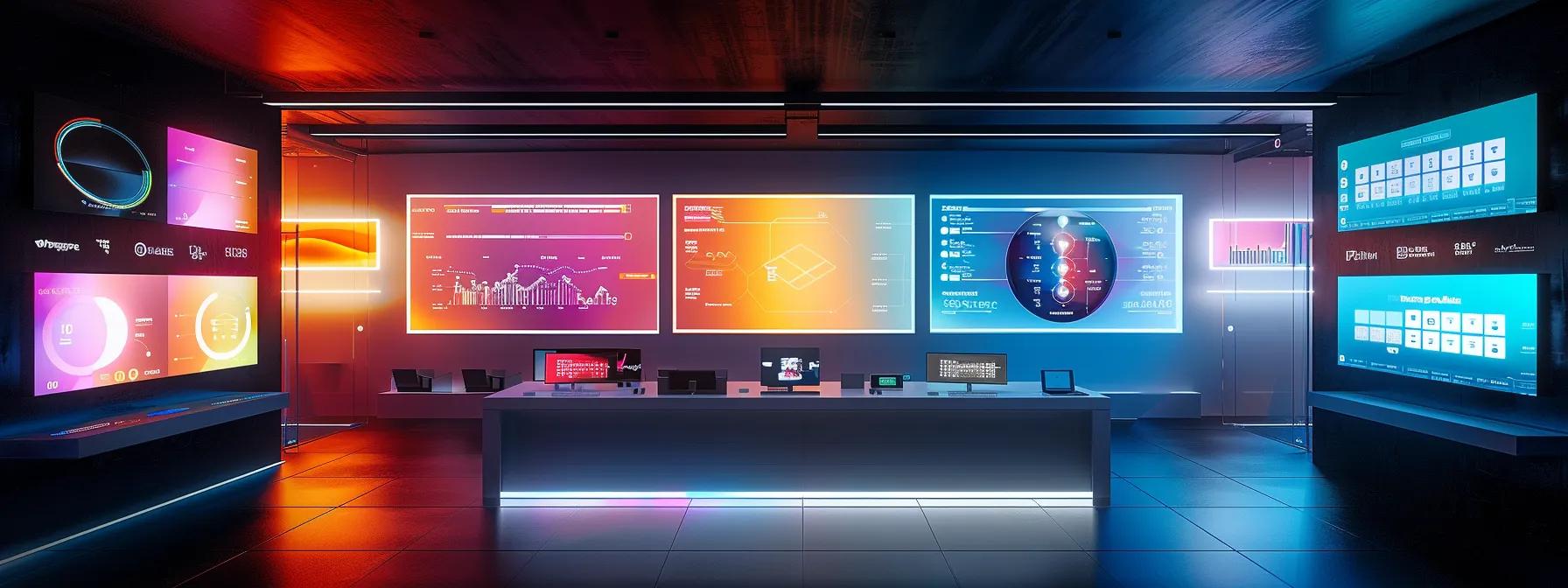
AI ad generators are tools designed to simplify the process of creating compelling advertising campaigns by leveraging advanced technology, including ugc ads. Key factors influencing pricing strategies include penetration pricing to attract new users and flat-rate pricing models that cater to different usages, alongside considerations for resources such as disk storage. The upcoming sections will explore these aspects in greater depth.
Defining AI Ad Generators and Their Purpose
AI ad generators utilize machine learning algorithms to automate the creation of advertisements, allowing businesses to streamline their marketing efforts. These tools analyze data and trends to produce compelling ad content that can enhance overall engagement. The average return on investment from campaigns employing AI ad generators often reflects their ability to maintain high-quality advertisements with reduced time and cost obligations.
The purpose of AI ad generators extends beyond mere efficiency; they empower marketers to leverage data-driven insights to refine their pricing strategies and target audiences accurately. By harnessing these tools, companies can bolster their reputation in the market while maximizing the value of their advertising spend. This combination of performance and adaptability underscores the importance of understanding the various pricing models available among major industry players.
Key Factors Influencing Pricing Strategies
Pricing strategies for AI ad generators are significantly influenced by the functionality offered by the software as a service (SaaS) model. In North America, some providers opt for subscription-based pricing, allowing users access to a suite of features for a consistent monthly cash outlay. This model appeals to businesses seeking predictable expenses while benefiting from regular updates and support, ultimately enhancing their advertising effectiveness.
Another key consideration is the product‘s market positioning and competitive landscape. Companies must evaluate their target audience and the value their AI ad generator delivers in comparison to competitors. This understanding drives pricing decisions tailored to meet specific needs and expectations, ensuring that their offering remains attractive within the diverse market of digital advertising solutions.
Understanding the basics of AI ad generator pricing prepares one for deeper insights. Now, let’s look closely at how major players structure their pricing models.
Analyzing Popular Pricing Models Among Major Players
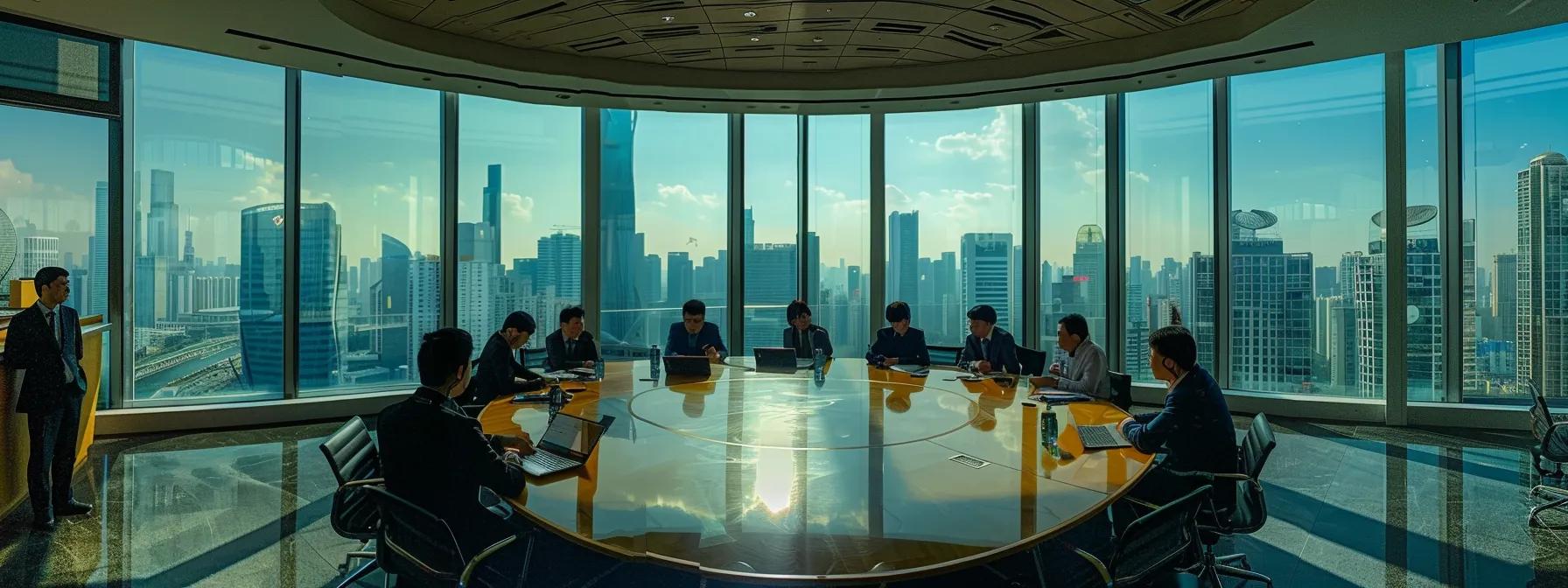
Analyzing Popular Pricing Models Among Major Players
This section examines the various pricing models adopted by prominent AI ad generator providers in the realm of social media marketing. It begins with a review of subscription-based models used by industry leaders, followed by a comparative analysis of pay-per-click versus pay-per-impression strategies. Additionally, performance-based pricing will be explained, offering insights into how these approaches influence marketing strategy and their effectiveness in targeting specific markets.
Subscription-Based Models Used by Industry Leaders
Subscription-based pricing models have become a cornerstone for many leading AI ad generator providers, primarily due to their ability to adapt to the fluctuating demand for advertising solutions. These models often signal a commitment to long-term relationships with clients, who benefit from unbroken access to updated tools and features. For example, a company might choose a tiered subscription allowing for an incremental percentage increase in capabilities based on their needs, enhancing brand awareness through consistent message delivery and maximum exposure.
Furthermore, subscription-based models simplify budget management for businesses while providing insights into user engagement and performance metrics. By utilizing these tools, advertisers can effectively analyze their campaign effectiveness, leading to better-informed decisions about marketing strategies. This concept of predictable pricing not only alleviates financial uncertainty but also contributes to improved brand consistency across platforms, addressing a common pain point among marketers seeking reliable solutions that enhance their advertising impact.
Pay-Per-Click vs. Pay-Per-Impression: A Comparative Analysis
Pay-per-click (PPC) and pay-per-impression (PPI) models present distinct approaches for advertisers utilizing AI ad generators. In a PPC framework, marketers pay only when a user clicks on their ad, making this model attractive for those aiming to maximize return on investment through direct engagement. This pricing strategy inherently relates to the concept of price elasticity of demand, as advertisers can adjust their bids based on the anticipated click-through rate, allowing for a more controlled workflow and effective budget management.
Conversely, the pay-per-impression model charges advertisers based on the number of times an ad is displayed, regardless of user interaction. This method can be beneficial for businesses that prioritize brand visibility and awareness, especially when launching new campaigns through search engines or chatbots. By employing virtual machines, companies can analyze performance metrics and optimize ad placements, enhancing their overall marketing strategy while better understanding audience behavior and impressions.
- Overview of Pay-Per-Click Model
- Advantages of Pay-Per-Click for Budget Management
- Understanding Pay-Per-Impression Model
- Benefits of Pay-Per-Impression for Brand Visibility
- Using Virtual Machines for Performance Analysis
Performance-Based Pricing Explained
Performance-based pricing represents a strategic approach where costs are linked directly to the effectiveness of the advertising campaigns delivered through an AI ad generator system. This model allows advertisers to pay based on tangible results, such as conversions or clicks, rather than a fixed fee, thus enhancing the overall value proposition. For instance, an advertiser might opt for a dynamic pricing strategy that adjusts based on their IP address and user behavior, ensuring budget efficiency in search advertising by targeting specific demographic profiles effectively.
This pricing structure aligns the goals of the users and the ad service provider, promoting a results-driven relationship. By focusing on performance metrics, companies can optimize their campaigns based on real-time data, allowing for immediate adjustments. Such adaptability not only improves engagement rates but also fosters a better understanding of audience preferences and behaviors, leading to more impactful advertising outcomes.
- Overview of Subscription-Based Models
- Comparative Analysis of Pay-Per-Click vs. Pay-Per-Impression
- Performance-Based Pricing Explained
- Benefits of Effective Budget Management
- Maximizing Brand Visibility through Strategic Pricing
As companies set their prices, each choice tells a story. Next, the differences across various platforms reveal more about their strategies and appeal.
Comparing Pricing Variations Across Different Platforms
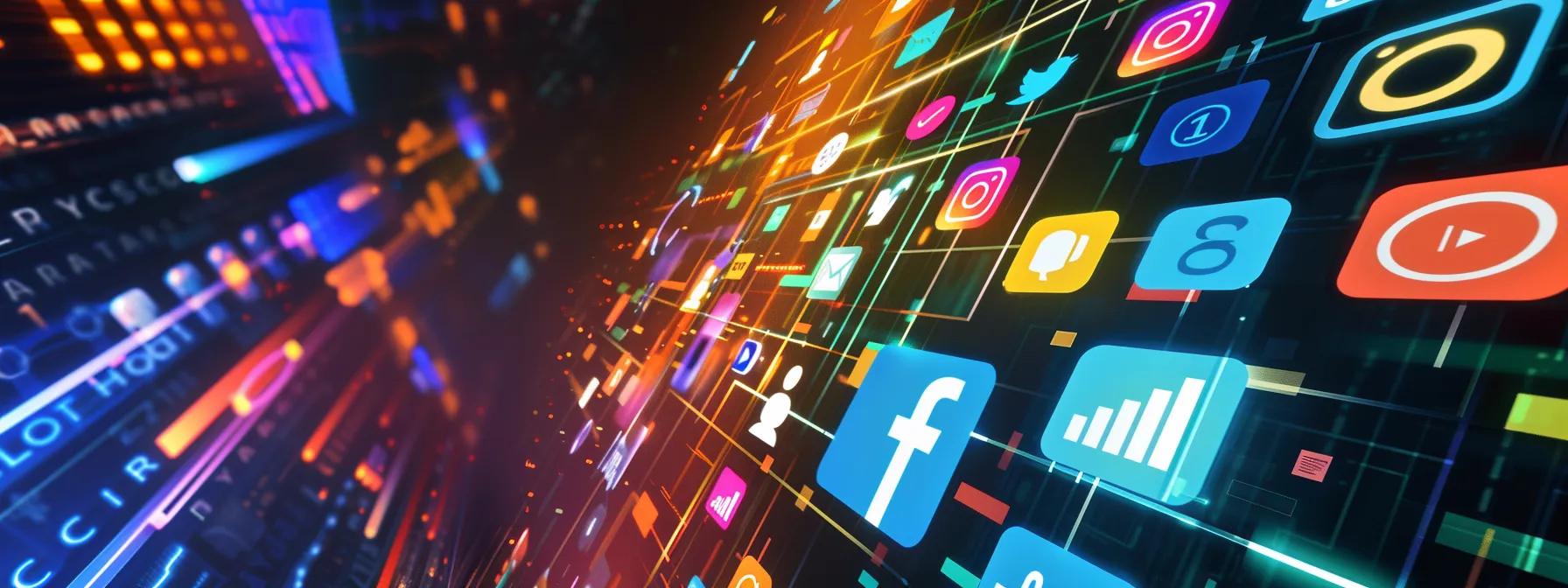
This section evaluates pricing variations across key platforms that offer AI ad generation services. It begins with Google Ads, detailing its unique pricing structure, followed by an exploration of Facebook Ads and their cost mechanisms. LinkedIn Ads will be examined to provide insights into professional targeting costs, alongside a look at other emerging platforms and their innovative pricing strategies, incorporating aspects such as psychological pricing and resource allocation for optimal ad performance.
Google Ads: Understanding Its Unique Pricing Structure
Google Ads employs a highly dynamic pricing structure that emphasizes innovation and scalability, allowing advertisers to customize their campaigns according to specific goals and budget constraints. This platform relies heavily on data analysis, enabling businesses to engage in thorough research to determine the most effective bidding strategies. By utilizing real-time insights, advertisers can make informed decisions that directly influence their profit margin, ultimately enhancing their marketing efforts.
The unique pricing model of Google Ads incorporates elements such as cost-per-click (CPC) and cost-per-thousand impressions (CPM), providing flexibility in campaign management. Advertisers benefit from the ability to adjust bids based on competitive positioning and user engagement metrics, ensuring their strategies remain effective over time. This approach not only facilitates responsive advertising but also allows businesses to tailor their campaigns, optimizing outcomes based on performance and user behavior.
Facebook Ads: Exploring Their Cost Mechanisms
Facebook Ads utilize a dynamic cost structure that significantly influences content marketing strategies. Advertisers can choose between different pricing mechanisms, such as cost per mille (CPM) or cost per click (CPC), allowing them to align their spending with specific campaign objectives. By leveraging advanced tools that incorporate natural language processing, businesses can optimize their ad targeting, thus minimizing risk and enhancing the effectiveness of their advertising spend.
The platform’s sophisticated algorithm manages latency concerns by delivering ads to users when engagement likelihood is highest. Advertisers need to remain vigilant about performance analytics, which offer insights into how ad spending translates into viewer interaction. Understanding these cost mechanisms is critical for marketers aiming to maximize the impact of their budgets, ensuring they achieve agility in adjusting strategies based on real-time performance data:
- Dynamic cost structure of Facebook Ads
- Pricing options: CPM vs. CPC
- Role of natural language processing in ad targeting
- Managing latency to enhance engagement
- Importance of performance analytics in decision-making
LinkedIn Ads: An Insight Into Professional Targeting Costs
LinkedIn Ads feature a pricing structure that emphasizes professional targeting, aligning with the specific needs of businesses. This platform utilizes a usage-based pricing model that allows advertisers to directly correlate their advertising costs with the engagement and visibility achieved among targeted users. By closely monitoring customer behavior, companies can optimize their campaigns to reach their ideal audience effectively, enhancing user experience through precise targeting.
Advertisers can select various bidding options, such as cost per click (CPC) or cost per impression (CPM), enabling them to choose the most suitable method based on their campaign objectives. Understanding these options is critical as they directly impact how ads are displayed and interacted with on the platform. This strategic approach not only elevates brand visibility but also maximizes the effectiveness of advertising budgets, ensuring that every dollar spent contributes to optimal campaign performance:
- Usage-based pricing models adapt to user engagement.
- Importance of understanding customer behavior.
- Flexibility with CPC and CPM bidding strategies.
- Enhancing user experience through targeted ads.
- Maximizing brand visibility with effective budgeting.
Other Emerging Platforms and Their Pricing Innovations
Emerging platforms are increasingly adopting innovative pricing models that cater to shifting consumer demands and enhance competitive positioning within the advertising landscape. For instance, some companies are integrating performance metrics directly into their pricing strategies, allowing businesses to adjust costs based on real-time engagement analytics. This approach not only reflects a commitment to consumer needs but also emphasizes the intelligence behind targeting strategies that can lead to greater brand visibility.
Moreover, a number of new market entrants are exploring tier-based pricing systems that align closely with manufacturing scalability, where charges adjust according to the volume of ads produced or the level of customization required. This flexibility empowers advertisers to strategically manage their budgets while ensuring their initiatives remain adaptive to market trends. By focusing on leadership in pricing innovation, these platforms can effectively address the complexities of audience engagement, thus fostering stronger connections with their consumers.
Prices vary as the sun rises and sets. Next, it is time to look beyond the numbers and see what true value these offers bring.
Evaluating Value Propositions in Relation to Pricing
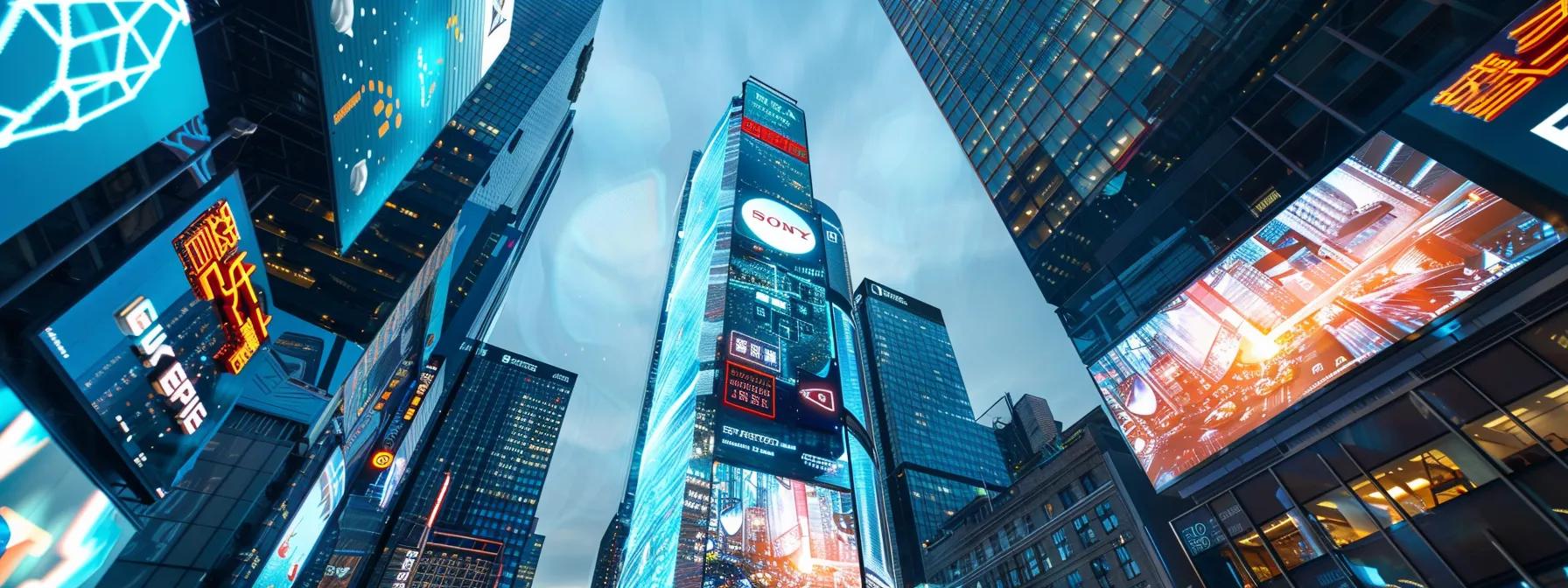
Evaluating the value propositions associated with AI ad generators involves assessing the return on investment (ROI) for various platforms, considering features and capabilities that affect costs, and understanding how audience targeting impacts pricing. These elements play a significant role in determining the total cost of ownership while influencing revenue and market share dynamics. A close examination of tools like Microsoft Azure for cloud storage reveals how these factors can shape advertising strategies across different industry players.
Assessing ROI for Different Platforms
Assessing the return on investment (ROI) for various platforms that utilize AI ad generators involves a thorough examination of the underlying SaaS pricing models. These models can significantly affect overall costs and the effectiveness of advertising strategies. Advertisers aim to evaluate their preferences regarding pricing structures, which can lead to improved inventory management and a better understanding of how analytics influence spending. By analyzing the ROI, advertisers can discern the true value of their chosen platform in terms of engagement, conversions, and revenue generation.
Different platforms offer distinct pricing strategies that can either enhance or limit the potential for achieving strong ROI. For instance, platforms employing flexible pricing based on performance metrics allow advertisers to optimize their budget in response to real-time analytics. As businesses navigate their options, understanding the nuances of price variations and how they relate to campaign outcomes will help in making informed decisions. This strategic awareness can lead to maximizing the return on advertising investments and drive continued growth in marketing effectiveness.
Features and Capabilities: How They Affect Costs
The features and capabilities of AI ad generators play a critical role in determining pricing structures within the online advertising landscape. An algorithm that effectively analyzes customer engagement data can significantly enhance campaign performance, allowing businesses to target their ads more precisely. As such, platforms offering advanced features may adopt a higher pricing model, reflecting the added value these functionalities provide to advertisers.
Furthermore, the choice of pricing options often hinges on the specific units of measurement used to assess ad effectiveness. For example, platforms that link costs to tangible outcomes such as conversions or impressions are able to justify their pricing based on quantifiable customer interactions. This approach not only helps advertisers understand the return on their investment but also empowers them to optimize their strategies more effectively.
The Role of Audience Targeting in Pricing
Audience targeting plays a significant role in shaping the pricing models of AI ad generators. Effective targeting requires a thorough understanding of customer demographics and behavior, facilitating the development of more tailored advertising strategies. This is where sentiment analysis becomes crucial; by analyzing customer feedback and emotions, businesses can account for preferences, leading to more precise predictions about the effectiveness of their ad campaigns and the corresponding license costs.
Pricing models that emphasize audience targeting often justify their costs based on the advanced capabilities they offer. For instance, platforms that utilize predictive analytics can adjust pricing based on how likely a target audience is to engage with an advertisement. This adaptive approach not only enhances the overall value proposition but also addresses the common pain point of optimizing marketing budgets, ensuring that advertisers receive measurable results from their investments:
- Importance of audience targeting in pricing strategies
- Utilization of sentiment analysis for effective engagement
- Role of predictive analytics in pricing adjustments
- Balancing license costs with audience engagement
- Maximizing marketing budgets through targeted advertising
Value is clearer when aligned with user needs. The journey into pricing choices will reveal how these needs shape the landscape of business decisions.
Examining User Intent and Business Needs in Pricing Choices
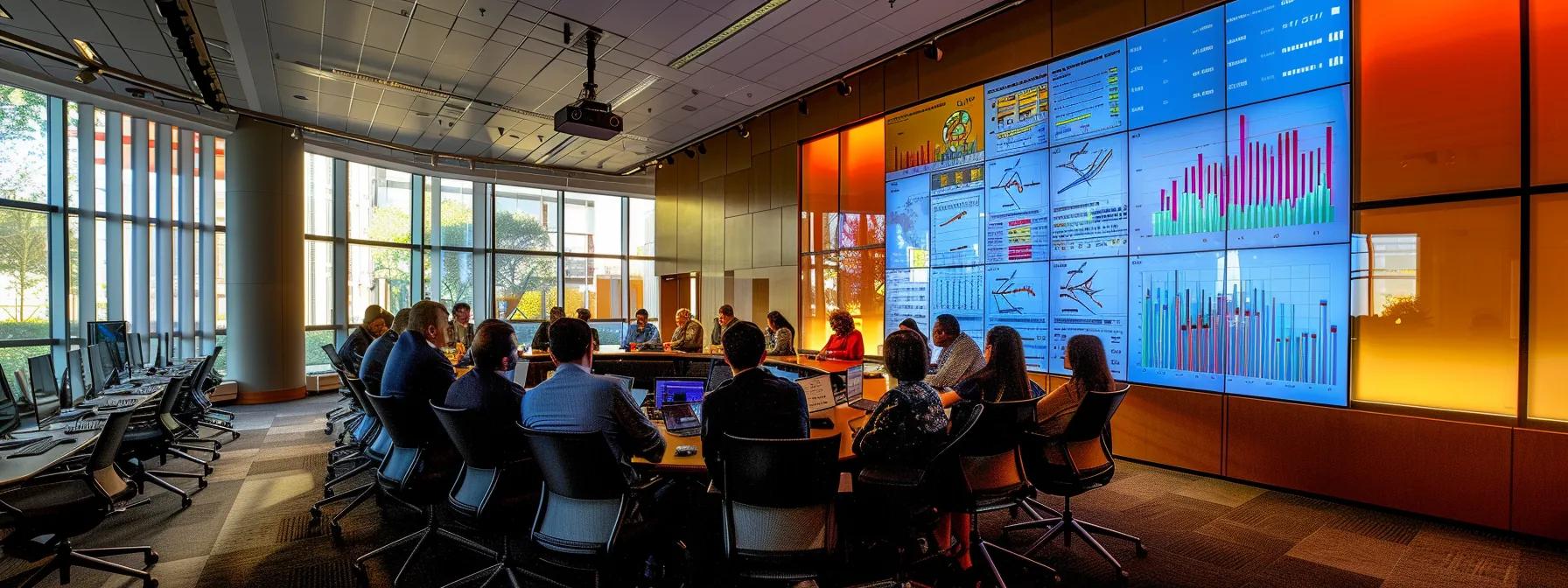
Aligning pricing models with marketing objectives is integral for organizations aiming to optimize their advertising efforts. This section includes case studies of successful ad campaigns across various platforms, showcasing how understanding the target audience and adapting strategies can impact expenses and enhance customer base engagement. Insights drawn from these examples will provide valuable lessons in effective pricing choices.
Aligning Pricing Models With Marketing Objectives
Aligning pricing models with marketing objectives is essential for organizations that utilize AI ad generators. The fee structures should reflect the infrastructure needs of the business while ensuring that marketers can achieve high-quality scores in their campaigns. For example, a company focusing on generating high engagement may benefit from a performance-based pricing model linked to their generative artificial intelligence capabilities, which adapts to the effectiveness of the ads produced.
Additionally, the choice of pricing model can significantly impact overall strategy and budget management. Businesses that prioritize robust machine learning techniques might find that a subscription model offers them consistent access to advanced features, allowing for sustained campaign improvements. By understanding their specific marketing goals and matching them with appropriate pricing strategies, organizations can optimize resource allocation and improve their advertising ROI.
Case Studies of Successful Ad Campaigns Across Various Platforms
Examining recent ad campaigns reveals how various platforms utilize AI ad generators to enhance their advertising strategies while aligning with business objectives. For instance, a company that provided incentives to early adopters saw a significant increase in customer engagement, ultimately translating to higher profit margins. By offering credits for referrals, they effectively encouraged users to experiment with their services, reinforcing the value of tailored campaigns within diversified pricing models.
Another example highlights a business focused on exceptional customer service that leveraged AI-generated ads to target specific demographics. By analyzing user behavior, they adjusted their campaigns to foster connections with potential clients, increasing click-through rates and conversions. This strategic use of AI in ad generation not only streamlined their advertising process but also fortified their market position, showcasing how understanding user intent can lead to successful financial outcomes.
User needs shape pricing decisions in an ever-changing landscape. As markets evolve, so do the pricing models for AI ad generators, signaling a shift worth examining.
Future Trends in AI Ad Generator Pricing Models
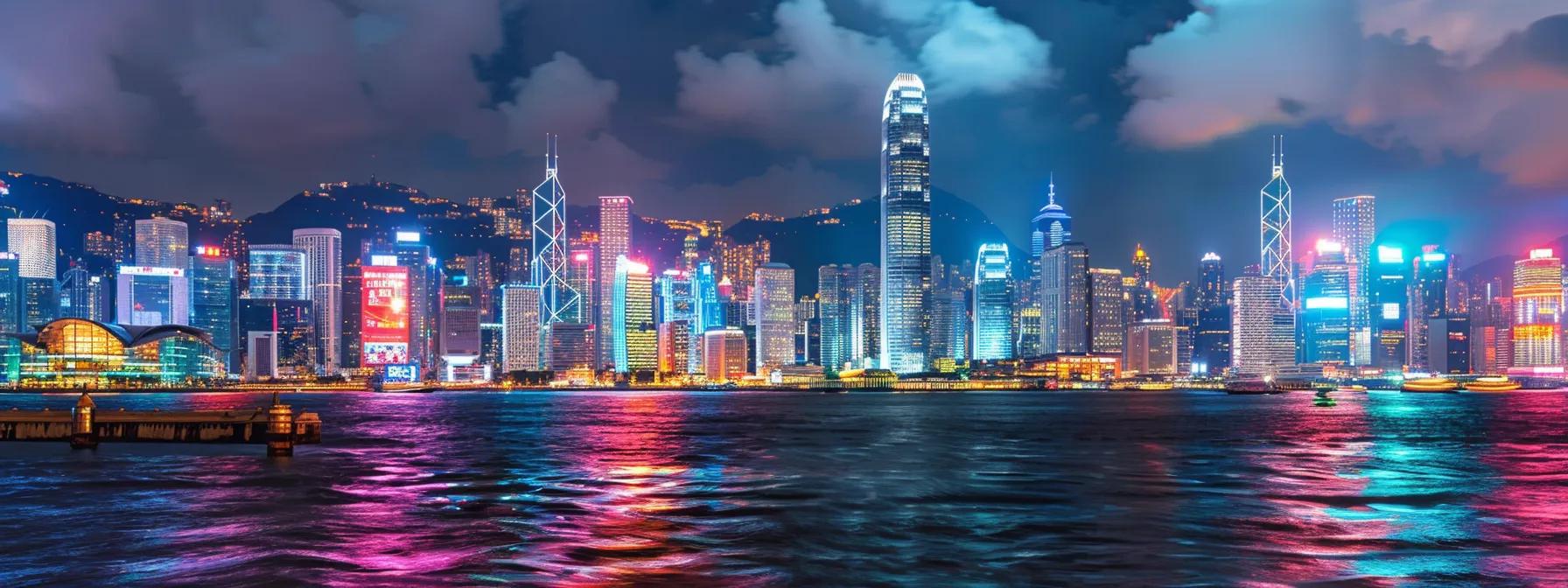
Predictions indicate that evolving pricing strategies for AI ad generators will increasingly incorporate advanced technologies, such as the Google Cloud Platform, enhancing the overall architecture of advertising solutions. As the ecosystem shifts, premium pricing models will likely become more prevalent, influencing how businesses adapt to these changes through tailored strategies that align with new parameters in the market.
The following sections will delve into the implications of technology advancements on pricing models and provide insights on effective strategies for businesses to navigate these pricing changes successfully.
Predictions for Evolving Pricing Strategies
As the digital marketing landscape evolves, AI ad generators are likely to adopt more dynamic pricing models that reflect usage patterns on social media platforms and search engine results pages. Industry players may implement hourly rates for services, allowing advertisers to pay based on real-time engagement metrics. This model not only aligns costs with specific outcomes but also enhances budget management, enabling businesses to allocate resources more effectively throughout their campaigns.
Moreover, integration with technologies like Google Cloud is expected to drive pricing innovations among major providers. As access to advanced data analytics becomes more prevalent, pricing structures may shift towards performance-based metrics, providing advertisers with insights into their advertising spend efficiency within the digital marketing domain. This strategic approach will empower marketers to make informed decisions while continuously optimizing their return on investment.
- Dynamic pricing models based on usage patterns.
- Integration of hourly rates for transparency and efficiency.
- Influence of Google Cloud on pricing innovations.
- Shift towards performance-based pricing metrics.
- Enhanced insights for informed decision-making in digital marketing.
The Impact of Technology Advancements on Pricing Models
Recent technological advancements are significantly reshaping pricing models in the AI advertising landscape. The integration of automation and DevOps practices allows companies to streamline their operations, resulting in more efficient pricing strategies that can adapt to market demands. Organizations that embrace this shift can effectively leverage auction-based pricing mechanisms, which dynamically adjust rates based on real-time supply and demand, offering a competitive edge in customer engagement.
The widespread adoption of advanced analytics tools enhances the ability to evaluate campaign performance, providing deeper insights into user behavior and preferences. By utilizing these insights, advertisers can refine their pricing models, transitioning from fixed rates to more flexible strategies that respond to market changes. This adjustment not only improves budget efficiency but also ensures that advertising efforts align more closely with consumer trends, leading to improved financial outcomes:
- Integration of automation and DevOps practices
- Dynamic adjustments through auction-based pricing
- Enhanced analytics for deeper insights
- Refining pricing models through market responsiveness
- Alignment of strategies with consumer trends
Strategies for Businesses to Adapt to Pricing Changes
Businesses can adapt to evolving pricing changes in AI ad generation by focusing on currency and measurement techniques to better understand their advertising spend. Implementing robust analytics tools allows organizations to track the volume of their ad placements and engagement levels, ensuring relevance in their marketing strategies. Adopting this analytical approach helps in identifying areas where budget adjustments may be necessary, ultimately minimizing churn rate and optimizing advertising efficiency.
Furthermore, establishing clear metrics to evaluate campaign performance can aid businesses in navigating fluctuating pricing structures. By concentrating on key performance indicators linked to specific advertising goals, companies can make strategic decisions aligned with their budget constraints. This targeted methodology not only enhances relevance in achieving advertising objectives but also provides a solid framework for managing costs effectively in a dynamic market environment:
- Understanding currency and measurement techniques.
- Utilizing analytics tools for tracking ad placements.
- Identifying opportunities to minimize churn rate.
- Establishing clear metrics for campaign performance.
- Aligning strategies with budget constraints.
Conclusion
Understanding how AI ad generator pricing models differ among major industry players is essential for advertisers seeking to optimize their marketing strategies. This knowledge allows businesses to identify the most effective pricing structures, whether subscription-based, pay-per-click, or performance-based, to align with their unique objectives. By analyzing these models, advertisers can make informed decisions that enhance their return on investment and ensure efficient budget management. Ultimately, grasping these differences empowers companies to navigate the competitive advertising landscape with confidence and effectiveness.