Navigating the costs of AI ad generators can be complex, especially when considering the varying pricing strategies across platforms. Many advertisers grapple with understanding the differences between token-based pricing and flat-rate models, which can significantly impact their budgets. This article will examine the fundamentals of AI ad generator pricing, analyze pricing structures of leading platforms, and explore the implications of ad spend on marketing strategies. By addressing these aspects, readers will gain insights into selecting a SaaS pricing strategy that can enhance their UGC ads and ultimately drive advertising revenue.
Key Takeaways
- Understanding pricing models helps advertisers optimize their budgets for AI ad generation
- Pay-per-click and subscription services offer distinct advantages for different advertising strategies
- Performance metrics are crucial in determining costs and enhancing the effectiveness of ad campaigns
- Features and add-ons can significantly influence overall expenses for AI ad generator platforms
- Dynamic pricing models are evolving to align advertising costs with real-time market demand
Understanding the Fundamentals of AI Ad Generator Pricing
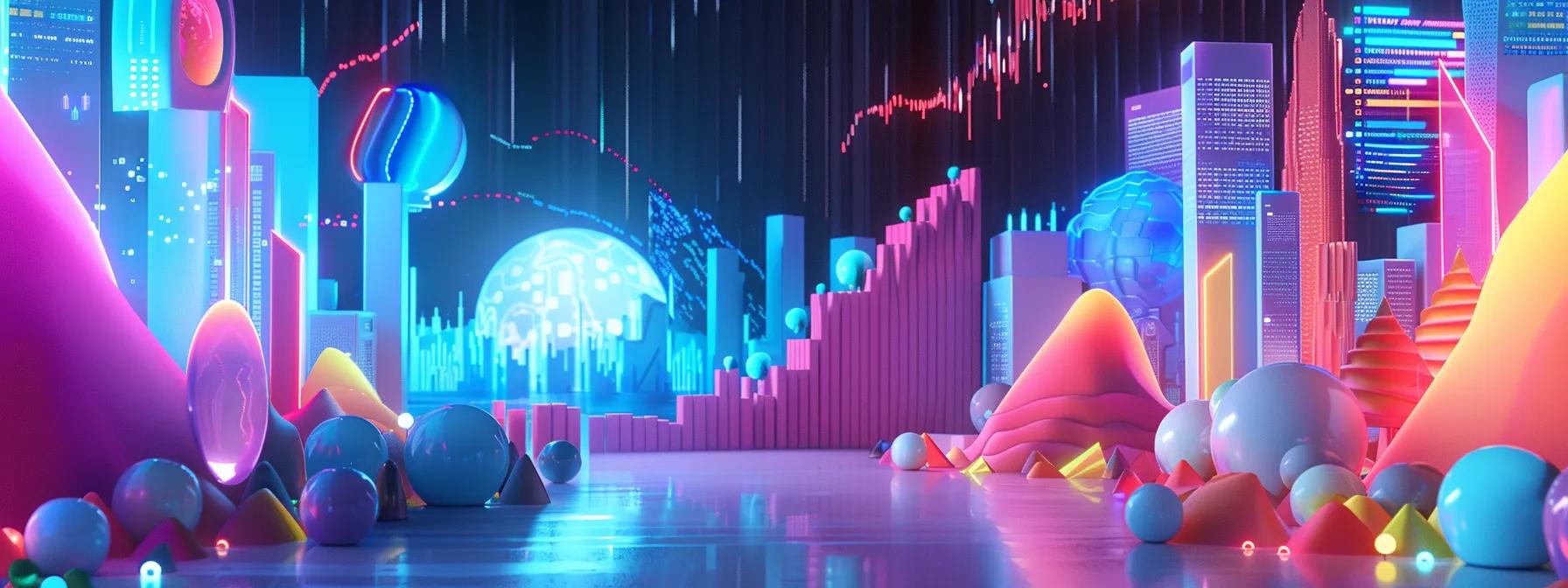
Understanding AI ad generator pricing involves several key components, including core pricing metrics crucial for launching an effective advertising campaign. Different models, such as pay-per-click, ugc ads, and subscription services, present unique advantages and drawbacks. Additionally, evaluating how performance affects pricing can inform decision-making. This section will provide insights into penetration pricing, flat rate options, and considerations around disk storage, guiding advertisers through the nuances of AI ad costs.
Defining Core Pricing Metrics in AI Ad Generation
In the realm of AI ad generation, understanding core pricing metrics is essential for advertisers aiming to optimize their budgets. One significant metric is the average cost per impression (CPI), which offers insights into how much is spent relative to the audience reach. Advertisers should also consider the value of machine learning algorithms in determining these costs, as effective targeting can lead to enhanced engagement and conversion rates, ultimately impacting overall performance.
Another crucial aspect involves examining different pricing strategies, such as pay-per-click (PPC) and subscription models, each presenting its own advantages. For instance, reputation plays a vital role in how these strategies are perceived; a reputable AI ad generator may command higher rates yet deliver superior results. Understanding these factors will equip advertisers to make informed decisions, ensuring that their investment in AI ad generation translates into meaningful returns.
Considering Usage Models: Pay-Per-Click vs. Subscription Services
When determining the best usage model for AI ad generation, advertisers in North America often weigh the options between pay-per-click (PPC) and subscription services. PPC allows brands to pay only when a user interacts with the ad, creating a direct correlation between cash spent and engaged users. This model can be particularly effective for businesses aiming to maximize their budget while gauging the effectiveness of various products without committing to large upfront costs.
On the other hand, subscription services, categorized under software as a service (SaaS), provide a fixed cost that covers a range of functions over a specified period. This option often appeals to companies that prefer predictable cash flow and access to a suite of tools without unexpected charges. By understanding the distinctions between these models, advertisers can select the option that aligns with their strategic objectives and financial capabilities, ultimately driving ROI through informed choices in AI ad generation.
Evaluating the Impact of Performance on Pricing
Performance metrics play a pivotal role in determining the pricing of AI ad generator services, particularly in the context of social media marketing. Advertisers must actively assess how engagement rates, conversion metrics, and audience insights impact overall costs. By leveraging observability tools, companies can refine their marketing strategy to ensure that the budget aligns with the effectiveness of their campaigns, ultimately enhancing returns on their investment.
Additionally, understanding how performance affects pricing allows marketers to explore opportunities for upselling or optimizing ad placements within their target market. When high-performing campaigns demonstrate significant audience engagement, advertisers can justify increased spend for those specific ads. Employing data-driven insights can help drive strategic adjustments that maximize the impact of each dollar spent, ensuring that pricing variations are effectively managed in pursuit of marketing goals.
Now that the basics of AI ad generator pricing are clear, it is time to look deeper. This next step reveals how various platforms approach their pricing structures and what it means for users.
Analyzing Pricing Structures Across Different AI Ad Generator Platforms
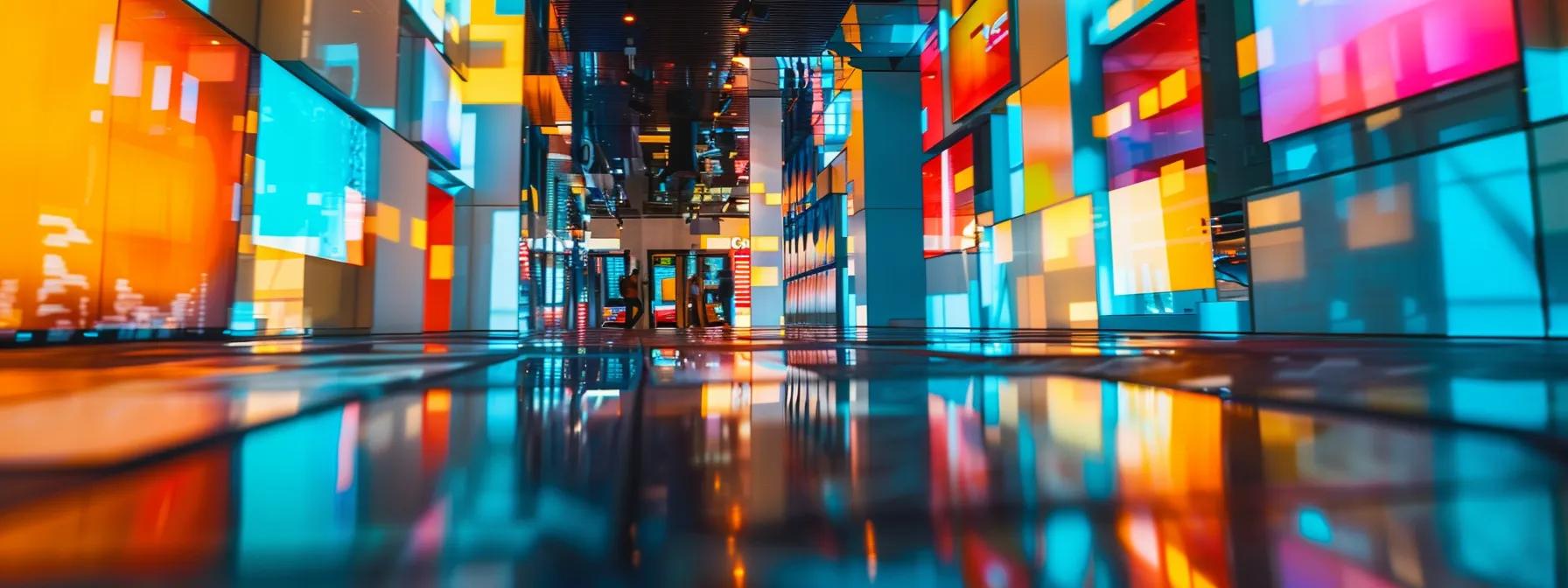
This section provides an overview of popular AI ad generator platforms and their diverse pricing models. It will examine factors that influence price differences among providers, signal trends driven by demand, and highlight how varying features and add-ons can affect overall costs. Understanding these elements is essential for advertisers looking to optimize brand awareness while navigating pricing variations.
Overview of Popular Platforms and Their Pricing Models
Various AI ad generator platforms offer distinct pricing models that cater to the diverse needs of advertisers. For instance, some platforms leverage a subscription-based approach, allowing users to access a range of tools powered by chatbots and virtual machines for a fixed monthly fee. This structure can simplify budgeting and facilitate streamlined workflows, as users know their costs upfront, which boosts planning efficiency.
On the other hand, certain platforms adopt a pay-per-click model that adjusts based on user engagement, a strategy influenced by the price elasticity of demand. In this case, as demand for effective ads grows, advertisers may find themselves spending more to maintain competitive visibility on search engines. By understanding these pricing variations, businesses can better align their advertising strategies with their financial goals, ensuring that their investments yield optimal returns.
Factors Influencing Price Differences Among Providers
Several factors contribute to the pricing differences among AI ad generator providers, especially in the domain of search advertising. One significant element is the pricing model adopted by each platform; some utilize dynamic pricing influenced by user engagement, while others may implement fixed pricing based on a specific value proposition. This variation requires advertisers to analyze their needs carefully to determine which system aligns best with their marketing goals, particularly in managing ad spending effectively.
The geographical location indicated by an IP address can also impact the cost structure of AI ad generator services. Providers may adjust their pricing based on localized competition and demand for specific advertising solutions. Consequently, understanding how regional trends and user behavior influence costs can enable advertisers to make strategic decisions about their campaigns, ensuring they receive the best value for their investments while maximizing performance across diverse markets.
Comparing Features and Add-Ons Affecting Costs
When evaluating AI ad generator platforms, assessing the features and add-ons can significantly impact overall costs. Key elements such as the number of servers utilized, the quality of the tools available for creating dynamic landing pages, and access to extensive resources can all drive pricing decisions. For example, platforms that offer premium features for A/B testing or advanced analytics may implement psychological pricing strategies, where the perceived value enhances the willingness to pay, ultimately affecting a marketer’s budget.
Moreover, understanding which features are essential versus those that are merely enhancements is crucial for creating an effective advertising strategy. Advertisers should prioritize tools that align with their specific objectives, as unnecessary add-ons can inflate costs without providing substantial benefits. For instance, if a platform includes integrated marketing automation tools that streamline retail campaign management, the additional expense may be justified by improved efficiency and increased revenue generation.
- Assess the importance of servers and their impact on pricing
- Understand the significance of landing page features
- Evaluate the value of available resources
- Consider psychological pricing in cost structures
- Identify essential features versus optional add-ons
As platforms vary in their pricing strategies, understanding what drives these costs becomes essential. Two main models emerge: token-based pricing and flat-rate pricing, each offering distinct advantages worth exploring.
Understanding Token-Based Pricing vs. Flat-Rate Pricing in AI Ad Generators
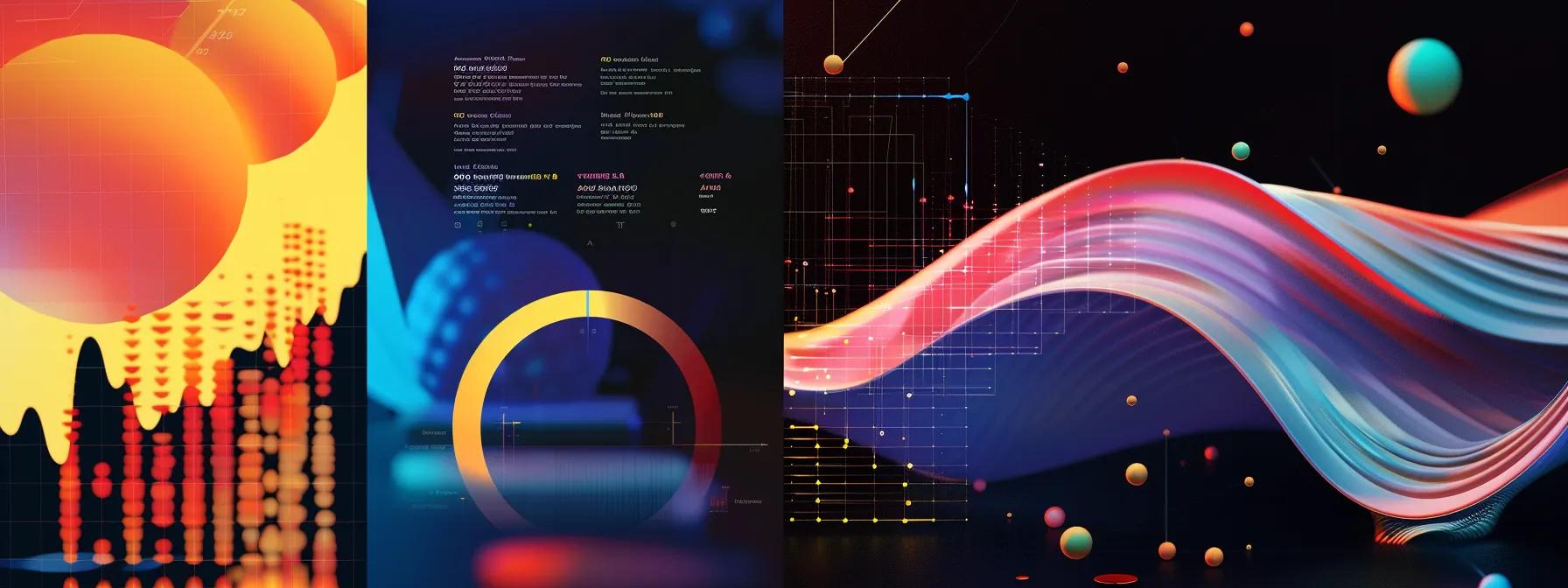
Token-based pricing in AI ad generation allows advertisers to pay based on the volume of usage, supporting scalability and promoting innovation through targeted campaigns. In contrast, flat-rate pricing offers predictable costs, simplifying budgeting but potentially limiting flexibility. This section will present the strengths and weaknesses of both approaches, providing insights into data analysis and profit margin considerations essential for informed decision-making.
How Token-Based Pricing Works in Ad Generation
Token-based pricing in AI ad generation allows advertisers to pay based on usage metrics, which can foster scalability in content marketing campaigns. By charging according to the volume of interactions, brands can closely monitor their expenditure against performance, aligning costs with the actual impact of their ads. This model also mitigates risk, as advertisers are only paying for services that yield tangible results, thus avoiding unnecessary overspend.
In addition, the integration of technologies such as natural language processing can enhance the effectiveness of token-based pricing. With lower latency in receiving feedback from campaigns, advertisers can quickly adjust their strategies based on real-time data, such as cost per mille (CPM) and engagement rates. This adaptive approach not only supports better decision-making but also ensures higher efficiency in reaching target audiences, maximizing the return on investment.
- Understanding the basis of token-based pricing.
- How this model aligns costs with performance.
- Mitigating risks associated with unpredictable spending.
- The role of natural language processing in enhancing campaign effectiveness.
- Adapting quickly to market changes with real-time data analysis.
The Implications of Flat-Rate Pricing on Budgeting
Flat-rate pricing in AI ad generators allows for fixed costs that simplify budgeting for advertisers. This pricing strategy enables brands to predict their expenses effectively while investing in tools designed to enhance visibility and improve user experience. For instance, companies can allocate a set budget over a period, ensuring that they can focus effectively on their marketing strategies without the constant worry of fluctuating costs based on customer behavior and engagement.
However, while flat-rate pricing provides budgeting stability, it may not align perfectly with specific usage patterns. Companies may find themselves paying for features they do not utilize fully, limiting their ability to adopt a more flexible, usage-based pricing model. By understanding the implications of flat-rate pricing, advertisers can make informed choices that balance cost predictability with the need for a strategy that adapts to changing customer behavior and market dynamics.
Advantages and Disadvantages of Each Pricing Approach
Token-based pricing offers significant advantages in affordability and adaptability for advertisers. This approach aligns costs directly with consumer engagement, allowing companies to tailor their advertising strategies based on real-time performance metrics. Success in sectors like manufacturing can be achieved by monitoring spending against output, enabling effective leadership in strategy formulation while fostering intelligence-driven decisions.
In contrast, flat-rate pricing provides stability in budgeting, which can appeal to businesses seeking predictability in their marketing expenses. However, this model may lead to inefficiencies, as companies might pay for features they do not utilize fully, potentially hindering their ability to optimize strategies. While the stability it offers can support a clear path forward, advertisers must remain vigilant about aligning their investment with their evolving needs in a competitive landscape.
As advertisers navigate the pricing models of AI ad generators, it becomes clear that understanding ad spend is equally crucial. The decisions made about budgets can shape not just costs, but the very success of campaigns as well.
The Role of Ad Spend and Budgeting in AI Ad Generator Pricing
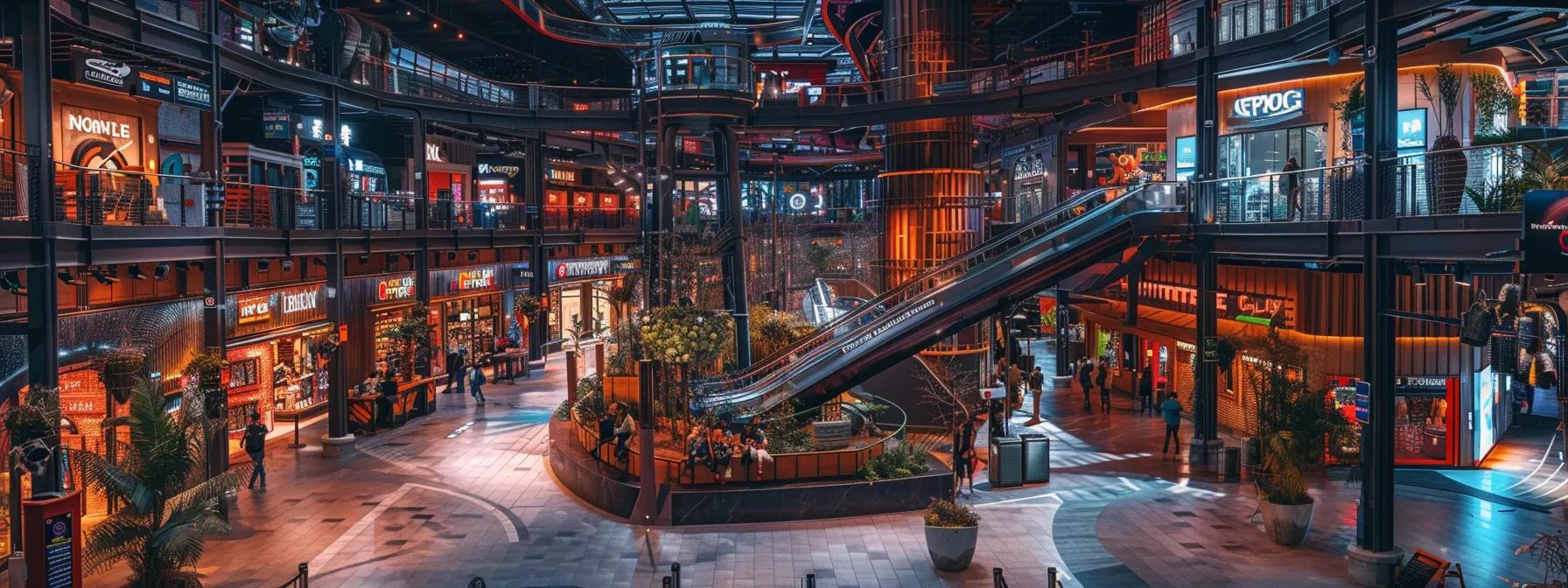
Ad spend significantly influences AI generator costs, directly impacting the total cost of ownership and overall revenue. Setting budget limits is essential for advertisers to align their financial expectations with return on investment (ROI) goals. Additionally, employing strategies to maximize the value from ad spend can enhance market share, especially when using platforms like Microsoft Azure with efficient cloud storage solutions. The following sections will delve into each topic, providing actionable insights for effective budgeting and spending in AI ad generation.
How Ad Spend Influences AI Generator Costs
The relationship between ad spend and AI generator costs is integral for advertisers seeking to maximize their investments. High ad expenditure often correlates with enhanced visibility and reach, directly influencing the effectiveness of campaigns. SaaS pricing models reflect this dynamic, where advertisers can track their spending against performance analytics to ensure they align their budgets with their strategic goals, allowing for better management of inventory and more accurate projections of overall price commitments.
Moreover, understanding how preference among different pricing strategies affects costs can empower advertisers to make informed decisions. For instance, a focus on high-impact ads may necessitate a higher budget, yet can result in superior engagement and return on investment. By leveraging data analytics, marketers can refine their ad strategies, optimize inventory usage, and better navigate the complexities of AI ad generator pricing, ultimately improving their overall campaign effectiveness.
Setting Budget Limits and ROI Expectations
Setting budget limits is a critical aspect of managing costs associated with AI ad generators, particularly in online advertising. Advertisers must consider algorithms that enhance customer engagement when determining how much to allocate. By establishing clear limits based on their specific marketing objectives, brands can effectively control spending while maximizing the return on investment (ROI) for their campaigns.
Moreover, aligning budget limits with a relevant unit of measurement, such as cost per acquisition or customer lifetime value, empowers advertisers to gauge their financial performance accurately. When the budget reflects these metrics, brands can make informed decisions about adjusting their advertising strategies, ensuring they invest resources where they can yield the best outcomes. This proactive approach not only helps in optimizing performance but also guides future spending decisions in the realm of AI ad generation.
Strategies for Maximizing Value From Ad Spend
To maximize value from ad spend, advertisers should begin with a comprehensive understanding of their licensing agreements with AI ad generation platforms. By reviewing these agreements, brands can identify features that optimize performance and enhance campaign effectiveness. For instance, employing sentiment analysis tools can significantly enhance targeting precision, ensuring that marketing messages resonate with the intended audience.
Moreover, implementing predictive analytics in budget allocation can lead to more informed spending decisions. By analyzing past campaign data and trends, advertisers can adjust their financial commitments based on anticipated outcomes. This data-driven approach not only facilitates better resource management but also ensures that each dollar spent contributes to achieving their defined ROI goals, making their budgeting process more effective and efficient.
Understanding pricing is only part of the journey. Next, real-world examples will reveal how top AI ad generators navigate their strategies.
Case Studies: Pricing Strategies of Leading AI Ad Generators
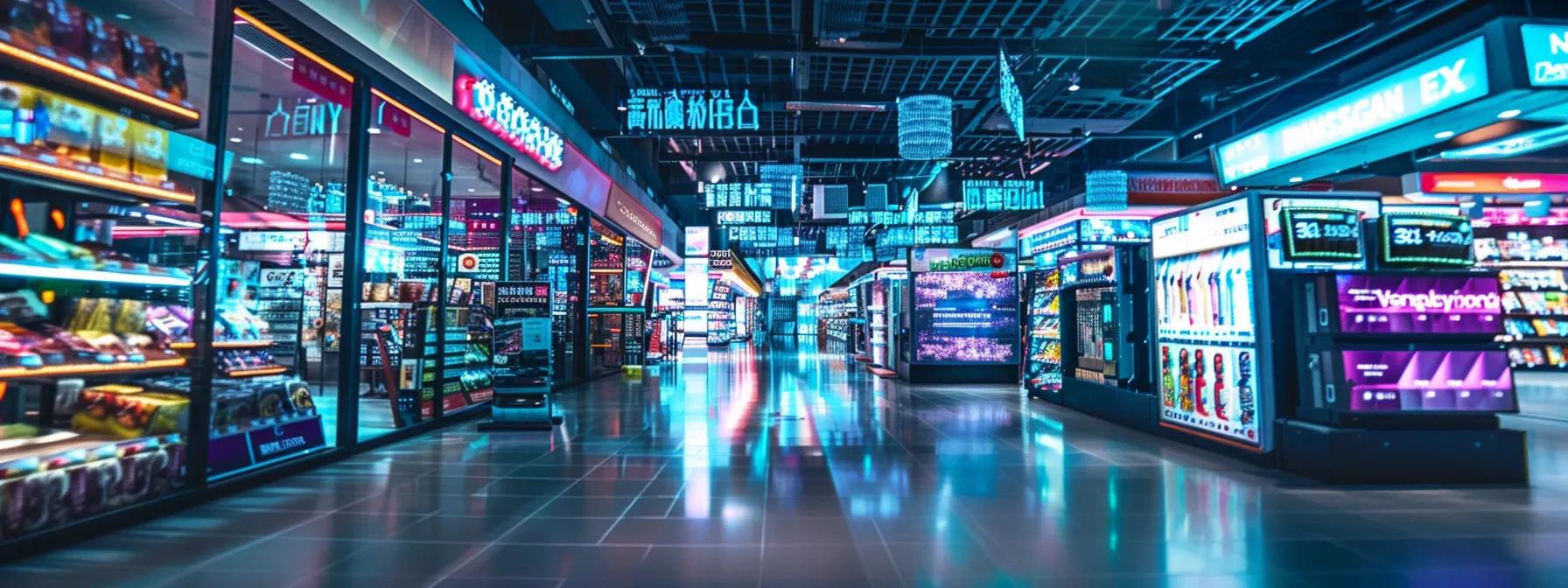
This section provides an analysis of the pricing strategies employed by leading AI ad generators. It begins with an examination of Google’s Ad AI pricing model, exploring how it aligns expenses with targeted audience engagement. Next, it examines OpenAI’s approach to ad costs, shedding light on its methodology for reaching diverse customer bases. Lastly, lessons from other emerging AI ad platforms will be discussed, offering practical insights to organizations aiming to optimize their advertising expenditures.
Analyzing Google's Ad AI Pricing Model
Google’s Ad AI pricing model integrates machine learning to optimize advertising costs based on various factors, including campaign performance and quality score. Advertisers are charged a fee that fluctuates depending on the effectiveness of their ads, allowing them to maximize their investment while leveraging generative artificial intelligence capabilities to enhance ad creativity and engagement. This dynamic pricing structure aligns well with current market demands and encourages advertisers to focus on ad quality, ultimately influencing their overall return on investment.
The underlying infrastructure of Google’s Ad AI supports sophisticated analytics, allowing advertisers to track their expenses relative to each campaign’s success. By emphasizing the importance of quality score, Google motivates advertisers to create high-performing ads that resonate with target audiences, thereby improving overall campaign effectiveness. This approach not only helps in managing costs effectively but also ensures that advertisers can refine their strategies based on data-driven insights, leading to more impactful advertising efforts.
Understanding OpenAI's Approach to Ad Costs
OpenAI adopts a credit-based pricing model for its ad services, enabling advertisers to purchase credits that can be used for various advertising experiments. This approach creates an incentive for brands to engage actively with the platform, adjusting their strategies based on the feedback received from each campaign. By allowing users to experiment with different ad formats and targeting options, OpenAI facilitates a more tailored advertising experience that can lead to greater profit.
Additionally, OpenAI emphasizes customer service as a core component of its pricing strategy, ensuring users have the support necessary to maximize their investments. Clients benefit from practical insights and guidance on effectively utilizing their credits, helping them refine their advertising efforts based on performance data. This combination of an incentive-driven model and proactive customer service positions OpenAI as a valuable partner for brands navigating the complexities of AI ad generator costs:
- Credit-based purchases enable flexible ad campaigns.
- Advertisers are encouraged to experiment with strategies.
- Customer service enhances user experience and guides investments.
- Data-driven insights support refining ad strategies.
- Enhanced profitability through tailored advertising efforts.
Lessons From Other Emerging AI Ad Platforms
Emerging AI ad platforms are increasingly leveraging the Google Cloud Platform to enhance their architecture and improve service delivery. These platforms typically implement premium pricing structures, allowing them to offer unique features and capabilities that can significantly benefit advertisers. By utilizing parameters like user engagement analytics and automated optimization tools, they create a robust ecosystem that supports effective advertising strategies.
Additionally, the price variations among these platforms often depend on the specific features they provide. For instance, some platforms focus on advanced targeting capabilities that can yield higher returns on investment, warranting their premium pricing. Advertisers should explore these offerings carefully to align their objectives with the pricing model, ensuring they choose a solution that meets their needs effectively:
- Invest in platforms using advanced architecture for better service.
- Evaluate features to justify premium pricing.
- Focus on user engagement analytics for optimal advertising strategies.
As businesses adapt, the landscape of pricing models shifts. The future holds new strategies that could redefine how AI ad generators charge for their services.
Future Trends in AI Ad Generator Pricing Models
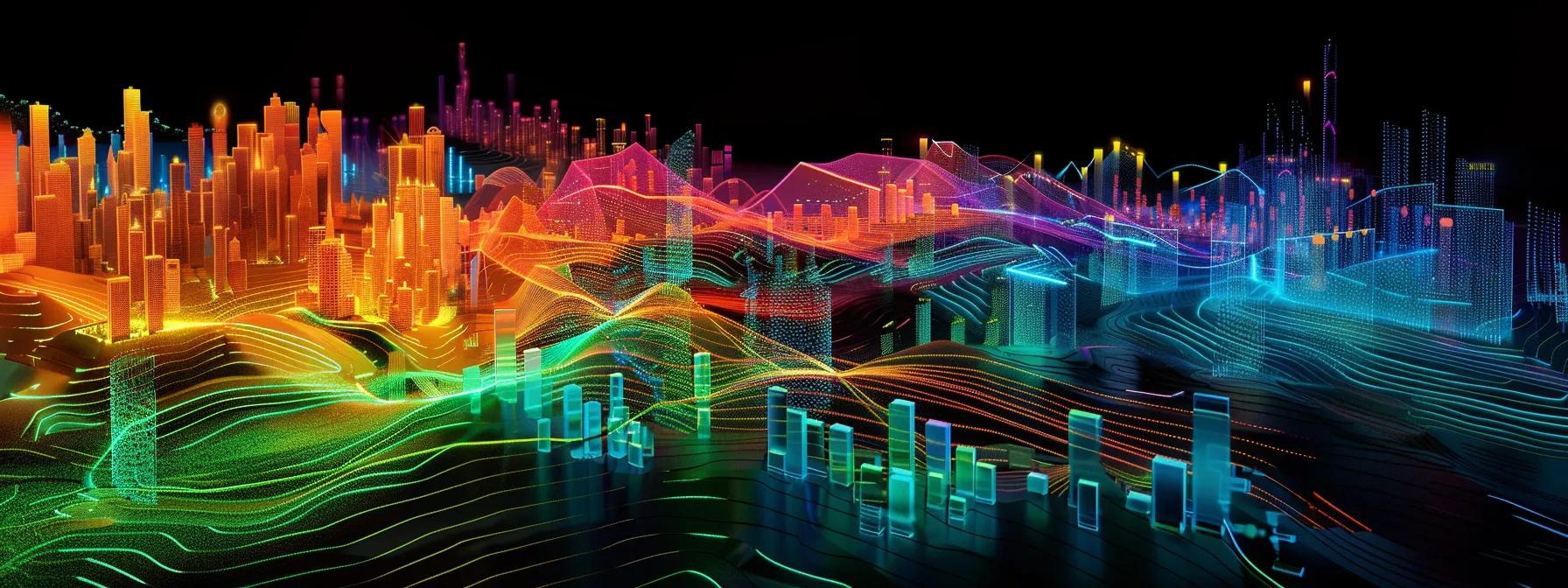
The future of AI ad generator pricing models is evolving, with a notable shift towards dynamic pricing in AI solutions. As consumer demand for personalized digital marketing experiences rises, pricing structures are expected to adapt accordingly. Innovations using platforms like Google Cloud may further impact pricing, creating opportunities for optimizing engagement across social media and search engine results pages.
This section will explore anticipated changes in consumer preferences, the shift to more flexible pricing models, and how these innovations may reshape the landscape of AI ad generation, providing advertisers with practical insights and strategies.
The Movement Towards Dynamic Pricing in AI Solutions
The shift towards dynamic pricing in AI solutions is reshaping the advertising landscape by fostering a more responsive and competitive environment. This model utilizes auction-based mechanisms, enabling advertisers to bid on advertising space based on real-time demand and performance metrics. As more companies adopt dynamic pricing, the focus on automation and data analysis becomes crucial to optimize ad spend and improve campaign effectiveness.
Anticipated Changes in Consumer Demand and Pricing Adaptation
As consumer preferences evolve, advertisers will increasingly demand more relevant and tailored advertising experiences. This shift is likely to influence pricing models, as the volume of data available for measurement grows. By utilizing advanced analytics, companies can adapt to changing consumer behaviors, reducing churn rates while maximizing ROI through targeted and efficient ad placements.
Future pricing strategies may include flexible currencies that respond dynamically to market trends. This approach enables advertisers to allocate resources based on real-time performance metrics, ensuring that expenditures align with actual engagement. By embracing these changes, businesses can foster a more adaptive advertising framework that meets the needs of diverse audiences while optimizing their advertising budgets.
Innovations in Pricing Structures and Their Potential Impact
Innovations in pricing structures for AI ad generators are increasingly centered around technologies like Kubernetes, which facilitate better resource management and scalability. By adopting container orchestration, companies can dynamically adjust their content creation costs based on real-time demand and user engagement. This ensures that brands only pay for the resources they consume, enhancing budget efficiency and enabling more responsive advertising strategies that align closely with business objectives.
Moreover, the emergence of flexible pricing models allows brands to select features that specifically meet their content creation needs, fostering a more tailored advertising experience. As these models evolve, advertisers can expect to see integration of advanced analytics that help forecast engagement levels, providing insights that empower brands to make informed decisions about ad spend. This adaptability not only enhances profitability but also positions businesses to stay competitive in a rapidly changing digital landscape.
Conclusion
Understanding the complexities of AI ad generator costs is essential for advertisers who aim to maximize their return on investment. By evaluating different pricing modelsāsuch as pay-per-click and subscription servicesāadvertisers can align their strategies with their financial goals and market dynamics. Additionally, analyzing performance metrics and feature offerings allows for informed decision-making regarding expenditures. Ultimately, navigating these costs effectively empowers brands to enhance their advertising impact and achieve sustained growth in a competitive landscape.