Measuring success in AI ad campaigns is essential for maximizing the effectiveness of UGC ads. Many advertisers struggle with understanding which metrics truly reflect their campaign’s performance. This article will outline key performance indicators (KPIs) to track, the role of data quality in analyzing results, and how budget impacts campaigns. By engaging with this content, readers will gain practical insights to improve their marketing data analysis, ensuring they can make informed decisions that enhance their advertising efforts and address common challenges.
Key Takeaways
- Understanding metrics is essential for optimizing AI ad campaign effectiveness
- Analyzing customer lifetime value helps tailor strategies for increased retention and profitability
- Implementing A/B testing allows for data-driven refinements in advertising approaches
- Utilizing predictive analytics enhances foresight into campaign performance and outcomes
- Incorporating customer feedback is vital for improving engagement and overall ad effectiveness
Understanding the Importance of AI Ad Campaign Metrics
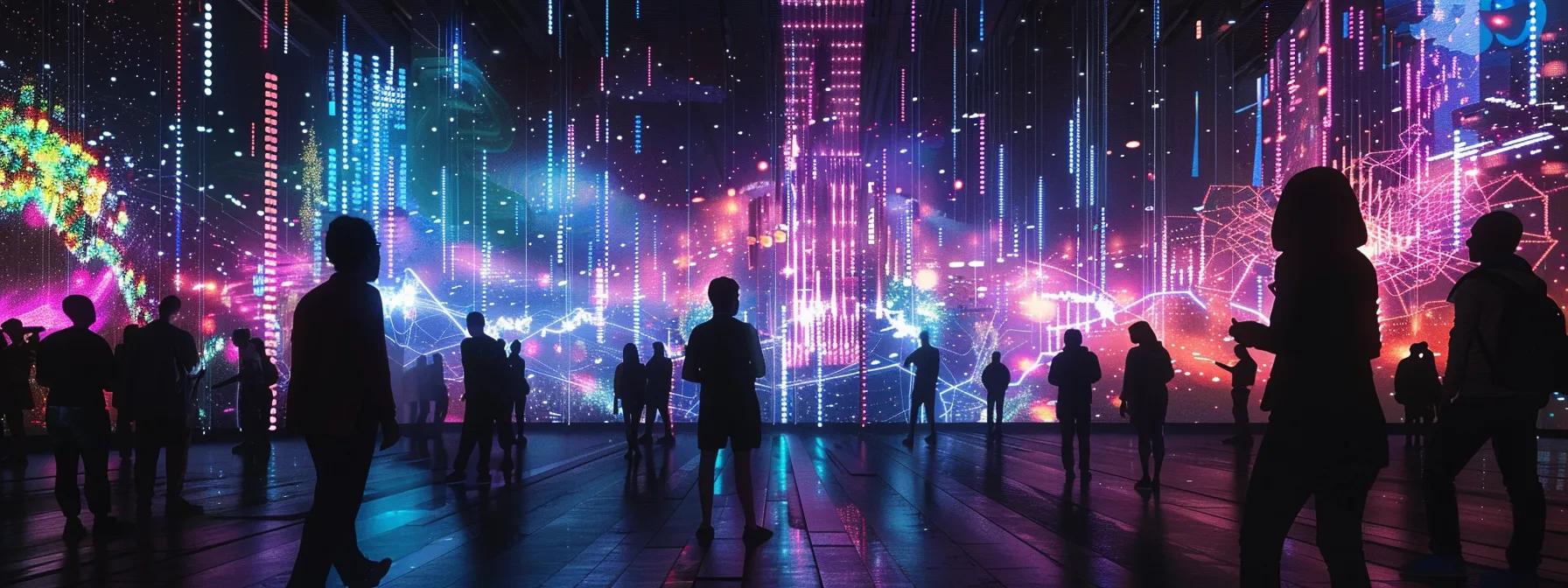
In the realm of AI ad campaigns, such as ugc ads, understanding metrics is fundamental for effective campaign management. Metrics offer insights into how well each ad performs, allowing marketers to optimize their strategies for better outcomes.
Attribution plays a critical role in determining which components of an ad campaign contribute to overall success. By analyzing attribution, advertisers can identify the touchpoints that most significantly enhance brand awareness, leading to more informed decision-making.
Cost per mille (CPM) is a key metric for evaluating the efficiency of ad spend in campaigns like Facebook ads. A lower CPM indicates a more cost-effective approach, ensuring that brand messages reach a larger audience without excessive expenditure.
Focusing on these metrics allows advertisers to refine their campaigns continuously. By utilizing data-driven insights, they can enhance their advertising efforts and achieve better results in terms of reach and engagement:
- Evaluate the effectiveness of different channels.
- Understand which ads drive engagement.
- Optimize spending for maximum impact.
- Enhance brand awareness through targeted campaigns.
Understanding AI ad metrics is the first step. Now, the real challenge lies in pinpointing the key performance indicators that will guide your campaign to success.
Identifying Key Performance Indicators for AI Ad Campaigns
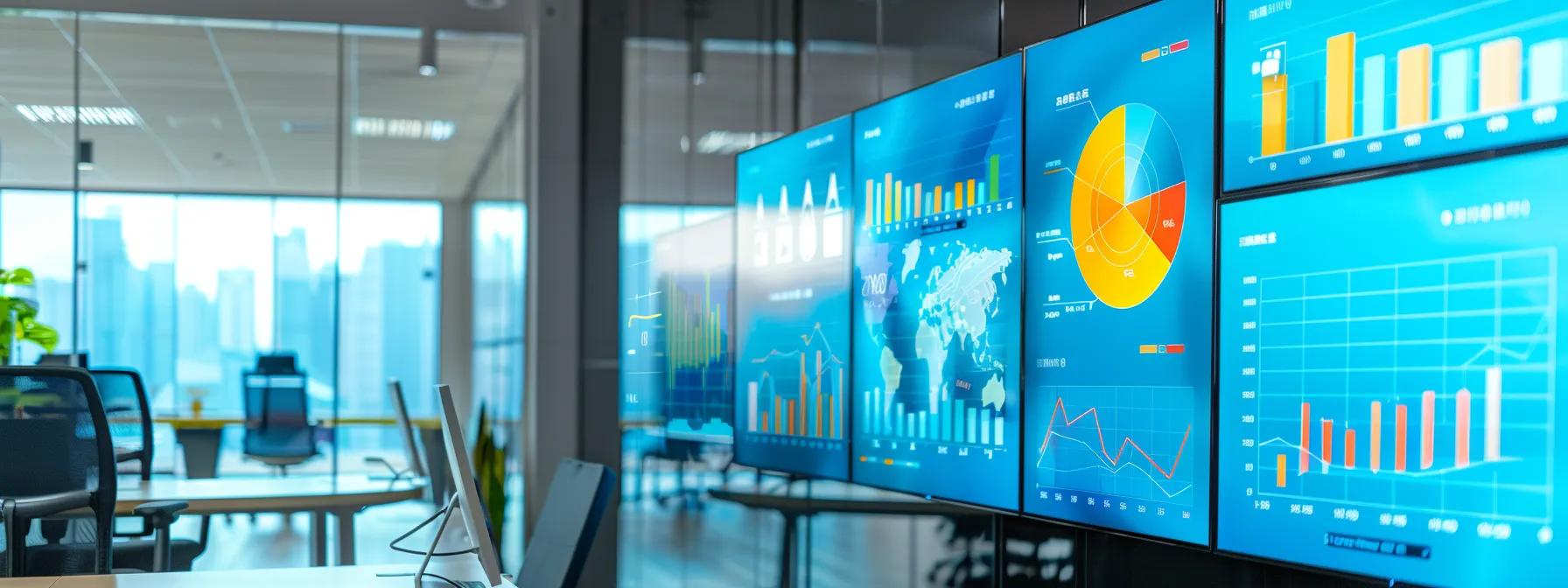
Successful AI ad campaigns rely on clearly defined key performance indicators (KPIs) to measure effectiveness. Metrics such as tracking impressions and reach provide insight into brand visibility, while monitoring click-through rates reveals engagement levels. Analyzing conversion rates and return on investment (ROI) showcases the direct benefits of campaigns. Evaluating customer lifetime value enhances understanding of long-term effects on customer satisfaction. Finally, using attribution models allows for more precise measurement across the marketing mix, optimizing strategies for better outcomes.
Tracking Impressions and Reach in Advertising
Tracking impressions and reach is vital for measuring the visibility of AI ad campaigns. By monitoring these metrics, advertisers gain insights into how many potential customers have encountered their content. This data is essential for understanding the effectiveness of the advertising infrastructure and helps in making informed decisions regarding pricing strategies and overall campaign adjustments.
Moreover, reach is not just about numbers; it also relates to enhancing the customer experience through tailored messaging. Insights from tracking these metrics can inform how well influencer marketing initiatives resonate with target audiences. As organizations refine their strategies based on these findings, they can improve engagement, ensuring that their advertising efforts effectively convert impressions into lasting customer relationships.
Monitoring Click-Through Rates for Engagement
Monitoring click-through rates (CTR) is crucial for assessing engagement in AI ad campaigns. By employing a straightforward formula to calculate CTR, marketers gain valuable insights into how effectively their social media content captures audience interest. Analyzing CTR data through effective data analysis transforms this metric into a strategy for determining the appeal of specific ads and the overall effectiveness of various marketing channels.
Understanding the correlation between CTR and profit margin can significantly influence advertising decisions. Higher click-through rates often translate to increased traffic and potential conversions, allowing advertisers to optimize their campaigns further. By focusing on boosting this key performance indicator, organizations can refine their content and strategies, ensuring they resonate with their target audience and drive desired business outcomes.
Analyzing Conversion Rates and ROI
Analyzing conversion rates is essential for evaluating the success of a marketing campaign, particularly in the context of display ads and account-based marketing (ABM). A higher conversion rate indicates that the ads resonate well with the target audience, transforming interest into action. By tracking these rates alongside the associated expenses of the campaign, marketers can determine which strategies yield the best return on investment and shift resources accordingly.
Return on investment (ROI) analysis goes hand-in-hand with conversion rate evaluation, providing a comprehensive view of a campaign‘s financial effectiveness. Detecting patterns in how different types of machine learning-driven ads perform can reveal insights into cost-efficiency and customer behavior. Understanding the impact of these factors equips advertisers with the knowledge needed to refine their advertising strategies, ensuring that future campaigns maximize returns while minimizing unnecessary costs.
Evaluating Customer Lifetime Value in Ad Campaigns
Evaluating customer lifetime value (CLV) is essential for understanding the long-term effectiveness of ad campaigns in performance marketing. CLV quantifies the total revenue expected from a customer throughout their relationship with a brand, making it a critical metric for assessing the return on investment (ROI) of media buying strategies. By integrating this metric, marketers can identify the most profitable customer segments, helping to tailor their tactics, including targeted email marketing campaigns, to maximize retention and increase overall value.
Utilizing treatment and control groups can further enhance the analysis of CLV in ad campaigns. By comparing the performance differences between groups exposed to specific ads and those that are not, organizations can gain insights into the effectiveness of their messaging and engagement strategies. This approach allows advertisers to refine their campaigns and develop more effective media buying techniques, ultimately improving customer satisfaction and boosting CLV across the board.
Using Attribution Models for Accurate Measurement
Attribution models serve as essential tools for marketers, enabling accurate measurement of customer interactions throughout both online and offline channels. These models clarify how various touchpoints contribute to a customer‘s journey, allowing advertisers to refine their audience segmentation strategies. For example, using a multi-touch attribution model can highlight the combined effect of digital ads, social media interactions, and offline promotions on driving conversions, reducing the complexity of understanding customer behavior.
Implementing these models can significantly enhance the efficacy of an advertising campaign. Marketers gain the ability to analyze which customer touchpoints are most effective, fostering informed decisions that align with their business objectives. By utilizing insights derived from attribution models, organizations can allocate resources more strategically, ensuring that marketing efforts resonate with their target audience and yield optimal results across all advertising efforts.
With the right indicators in hand, the next step unfolds clearly. Data will reveal the truths behind success, shedding light on unseen patterns and paths forward.
Analyzing the Role of Data in Measuring Success
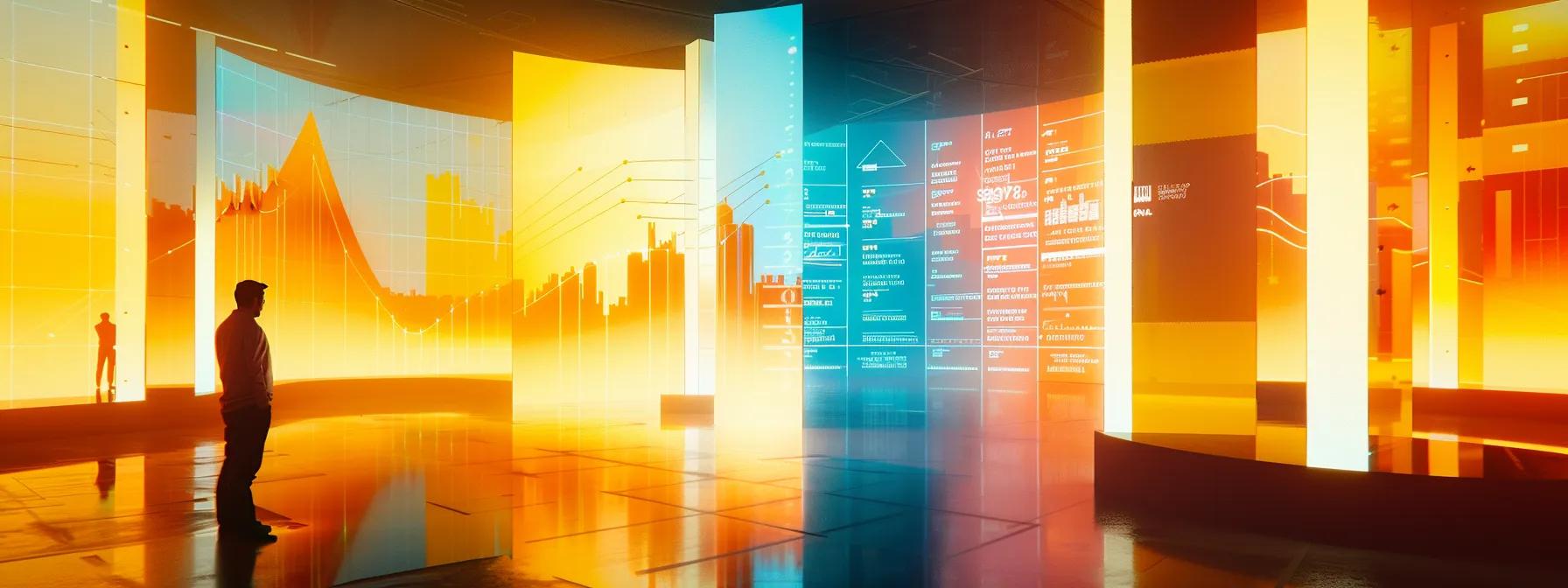
Utilizing predictive analytics allows advertisers to forecast campaign performance, enhancing visibility into future outcomes. Implementing A/B testing provides a systematic approach to continuous improvement, enabling brands to refine their landing pages for optimal engagement with the target audience. Leveraging machine learning offers real-time insights that adjust strategies dynamically, ensuring that campaigns effectively maximize their assets and comply with relevant regulations.
Utilizing Predictive Analytics for Performance Forecasting
Utilizing predictive analytics significantly enhances the measurement of success in AI ad campaigns by enabling marketers to anticipate campaign performance. By analyzing historical data, marketers can identify trends and patterns that inform their online advertising strategies, leading to more targeted and effective campaigns. This proactive approach helps organizations stay ahead in a competitive landscape, ensuring that they maximize their advertising budgets while meeting regulatory compliance requirements.
Additionally, predictive analytics allows advertisers to test various elements within their campaigns before full-scale implementation. By employing A/B testing to evaluate different ad creatives or audience segments, they gain actionable insights that can optimize their measurement processes. This systematic evaluation aids in fine-tuning campaigns, ultimately driving better engagement and conversion rates, which are essential for assessing success in the ever-evolving online advertising environment:
- Identify key patterns and trends from historical data.
- Anticipate future campaign performance.
- Conduct A/B testing for effective measurements.
- Ensure regulatory compliance while optimizing strategies.
Implementing a/B Testing for Continuous Improvement
Implementing A/B testing is a crucial strategy for advertisers aiming to enhance display advertising campaigns. This methodology allows marketers to compare two versions of an ad to determine which one yields better results based on specified performance indicators. For example, by adjusting a single element, such as the call-to-action, advertisers can gather data on how much this change impacts conversion rates, providing a percentage that reflects success and guiding future campaigns.
Understanding the outcomes of A/B testing is essential for refining marketing analytics and improving overall campaign effectiveness. By systematically analyzing the performance of different ad variations, marketers gain actionable insights that inform adjustments and optimizations. This iterative process not only enhances current strategies but also builds a foundation for long-term success in measuring performance across all advertising efforts.
Leveraging Machine Learning for Real-Time Insights
Leveraging machine learning significantly enhances campaign measurement in digital marketing by providing real-time insights that inform strategic decisions. By analyzing patterns in customer behavior, marketers can increase open rates and engagement levels through targeted messaging tailored to specific audience preferences. This approach facilitates the optimization of return on marketing investment, ensuring that resources are allocated effectively to maximize effectiveness.
Moreover, machine learning algorithms can adapt to changing market conditions, allowing advertisers to refine their campaigns on the fly. For example, as data accumulates, insights gained can reveal trends that affect customer engagement, enabling immediate adjustments to ad placements or creative content. This responsiveness not only improves campaign performance but also contributes to a more agile marketing strategy, essential for success in the fast-paced landscape of digital advertising.
Data tells a story, but the audience shapes its meaning. Next, it’s time to see how people connect with AI ad campaigns and what that means for success.
Assessing Audience Engagement in AI Ad Campaigns
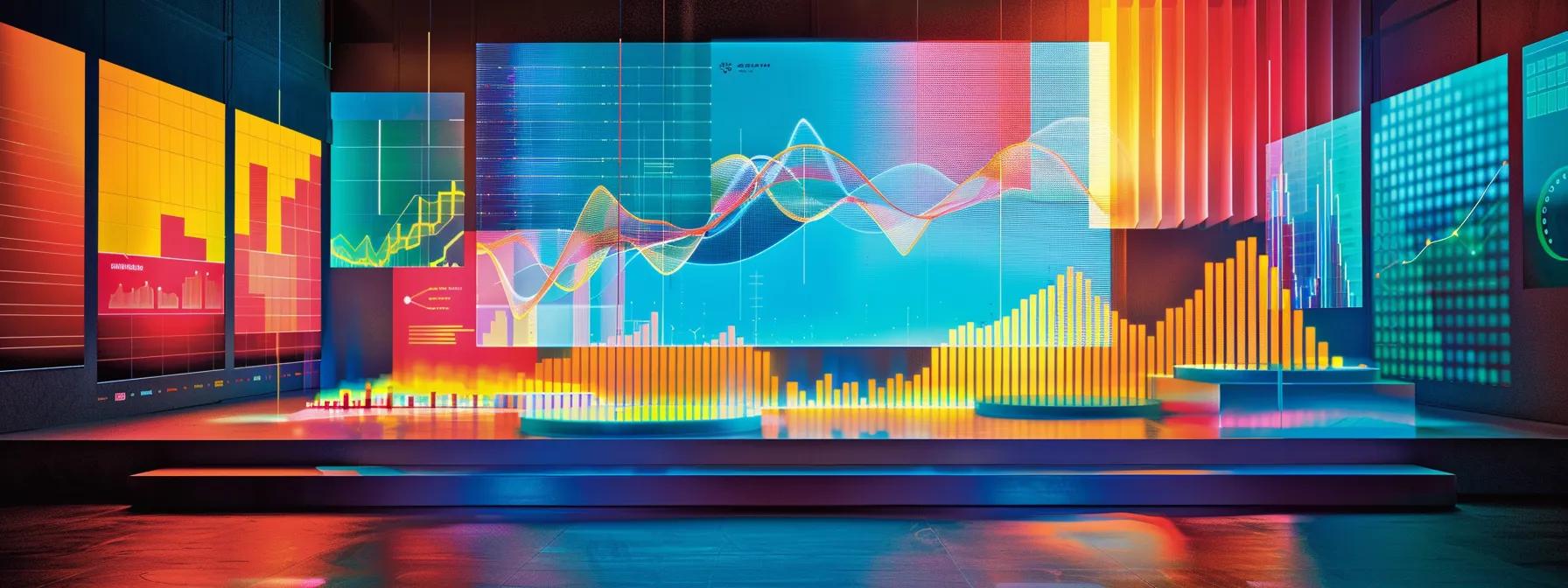
Measuring audience engagement in AI ad campaigns extends beyond tracking clicks. It involves analyzing engagement metrics, understanding user behavior through heatmaps, and incorporating social media signals into overall metrics. This comprehensive approach enables brands to work smarter by optimizing their copywriting and enhancing profit margins, ultimately leading to more effective Google Ads campaigns.
Measuring Engagement Metrics Beyond Clicks
Measuring engagement metrics beyond clicks is essential for evaluating the overall efficiency of performance marketing campaigns. Advertisers should consider metrics such as time spent on page, scroll depth, and interaction rates with content. These indicators help gauge whether ads are capturing audience attention and driving meaningful interactions, which are crucial for assessing campaign success and supporting lead generation efforts.
Understanding audience engagement goes beyond mere click rates, facilitating a deeper evaluation of the effectiveness of advertising strategies. For instance, tracking social media shares and comments can provide insights into how well content resonates with the audience. This comprehensive look at engagement metrics enables brands to refine their messaging and tactics, enhancing the potential for achieving better outcomes across their marketing campaigns.
Understanding User Behavior Through Heatmaps
Understanding user behavior through heatmaps provides invaluable insights for enhancing AI ad campaigns. Heatmaps visually represent user interactions on web pages, allowing marketers to identify where users click, scroll, and spend their time. This information is essential for fine-tuning placement strategies, optimizing call-to-action buttons, and reducing bounce rates, ultimately leading to increased revenue and improved cost per action for advertisers.
By analyzing heatmap data, marketers can also understand engagement patterns specific to mobile app advertising. For instance, observing how users interact with ads on mobile devices versus desktops can inform the development of targeted content strategies. Such insights ensure that advertising efforts align with user preferences, fostering higher engagement levels and more effective search engine optimization practices.
Incorporating Social Media Signals Into Metrics
Incorporating social media signals into metrics enhances the assessment of audience engagement in AI ad campaigns. By analyzing consumer interactions, such as shares, likes, and comments, advertisers gain insight into the effectiveness of their display advertising efforts. This data assists marketers in understanding how social engagement correlates with advertising ROI, allowing them to refine strategies and improve campaign performance significantly.
Furthermore, leveraging natural language generation tools can streamline the analysis of social media data, making it easier to detect patterns and trends. For instance, by monitoring sentiment analysis from social media, advertisers can determine how their messaging resonates with consumers and identify any potential issues related to advertising fraud. This approach not only fosters more effective engagement but also equips marketers with actionable insights that support sustained success in their campaigns.
Audience engagement reveals much about the success of AI ad campaigns. Next, the budget plays a crucial role, shaping outcomes and strategies that cannot be ignored.
Understanding the Impact of Budget on AI Ad Campaigns
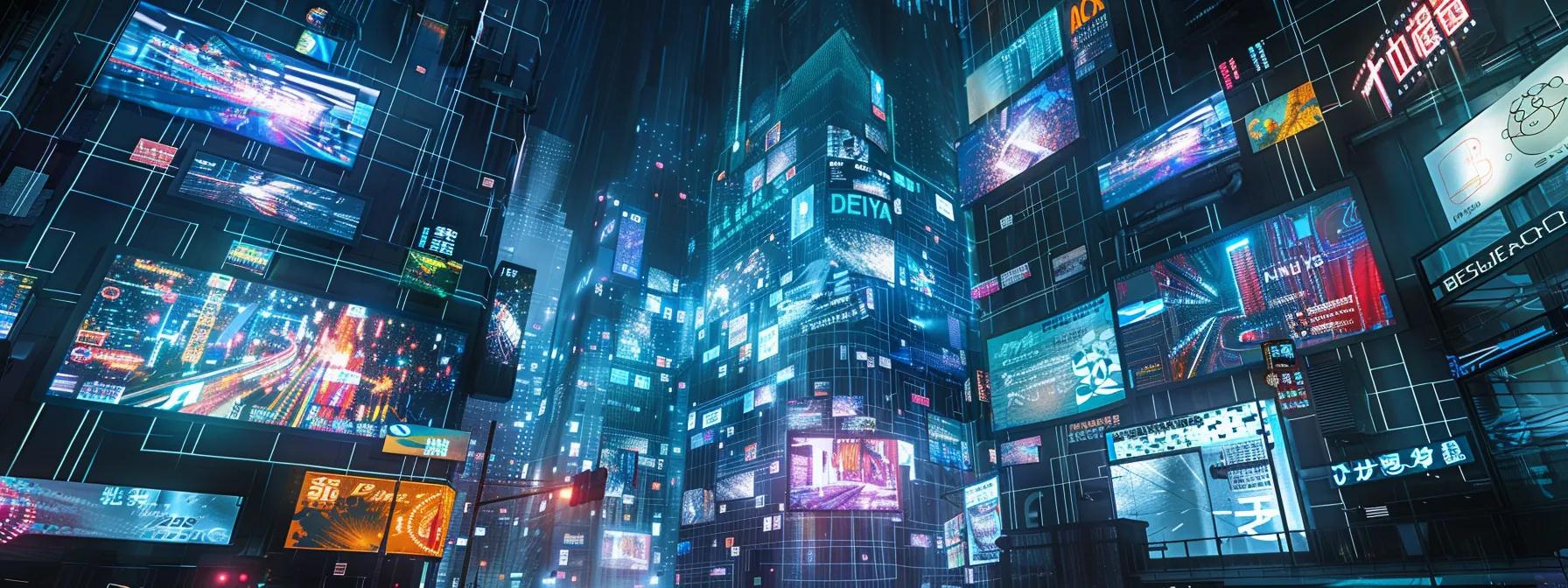
Tracking budget allocation and spending efficiency is critical for measuring success in AI ad campaigns. This section covers calculating cost per acquisition in advertising and assessing how budget impacts overall campaign performance. By examining these advertising metrics, brands can enhance relevance and creativity within their campaigns while maintaining the optimal frequency of ad delivery.
Tracking Budget Allocation and Spending Efficiency
Tracking budget allocation and spending efficiency is crucial for gauging success in AI ad campaigns. By analyzing customer acquisition cost (CAC), marketers can effectively assess whether their investments align with campaign performance goals. For instance, using smart bidding strategies can help advertisers allocate their budget more efficiently, ensuring that each dollar spent contributes to desired outcomes.
A thorough evaluation of budget allocation paired with insights from a customer data platform (CDP) can provide clarity on how campaign performance is influenced by spending decisions. By examining various marketing campaigns and their respective costs, brands can make informed adjustments to enhance both efficiency and effectiveness in their advertising efforts:
- Assess customer acquisition cost for each campaign.
- Utilize smart bidding to optimize budget utilization.
- Leverage insights from a customer data platform.
- Analyze campaign performance relative to spending efficiency.
Calculating Cost Per Acquisition in Advertising
Calculating cost per acquisition (CPA) is a crucial aspect of measuring success in digital advertising. This metric helps determine how much income is generated for each customer acquired, providing valuable insights into the effectiveness of marketing strategies. By leveraging algorithms designed to analyze user behavior and pageviews, marketers can optimize their campaigns to lower costs while maximizing the return on investment.
For instance, if a company spends $1,000 on a campaign that generates 100 leads, the CPA would be $10 per lead. Understanding this figure assists organizations in assessing their budget allocation and guiding future advertising efforts. By focusing on CPA, marketers can refine their tactics to ensure resources are utilized effectively and results are aligned with overall business objectives:
- Define the total campaign costs.
- Track the number of conversions or leads generated.
- Calculate CPA to evaluate financial efficiency.
- Adjust strategies based on CPA results for improved outcomes.
Assessing Budget Impact on Campaign Performance
Assessing the budget impact on campaign performance is essential for any effective marketing strategy. Analyzing how resources are allocated can reveal trends that directly influence return on investment, allowing advertisers to determine which channels provide the best results. For instance, if an ad campaign generates a high volume of leads but also demonstrates significant inefficiencies, this discrepancy can indicate areas needing adjustment to improve overall reputation and effectiveness.
Marketers must remain vigilant about potential ad fraud that could inflate perceived campaign success and mislead decision-making. By closely examining metrics derived from web page engagements and conversions, organizations can refine their strategies and redistribute their budgets toward initiatives that deliver measurable value. This comprehensive approach not only maximizes return on investment but also ensures sustainable growth and long-term success in an increasingly competitive advertising environment.
Budget shapes strategy, but results matter more. A clear report to stakeholders transforms numbers into meaning, guiding future choices.
Reporting Metrics to Stakeholders Effectively
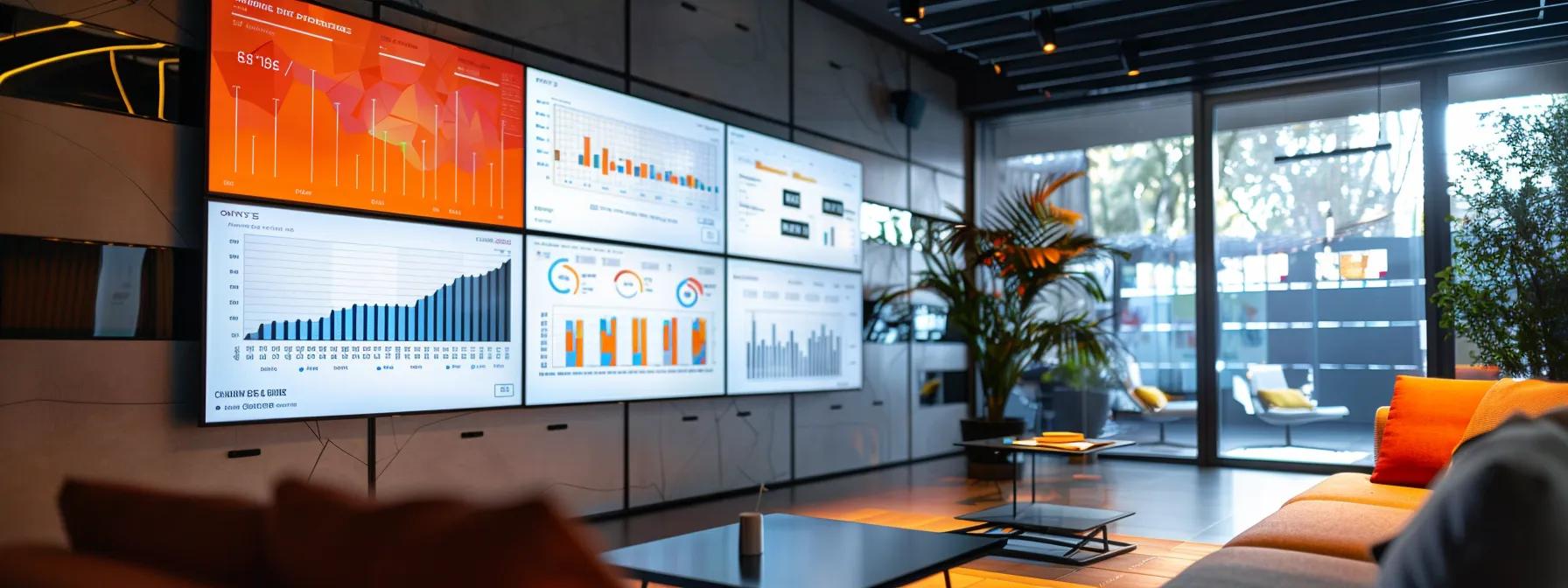
Creating clear and informative performance reports is essential for communicating the effectiveness of an advertising campaign. Tailoring reports for different audience types ensures that stakeholders grasp critical insights, whether they focus on marketing channels or social media. Additionally, visualizing data through automation and machine learning techniques can enhance understanding, making it easier to convey the success metrics needed for strategic decision-making.
Creating Clear and Informative Performance Reports
Creating clear and informative performance reports is essential for understanding campaign effectiveness in AI ad initiatives. By focusing on key touchpoints, advertisers can highlight how various interactions contribute to overall success. Utilizing data from sources like Google Ads data, marketers can present actionable insights that cut through the noise, illustrating performance trends and areas for improvement across different channels.
Effective reporting not only communicates results but also addresses stakeholder needs by providing a comprehensive overview of each campaign‘s impact. Incorporating visual elements and clear metrics helps stakeholders grasp the relationship between search engine performance and ad strategies. This clarity allows for more informed decision-making and facilitates adjustments that enhance future campaigns.
Tailoring Reports for Different Audience Types
Tailoring reports for different audience types is vital for effectively communicating the performance of AI advertising campaigns. Each stakeholder, whether they are executives, marketing teams, or content creators, requires specific insights that align with their responsibilities. For example, executives may focus on high-level analytics and overall ROI, while marketing teams might require detailed breakdowns of search ads performance and engagement metrics for informed decision-making.
By using data visualization tools, marketers can present complex information in a digestible format tailored to each audience’s comprehension levels. Incorporating insights from deep learning algorithms can enhance the relevance of the data displayed, ensuring that stakeholders understand the value generated from content creation efforts. Understanding these varied requirements not only improves transparency but also fosters collaboration across teams, guiding them in optimizing advertising campaigns.
- Identify the specific needs of each audience type.
- Utilize data visualization tools for clarity.
- Incorporate insights from deep learning.
- Present content creation outcomes effectively.
- Foster collaboration through transparent reporting.
Visualizing Data for Better Understanding
Visualizing data plays a crucial role in comprehending the effectiveness of AI ad campaigns, particularly when addressing issues like banner blindness. By presenting key parameters through engaging visuals, stakeholders can better understand customer behavior and engagement levels. For instance, infographics and charts that showcase predictive analytics can highlight trends in viewer interactions, allowing marketers to tailor their strategies for improved personalization and increased visibility.
Moreover, effective data visualization encourages stakeholders to make informed decisions based on presented customer data. Simple, intuitive graphics enable teams to grasp the impact of various campaign elements, supporting strategic discussions on optimization. Understanding these insights not only mitigates confusion but also empowers advertisers to refine their approaches, maximizing the success of their AI ad initiatives while addressing customer needs more effectively.
The landscape of AI advertising is shifting. To stay ahead, advertisers must anticipate the metrics that will shape the future.
Preparing for Future Trends in AI Advertising Metrics
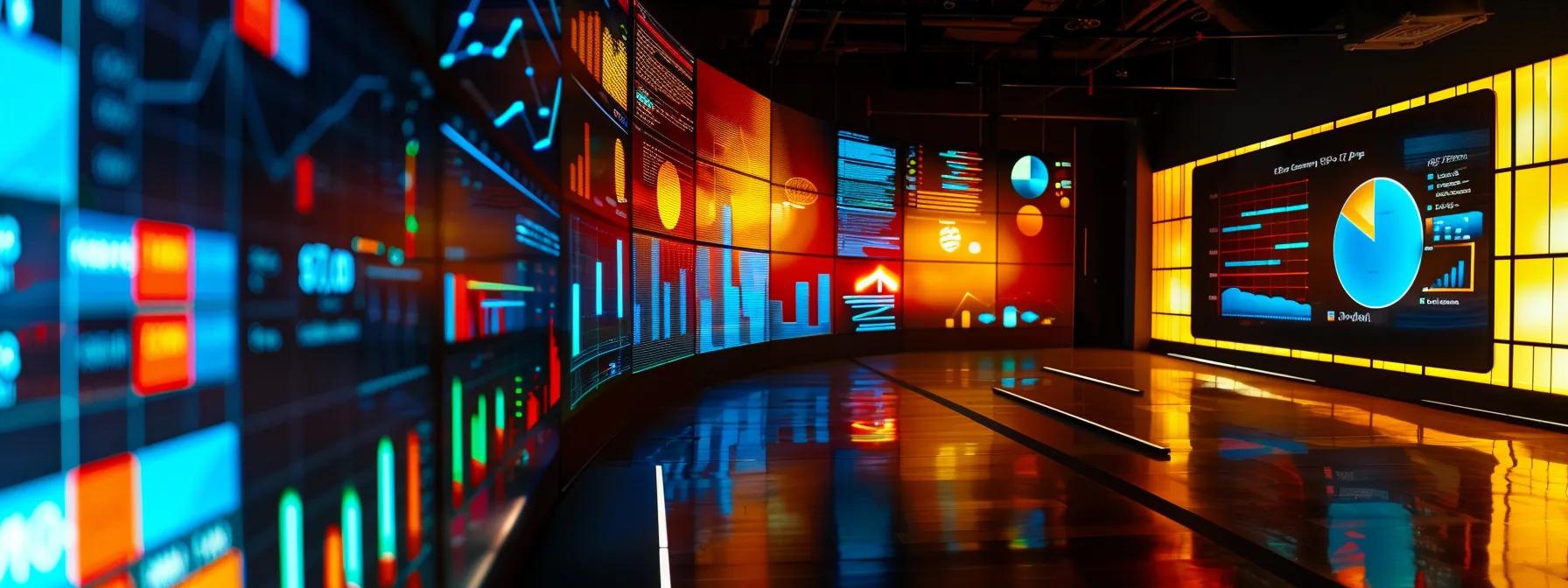
To successfully measure outcomes in AI ad campaigns, staying updated with emerging metrics and technologies is essential. This includes integrating customer feedback into measurement strategies to enhance content marketing efforts. Additionally, advertisers must anticipate changes in AI regulation and compliance to ensure alignment with industry standards. Each of these elements plays a crucial role in adapting and optimizing campaign success moving forward.
Staying Updated With Emerging Metrics and Technologies
Staying updated with emerging metrics and technologies is vital for marketers aiming to measure success in AI ad campaigns. As advertising evolves, new tools and metrics surface that can significantly enhance campaign effectiveness. By actively engaging with industry publications, webinars, and networking events, marketers can gain insights into the latest advancements, ensuring that their strategies remain relevant and impactful.
For instance, incorporating machine learning technologies can refine audience targeting and optimize ad placements in real-time. Advertisers should also consider integrating customer feedback mechanisms to assess the effectiveness of ads more accurately. Embracing these innovations not only enhances analysis but also supports the continuous improvement of campaign strategies:
- Monitor industry changes and innovations.
- Integrate machine learning into ad strategies.
- Incorporate customer feedback for better precision.
Integrating Customer Feedback Into Measurement Strategies
Integrating customer feedback into measurement strategies is essential for enhancing the effectiveness of AI ad campaigns. Marketers can gather insights directly from consumers through surveys, social media interactions, and comment sections, allowing for a comprehensive understanding of audience sentiments. By analyzing this feedback, advertisers can refine their messaging and improve user experiences, ultimately increasing engagement and conversion rates.
Furthermore, leveraging customer feedback enables marketers to identify trends and preferences that may not be captured through traditional metrics alone. For example, understanding which aspects of an advertisement resonate with consumers can inform adjustments to ad creatives and targeting strategies. This approach not only supports better decision-making but also builds a more personalized connection with the audience, enhancing overall campaign success in the competitive landscape of AI advertising.
Anticipating Changes in AI Regulation and Compliance
Anticipating changes in AI regulation and compliance is essential for marketers looking to measure success in AI ad campaigns effectively. As regulatory frameworks evolve, organizations must adapt their strategies to remain compliant while leveraging AI technologies. For example, understanding new data protection laws can guide how customer data is collected and utilized, ensuring that advertising practices align with legal requirements and ethical standards.
Marketers should actively monitor developments in AI legislation and incorporate compliance checks into their campaign strategies. This proactive approach allows businesses to mitigate risks associated with non-compliance, which can lead to costly penalties or damage to brand reputation. By fostering a culture of compliance and staying informed, advertisers can not only enhance their operational effectiveness but also build stronger trust with their audience, leading to improved engagement and long-term success in their AI-driven marketing efforts.
Conclusion
Measuring success in AI ad campaigns is crucial for optimizing performance and ensuring effective resource allocation. By analyzing key metrics such as click-through rates, conversion rates, and audience engagement, advertisers can refine their strategies to achieve maximum impact. Additionally, integrating customer feedback and staying updated on emerging technologies enhances campaign relevance and effectiveness. Ultimately, a data-driven approach empowers marketers to make informed decisions that lead to sustained growth and improved return on investment.