Are you struggling to find the right AI ad generator that suits your budget while maximizing advertising revenue? With the rapid rise of UGC ads and various platforms offering different SaaS pricing strategies, navigating these options can be challenging. This article will break down key differences in pricing models across major AI ad platforms, highlight distinct pricing features, and analyze factors influencing costs. By understanding these elements, readers will gain valuable insights to make informed decisions, ultimately enhancing their advertising capabilities through effective deep learning applications.
Key Takeaways
- Understanding pricing models aids advertisers in selecting the right AI ad generator solutions
- Customization and integration can significantly impact overall costs and campaign effectiveness
- Auction-based pricing models enhance budgeting decisions based on real-time performance metrics
- Advanced analytics in pricing strategies improve adaptability and relevance of advertisements
- Staying informed about regulatory changes is essential for effective budgeting in advertising campaigns
Understanding the Importance of Pricing Models in AI Ad Generation
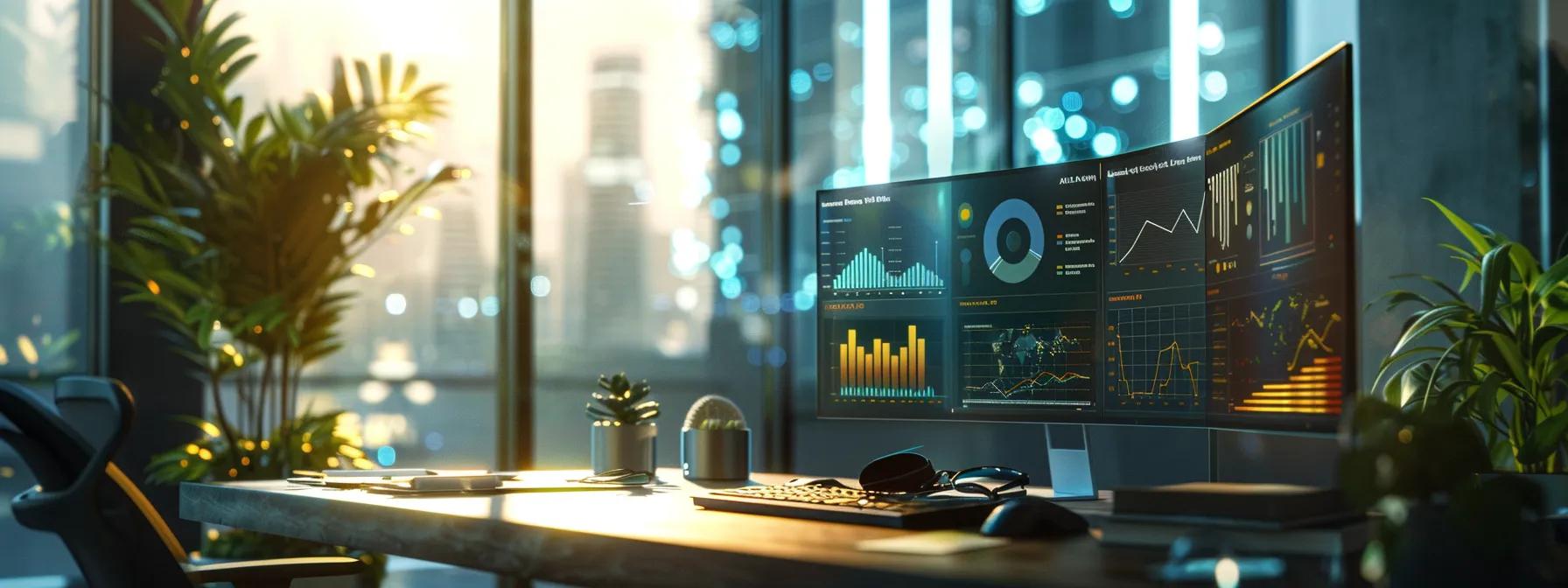
AI ad generators play a crucial role in modern advertising campaigns, offering diverse functionalities and benefits, including ugc ads. Understanding various pricing models, such as penetration pricing or flat rate options, is essential for users as they directly influence business strategies. This section will explore how different pricing strategies impact disk storage usage and the sequence of campaign execution, providing practical insights for advertisers.
Overview of AI Ad Generators and Their Uses
AI ad generators utilize machine learning to create targeted advertisements efficiently. These platforms analyze user data to produce ads that resonate with specific audiences, enhancing overall campaign performance. The average user values these tools for their ability to streamline the ad creation process, ultimately saving time and resources.
Different pricing strategies are employed by AI ad generator platforms, impacting user decisions based on their reputation and offered features. For instance, a flat rate model may appeal to businesses seeking predictable budgets, while a usage-based model might attract those prioritizing value and scalability. Understanding these dynamics helps advertisers select solutions that align with their objectives and budget constraints.
Why Pricing Models Matter for Users
The choice of pricing models in AI ad generation impacts users significantly, as it affects their budgeting and cash flow management. For businesses in North America, selecting the right product could mean the difference between overspending on unnecessary features and maximizing return on investment. Software as a service (SaaS) platforms often offer varied pricing structures, allowing users to tailor their expenditure based on specific functions and usage patterns.
Understanding these pricing models fosters better decision-making for advertisers. Users can assess which model aligns with their business goals, such as whether a subscription service offers long-term savings or if a pay-per-use option fits their project scale. By considering factors like cash flow and the specific functions needed, advertisers can effectively choose the best AI ad generator for their campaigns:
- Evaluate pricing structures based on budget constraints.
- Identify the necessary features for optimal ad generation.
- Consider the potential return on investment from chosen models.
Impact of Pricing on Business Strategies
The pricing models chosen for AI ad generation have a direct influence on the overall marketing strategy of businesses, especially in the realm of social media marketing. For instance, platforms adopting a subscription-based pricing may establish a predictable expenditure that allows companies to allocate resources more effectively, minimizing overspending on unnecessary features. Conversely, usage-based models can provide flexibility, enabling businesses to optimize their campaigns in line with their target market‘s behavior, enhancing observability and responsiveness.
Furthermore, understanding and utilizing the appropriate pricing strategy can lead to opportunities for upselling additional features or services, thus maximizing profitability. By analyzing the specific needs of their target market and aligning them with the appropriate pricing structure, organizations can develop compelling marketing strategies that resonate with customers while maintaining budgetary control. This approach ultimately supports the growth of their advertising efforts and ensures a more robust return on investment.
- Identify key pricing models impacting marketing strategies.
- Assess how pricing affects resource allocation in campaigns.
- Explore opportunities for upselling when selecting AI ad generators.
Pricing models shape how advertisers navigate the landscape of AI ad generation. Now, let’s explore the unique pricing features offered by major platforms and see what sets them apart.
Examining Distinct Pricing Features Across Major AI Ad Platforms
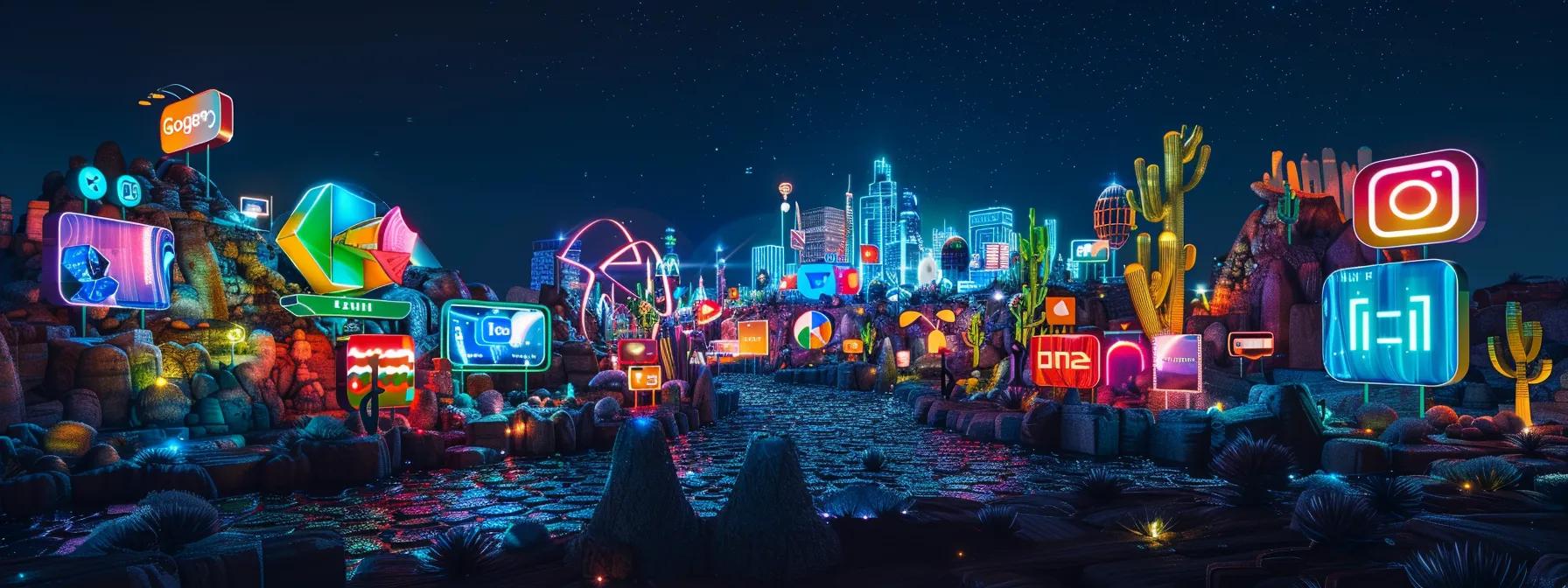
This section examines the distinct pricing features of major AI ad platforms. It will provide insights into Google Ads AI features and pricing structure, offer an overview of OpenAI’s ad generation pricing, and compare the pricing models of Microsoft Advertising. Additionally, the unique pricing characteristics of Facebook Ads AI will be highlighted, emphasizing the impact of these models on brand awareness and consumer demand.
Google Ads AI Features and Pricing Structure
Google Ads utilizes advanced AI features to enhance its search engine marketing capabilities, ensuring targeted ads reach the right audience effectively. The integration of chatbots within Google Ads facilitates seamless interaction with potential customers, addressing inquiries in real-time while optimizing the ad workflow. By analyzing the price elasticity of demand, advertisers can adjust their bids based on the responsiveness of their target market, ensuring optimal budget allocation for maximum return.
The pricing structure of Google Ads is designed to offer flexibility, with options that cater to various business needs. Advertisers can leverage virtual machine technology to run simulations, helping them understand how their advertising budget may perform under different scenarios. This strategic approach allows companies to fine-tune their campaigns based on real-time data, ultimately driving better results while managing costs efficiently.
OpenAI's Ad Generation Pricing Overview
OpenAI’s ad generation pricing utilizes a dynamic pricing system, which adjusts costs based on various factors, including demand and user engagement. This approach allows advertisers to optimize their search advertising strategies by aligning expenditure with the perceived value proposition of their campaigns. For example, businesses can set parameters based on their IP address to target specific demographics, ensuring budgets are allocated efficiently and effectively.
By leveraging OpenAI’s capabilities, companies can execute campaigns with a focus on real-time adjustments, enhancing their ability to respond to market trends. The pricing model promotes adaptability, allowing advertisers to scale efforts based on performance metrics, ultimately maximizing return on investment. This system not only supports varied strategies but also encourages a deeper understanding of audience behaviors relevant to search advertising.
Comparing Pricing Models of Microsoft Advertising
Microsoft Advertising employs a range of pricing models designed to optimize resource allocation for advertisers. One notable approach includes psychological pricing strategies aimed at providing perceived value to retail advertisers by allowing them to set competitive bids that resonate with their target market. This method can be particularly effective in enhancing the performance of landing pages, as well-crafted bids can directly influence consumer behavior and the ultimate success of ad campaigns.
Another key feature of Microsoft Advertising’s pricing model involves the use of server-driven bidding mechanisms that adjust costs based on real-time performance metrics. This flexibility empowers advertisers to refine their strategies continuously, ensuring that budgets align with changing market demands and user engagement. By adapting pricing according to these insights, businesses can maximize their advertising effectiveness while achieving a significant return on investment.
Unique Pricing Features of Facebook Ads AI
Facebook Ads AI provides a unique pricing structure that emphasizes innovation and data analysis to enhance advertising effectiveness. Advertisers can take advantage of advanced audience targeting options, which allow for more precise ad placements based on user interests. This level of research enables businesses to optimize their campaigns, ultimately driving higher profit margins as they reach the right consumers without overspending on broad targeting.
Scalability is another critical aspect of Facebook Ads AI’s pricing features, enabling advertisers to adjust their spending according to campaign performance. By utilizing real-time analytics, businesses can swiftly modify their strategies based on user engagement and market trends. This adaptability ensures that advertising budgets remain aligned with campaign goals, maximizing ROI and allowing companies to focus on what truly drives results.
Understanding the distinct pricing features of major AI ad platforms lays the groundwork for a deeper look. Next, the focus shifts to how these pricing models differ between AI ad generators, revealing their impact on advertisers.
How Do Pricing Models Differ Between AI Ad Generators?
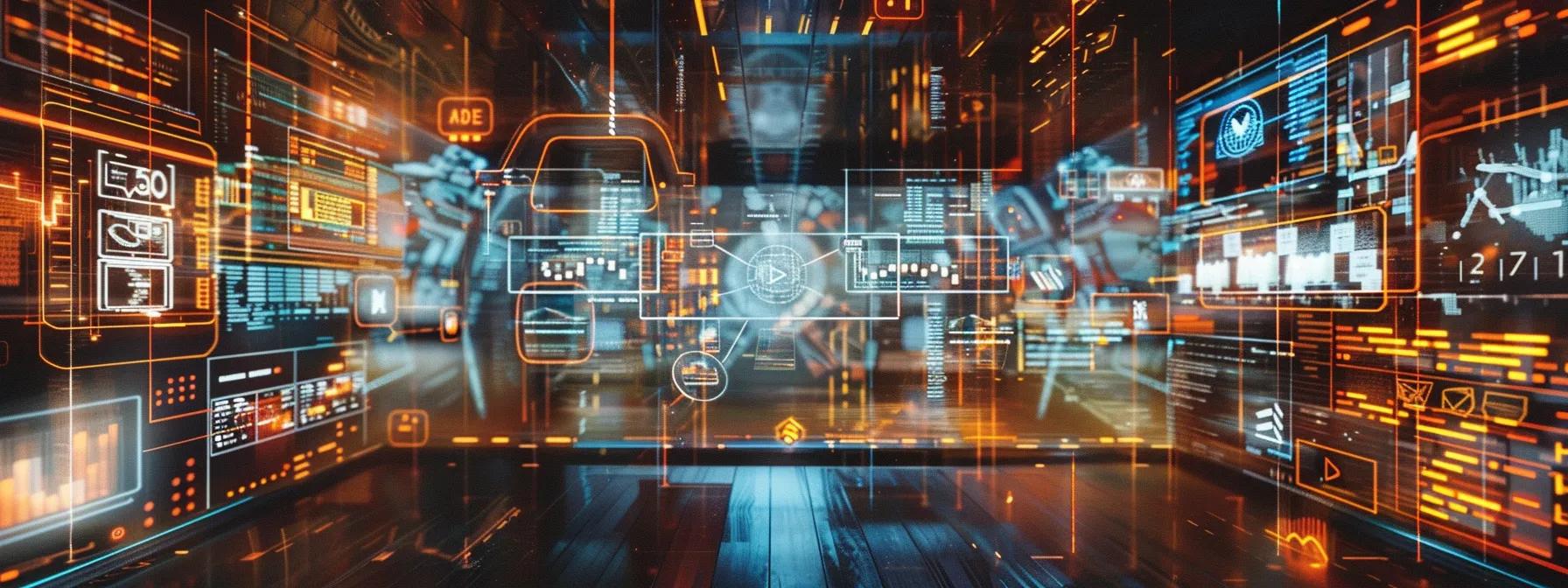
Pricing models for AI ad generators vary significantly, with distinctions between flat rate and pay-per-usage models influencing overall expenditure. Subscription-based pricing presents predictable costs, while tiered pricing structures allow for adaptability according to usage levels. Understanding these aspects helps advertisers mitigate risk and manage latency, especially regarding content marketing initiatives that leverage natural language processing and cost per mille effectiveness.
Flat Rate vs. Pay-Per-Usage Models
Flat rate pricing models provide customers with predictable costs, enabling budget management and resource allocation without unexpected expenses. This structure can enhance user experience by ensuring visibility into costs, which may appeal to businesses that prefer financial stability over fluctuating expenses. For instance, a company can effectively plan their advertising budget around fixed costs, facilitating a strategic approach to their marketing efforts.
In contrast, pay-per-usage models, also known as usage-based pricing, allow businesses to pay based on their engagement levels. This approach can adapt more easily to variations in customer behavior, providing flexibility in advertising efforts. Companies that experience changing demand or have varying campaign needs can maximize efficiency and adjust expenditures based on insights gained from real-time analytics and user interactions.
Subscription-Based Pricing Explained
Subscription-based pricing in AI ad generators presents a consistent cost structure that empowers businesses to effectively manage their advertising budgets. Through this model, organizations can allocate resources more efficiently, aligning their strategy with long-term goals while minimizing financial uncertainties. For example, a manufacturing firm utilizing subscription-based services can adopt AI-driven intelligence to enhance their consumer outreach without incurring unexpected costs.
This pricing approach also grants advertisers access to premium features that can be leveraged to improve campaign execution. By entering into a subscription agreement, companies can benefit from regular updates and innovations, maintaining leadership in a competitive market. As they embrace this model, they can focus on refining their advertising tactics, ultimately enhancing their engagement and conversion rates across diverse consumer segments.
Tiered Pricing Structures and Their Implications
Tiered pricing structures offer businesses the flexibility to select a plan that aligns with their operational needs and budgetary constraints. By providing different levels of service based on usage, companies can optimize their total cost of ownership while ensuring that their revenue generation remains robust. For example, businesses leveraging platforms like Microsoft Azure benefit from these structures, effectively managing cloud storage costs and scaling resources as required.
This pricing approach not only helps organizations allocate resources strategically but also enhances their market share by attracting a broader audience. As advertisers gain insights on their campaign performance, they can make informed decisions to adjust their spending and maximize the effectiveness of their advertising efforts. Businesses focusing on tiered pricing can thus refine their strategies and better respond to dynamic market demands:
- Flexible resource allocation allows for improved cost management.
- Scalable service offerings enhance revenue potential.
- Insights from campaign performance inform future spending strategies.
The costs of AI ad generation are influenced by many factors. By examining these, advertisers can make smarter choices.
Analyzing Cost Factors in AI Ad Generation Services
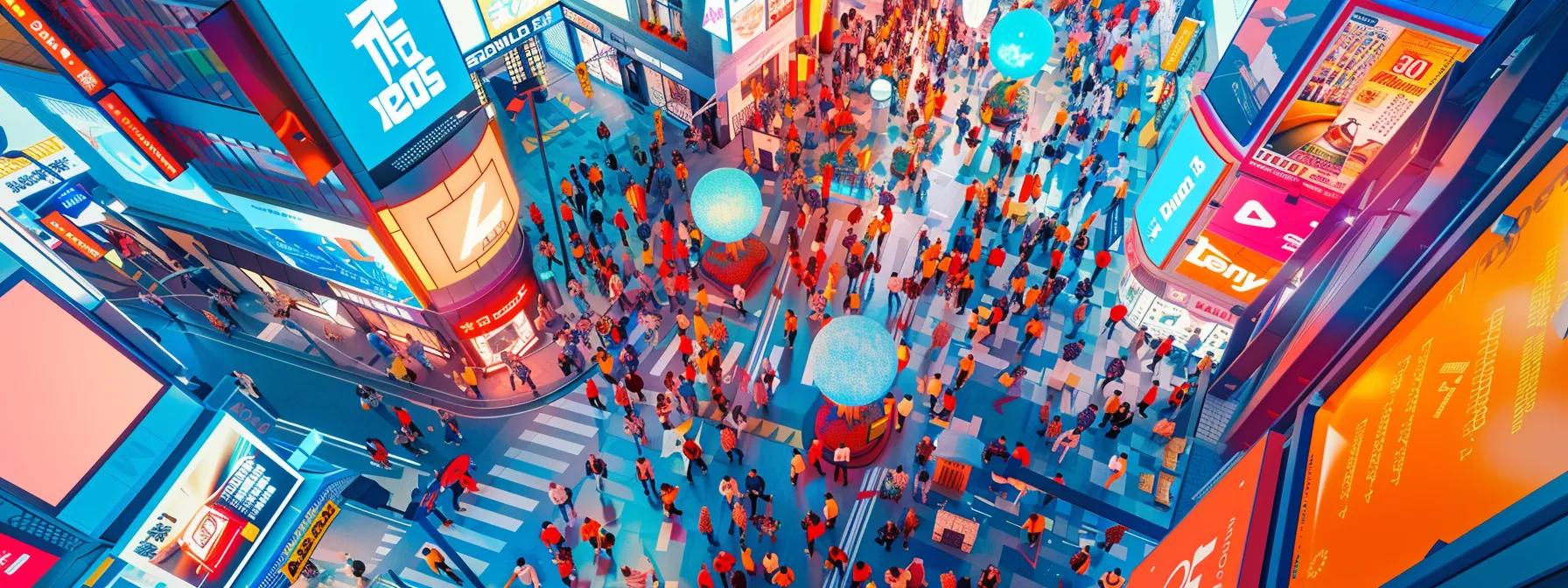
The cost factors in AI ad generation services are shaped by several key elements that impact overall pricing and effectiveness. Ad volume significantly influences price, determining the level of investment required for campaigns. Customization options can alter costs, reflecting user preference for tailored solutions. Additionally, integration with other marketing tools often entails extra expenses, making it vital for advertisers to consider analytics and inventory management when evaluating saas pricing models.
Influence of Ad Volume on Pricing
The volume of ads significantly influences the pricing structures offered by AI ad generators, acting as an essential unit of measurement in determining costs. Higher ad volumes typically lead to increased efficiencies within the underlying algorithms, allowing platforms to offer options that provide better value as campaigns scale. For instance, advertisers leveraging bulk ad creation may enjoy tiered pricing discounts, enabling them to enhance customer engagement without substantially elevating their overall expenditure.
In the realm of online advertising, understanding how ad volume impacts pricing is crucial for strategic budgeting. Advertisers must evaluate their expected output and the associated costs to determine the most effective pricing model for their campaigns. By thoroughly examining the relation between ad volume and potential pricing variations, advertisers can make informed decisions that align their spending with their business objectives and maximize the effectiveness of their customer engagement strategies:
- Volume of ads serves as a key factor in determining overall costs.
- Algorithms adjust pricing based on efficiencies related to ad quantity.
- Bulk ad purchases often come with pricing incentives and discounts.
- Understanding this relationship aids in strategic budgeting for campaigns.
- Effective pricing models can enhance customer engagement and optimize ad performance.
Role of Customization in Pricing
Customization plays a significant role in the pricing of AI ad generation services. Platforms often offer various licensing options that allow businesses to tailor their usage according to specific needs. For instance, organizations seeking advanced features such as sentiment analysis can expect to pay more, as these functionalities enhance campaign effectiveness through improved audience targeting and message resonance.
Understanding how customization affects pricing enables businesses to make informed decisions aligned with their marketing strategies. For example, companies that utilize predictive analytics to forecast campaign performance might find customized packages beneficial for their accounting and budgeting purposes. This strategic investment in tailored solutions can ultimately lead to more successful advertising outcomes:
- Customization enhances campaign effectiveness through tailored features.
- Licensing options allow firms to adjust their usage based on needs.
- Sentiment analysis can improve audience targeting and messaging.
- Predictive analytics aids in strategic budgeting and decision-making.
- Understanding customization benefits leads to informed investment choices.
Integration With Other Marketing Tools and Its Cost
Integration with other marketing tools can significantly impact the overall cost of using AI ad generation services. For instance, tools like Sprout Social allow organizations to combine their advertising efforts with social media management, providing a comprehensive approach to reach their target audience effectively. However, this integration often comes with additional expenses that can affect the organization’s budget, making it essential for businesses to evaluate whether the benefits outweigh the costs, especially concerning their customer base.
Understanding the cost implications of integrating AI ad generators with existing marketing tools is crucial for effective financial planning. Organizations should consider potential expenses associated with software compatibility and support services when assessing pricing structures. Investing in integrated solutions can enhance campaign performance and audience targeting but requires careful analysis to ensure that they align with the organization‘s overall marketing strategy and goals.
The numbers tell a tale of their own, revealing both immediate expenses and hidden costs along the way. Next, the focus shifts to what these figures mean for the future, guiding advertisers towards lasting value in AI ad generation.
Evaluating the Long-Term Value of AI Ad Generation Pricing
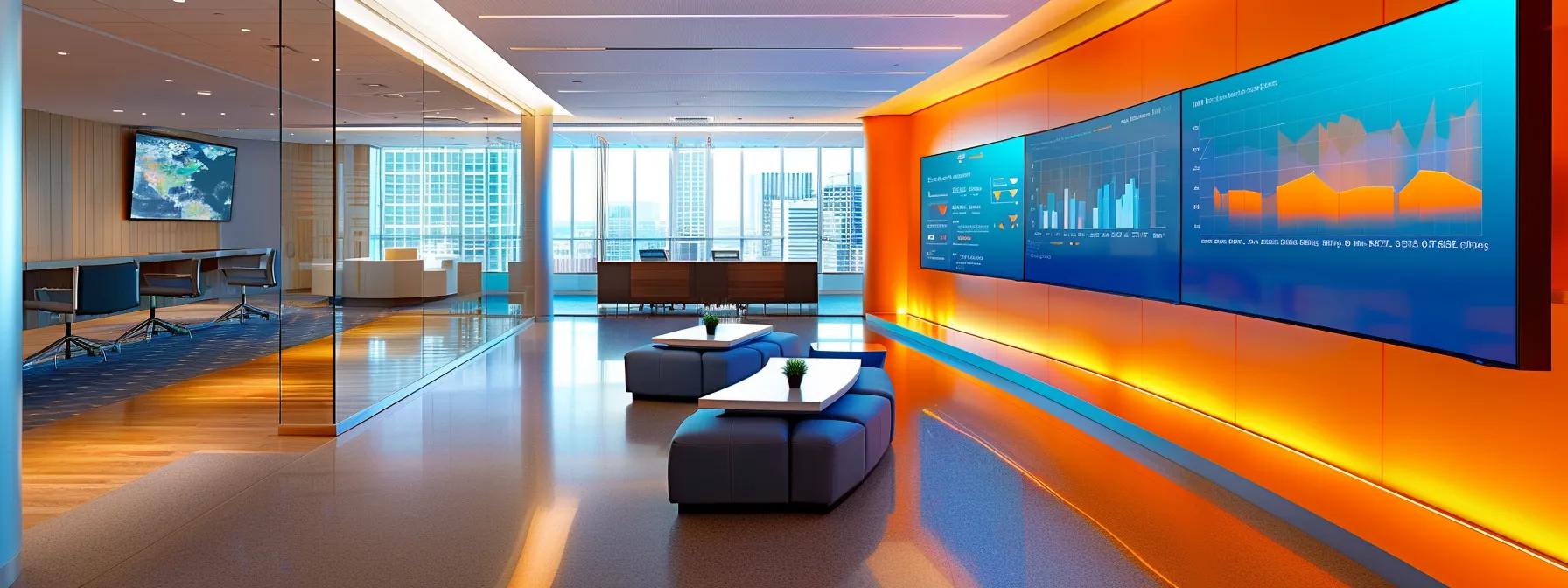
Analyzing the long-term value of AI ad generation pricing encompasses several key components. A cost-benefit analysis of different platforms highlights the fee structures and infrastructure considerations that impact overall campaign efficiency. Additionally, evaluating the return on investment from AI-driven ad campaigns is essential for maximizing quality score and effectiveness. Understanding the total cost of ownership for generative artificial intelligence solutions enables businesses to make informed decisions that align with their marketing strategies.
Cost-Benefit Analysis of Different Platforms
Conducting a cost-benefit analysis of various AI ad generation platforms allows businesses to identify which service provides the best value in relation to financial investment. Understanding pricing models, such as those offering credits or incentives for higher engagement rates, equips advertisers to make informed decisions that can significantly impact profit margins. For example, a platform that offers trial credits can encourage experimentation with different ad formats, leading to a better understanding of what resonates with the target audience.
Moreover, customer service plays a pivotal role in assessing the long-term value of these platforms. Exceptional support can enhance the user experience, ensuring that advertisers have the resources they need to troubleshoot and optimize their campaigns effectively. By comparing the cost structures and support services of various AI ad generators, businesses can align their expenditure with their strategic goals, ultimately improving their overall advertising effectiveness.
Return on Investment for AI-driven Ad Campaigns
The return on investment (ROI) for AI-driven ad campaigns can be significantly influenced by the underlying architecture of the platforms utilized. For instance, leveraging the Google Cloud Platform allows advertisers to harness powerful data analytics and machine learning capabilities, optimizing the parameters that drive campaign success. This integration not only enhances targeting accuracy but also supports a scalable ecosystem that can adapt to fluctuating market demands, thereby maximizing returns.
Premium pricing strategies in AI ad generators often reflect the advanced features and support they offer, which can result in higher ROI for users willing to invest. By choosing platforms that provide robust analytics and real-time data insights, advertisers can make informed decisions that improve campaign performance. The resulting feedback mechanisms facilitate continuous improvement, allowing businesses to better align their marketing strategies with consumer needs while enhancing overall profitability.
Assessing the Total Cost of Ownership for AI Ad Generators
Assessing the total cost of ownership for AI ad generators is essential for organizations engaged in digital marketing. A comprehensive understanding of costs includes not only the subscription fees or pay-per-use charges but also the time spent on integrating these tools into existing workflows. For instance, using platforms that leverage Google Cloud for their infrastructure can provide robust data analytics, thus potentially reducing the hours needed for campaign analysis and execution while positively impacting their search engine results page (SERP) performance.
Furthermore, companies must consider the long-term benefits that effective AI ad generation brings to their social media strategies. By evaluating ongoing costs against the improvements in ad performance and customer engagement, businesses can better justify their investments. For example, a platform that enhances campaign efficiency can lead to better audience targeting and higher returns, making the overall cost more manageable and worthwhile in the context of sustained digital marketing initiatives.
As the landscape shifts, new pricing models emerge on the horizon. What lies ahead in AI ad generation pricing offers both challenge and opportunity for advertisers.
Future Trends in AI Ad Generator Pricing Models
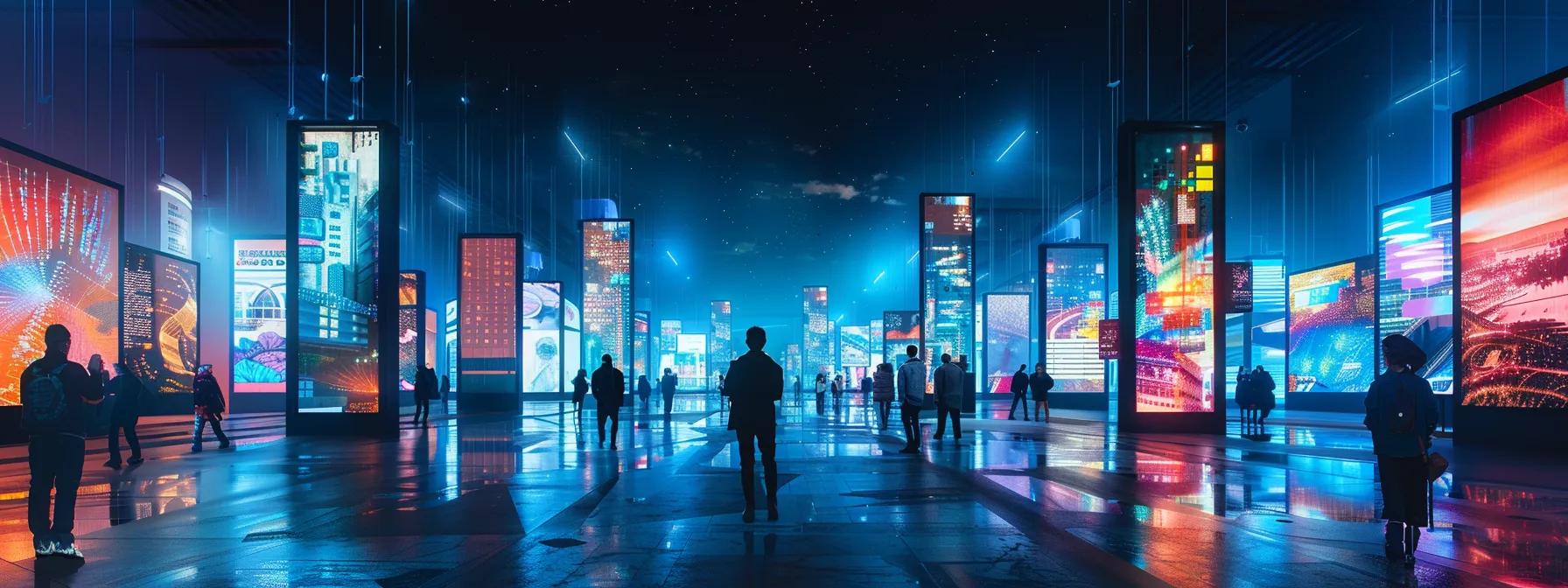
Anticipated changes in pricing strategies for AI ad generators are increasingly influenced by automation and emerging technologies. As adoption of sophisticated devops methodologies grows, advertisers may see new auction-based pricing models that enhance cost efficiency. Furthermore, regulatory factors will play a crucial role in shaping these pricing frameworks, ensuring alignment with industry standards and best practices.
This section will delve into how these trends are expected to reshape the ad generation landscape, providing practical insights on their implications for pricing and overall campaign effectiveness.
Anticipated Changes in Pricing Strategies
As the advertising landscape evolves, AI ad generator pricing strategies are expected to undergo significant changes, primarily driven by the growing demand for transparency and efficiency. Currency fluctuations and increased attention to measurement precision can lead to the development of auction-based pricing models that allow advertisers to make better budgeting decisions based on real-time volume and performance metrics. These models can enhance the relevance of ads while addressing the typical churn rate associated with traditional pricing approaches.
Moreover, the integration of advanced analytics is anticipated to shape how AI ad platforms approach pricing. Advertisers will benefit from dynamic pricing structures that adjust to changes in user engagement and market demand. By focusing on cost efficiency and adaptability, platforms can improve their service offerings, ensuring that businesses receive maximum value for their campaigns. This strategic shift may include various forms of tiered pricing options aimed at optimizing resource allocation:
- Adoption of auction-based pricing models for improved budgeting.
- Increased transparency concerning currency and measurement adjustments.
- Dynamic pricing structures reflecting real-time engagement metrics.
- Enhanced relevance of ads by addressing churn rate concerns.
- Focus on optimizing resource allocation through tiered pricing.
Emerging Technologies and Their Impact on Costs
Emerging technologies like Kubernetes are transforming the landscape of AI ad generation, particularly in terms of cost efficiency. By facilitating resource orchestration and scaling, these technologies allow platforms to reduce operational expenses, which can often be passed on to advertisers in the form of competitive pricing. For brands looking to enhance their content creation strategies, leveraging Kubernetes can significantly streamline workflows, enabling quicker adaptations to market trends while optimizing expenditure.
As AI ad platforms integrate emerging technologies, the impact on pricing will continue to evolve. These advancements can lead to more sophisticated algorithms that improve ad targeting and personalization, ultimately enhancing user engagement and conversion rates. For advertisers, this means that investing in AI-driven solutions could result not just in better campaign performance but also in reduced costs associated with ineffective ad spend, providing a crucial advantage in a competitive market landscape.
Regulatory Factors Affecting Pricing in Ad Generation
Regulatory factors play a significant role in shaping pricing models for AI ad generation services. Governments and industry bodies often implement guidelines to protect consumer data and privacy, which can influence how advertisers allocate budgets. For instance, compliance with regulations may necessitate increased investments in data protection measures, affecting overall pricing strategies for platforms offering AI-driven advertising solutions.
As regulations evolve, AI ad generators will need to adjust their pricing structures to maintain compliance while also offering competitive rates. This rebalancing could lead to the development of new fee models that account for the costs of adhering to safety standards and compliance requirements. Advertisers must stay informed about these regulatory changes to optimize their spending and ensure their campaigns remain effective and compliant:
- Impact of consumer data protection regulations on pricing strategies.
- Necessity for compliance leading to potential adjustments in fee structures.
- Need for advertisers to stay updated on regulatory changes for effective budgeting.
Conclusion
Understanding the pricing models of AI ad generators across major platforms is essential for advertisers aiming to optimize their campaigns and budget effectively. By evaluating distinct pricing structures, such as flat rate, subscription-based, and pay-per-usage models, users can make informed choices that align with their marketing goals. This knowledge empowers businesses to maximize return on investment while leveraging advanced features tailored to their needs. Ultimately, grasping these differences ensures advertisers can navigate the complexities of AI-driven advertising and enhance their overall strategy.