Measuring the success of advertising strategies has become increasingly complex with the rise of AI-generated ads. Traditional marketing approaches often rely on established metrics, while modern AI-driven techniques introduce unique performance indicators. This article will compare key metrics for AI advertising, focusing on real-time data and analytics, and also explore how traditional marketing strategies measure success. By understanding these differences, readers will gain insights into optimizing customer retention and enhancing their brand within increasingly competitive markets. This knowledge will help advertisers efficiently filter ad performance and improve shopping cart conversions.
Key Takeaways
- AI-driven advertising campaigns adapt messaging based on real-time consumer behavior for improved engagement
- Lower customer acquisition costs can result from more targeted AI-driven marketing strategies
- Integrating predictive analytics enhances campaign outcomes by aligning advertising with consumer preferences
- Traditional marketing strategies often lack the agility to respond to real-time market shifts
- Continuous analysis of data is crucial for maintaining relevance and optimizing advertising effectiveness
Understanding the Success Metrics for AI-Driven Advertising
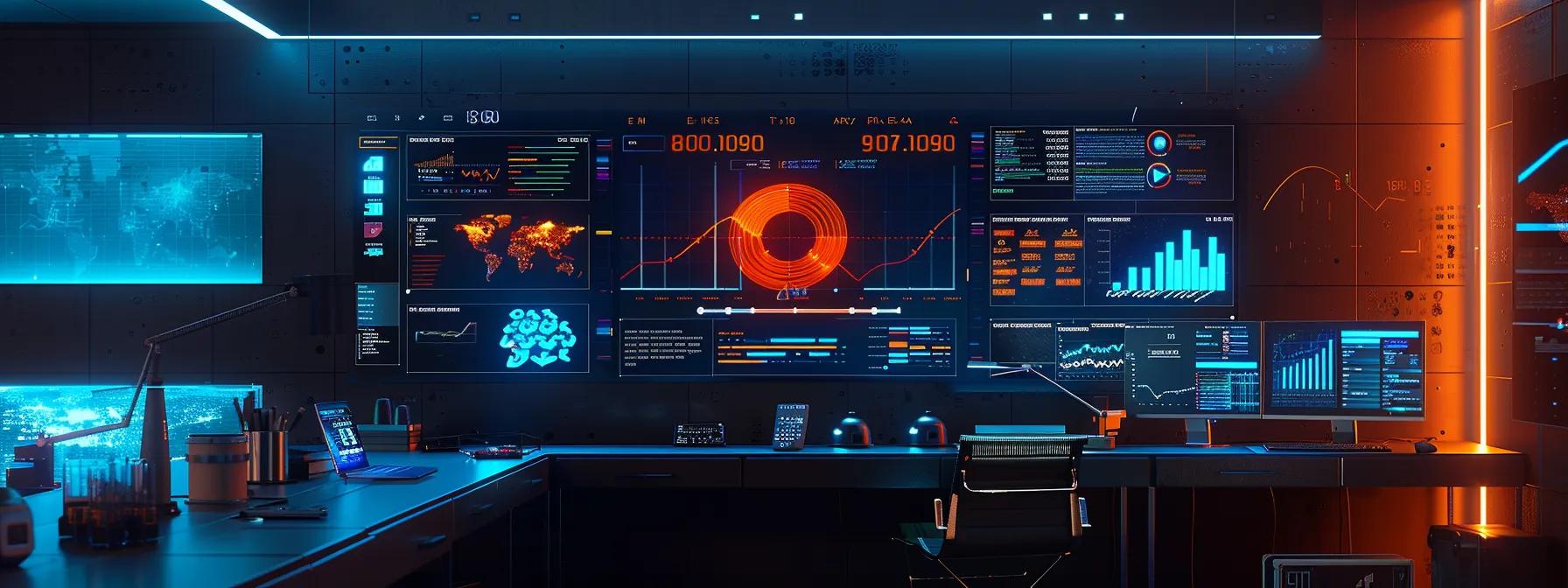
Success in advertising can be defined through various metrics that measure effectiveness. In this context, understanding the differences between AI-driven approaches, such as ugc ads, and traditional strategies is essential. Key areas of focus include tracking active users and analyzing customer data, which inform digital marketing strategies and enhance landing page performance. The subsequent sections will provide detailed insights into these distinctions and their implications.
Defining Success in the Context of Advertising
In advertising, defining success requires an understanding of various metrics that determine campaign effectiveness. AI-generated ads can provide unique insights into consumer behavior, allowing marketers to monitor key indicators such as bounce rate. By analyzing this metric, businesses can identify areas where potential customers disengage, helping them refine their landing pages and overall strategy to foster better engagement and leadership in their market niche.
Data-driven evidence plays a crucial role in assessing the performance of both AI-generated and traditional advertising methods. For instance, the integration of creative commons resources can enhance the quality of an ad campaign. By leveraging these creative materials, advertisers can produce compelling content that resonates with their audience, ultimately improving conversion rates and achieving better results compared to standard approaches.
Distinguishing Between AI-Driven and Traditional Approaches
Distinguishing between AI-driven and traditional marketing strategies reveals considerable differences in approach and effectiveness. AI-generated ads utilize advanced algorithms to analyze consumer behavior and tailor content to enhance user experience, often leading to improved engagement metrics. In contrast, traditional techniques may rely heavily on a more generalized strategy that does not adapt to real-time data, potentially missing significant opportunities to connect with consumers based on their specific interests and needs.
The truth about these two methods lies in their data utilization. AI-driven campaigns can utilize search engine data to refine targeting and personalize messaging, providing a more relevant experience for the user. Moreover, businesses that adapt their strategies to incorporate AI insights typically report higher conversion rates, demonstrating that an evidence-based approach to advertising can be more effective than conventional methods that do not leverage immediate data feedback:
- AI adapts to real-time consumer behavior for instant engagement.
- Traditional strategies often rely on outdated data and assumptions.
- Personalization in AI-driven ads enhances user experience significantly.
- Search engine insights drive better targeting in AI advertisements.
- Rapid adjustments in AI campaigns can lead to higher conversion rates.
Success in AI advertising relies on numbers. Next, the focus shifts to the key performance indicators that signal true effectiveness.
Key Performance Indicators for AI Advertising
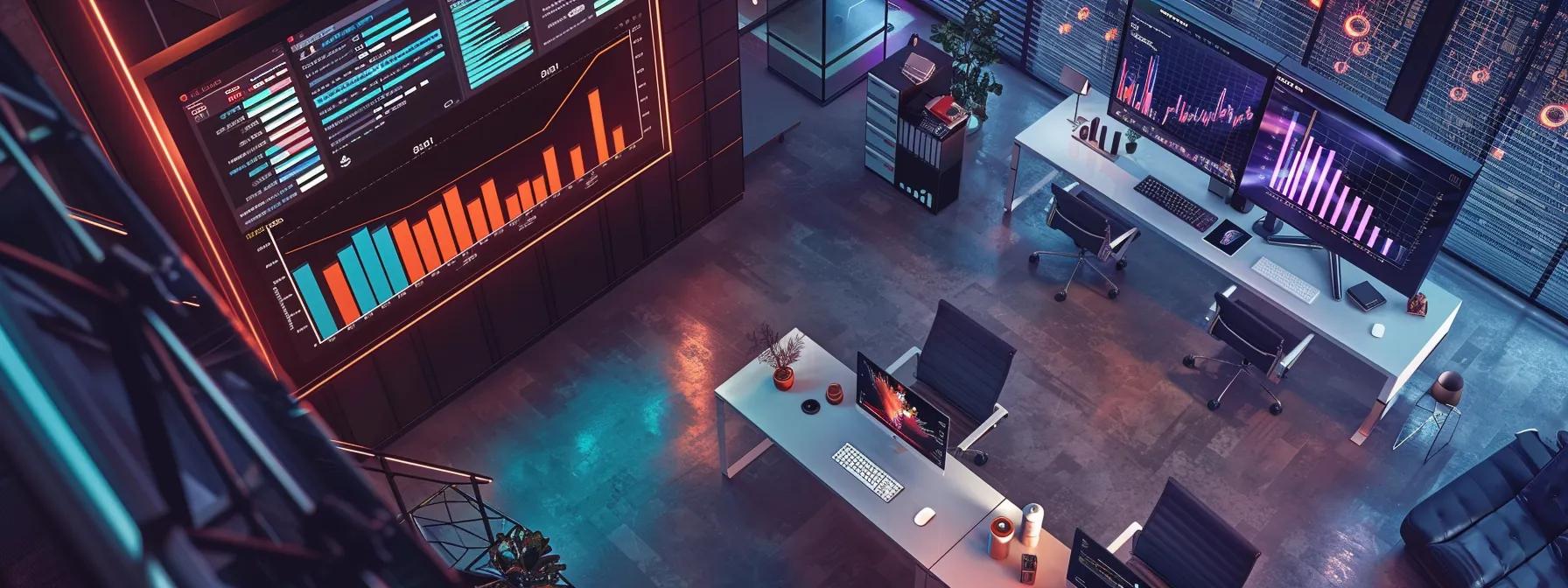
Measuring the effectiveness of AI advertising requires a closer examination of various key performance indicators. This section explores critical aspects such as return on investment for AI campaigns, engagement metrics associated with AI ads, and click-through rates comparing AI and traditional strategies. Additionally, it discusses customer acquisition costs and evaluates customer lifetime value across different approaches.
Understanding these metrics is essential for organizations seeking to leverage advanced technologies such as natural language processing and recommender systems within their advertising engine, ensuring they maximize the benefits of AI-driven strategies.
Return on Investment for AI Campaigns
Return on investment (ROI) for AI campaigns is becoming a critical metric in the retail sector, as businesses seek to understand the financial impact of their advertising efforts. By incorporating advanced automation techniques, organizations can achieve a more accurate assessment of customer acquisition costs. This allows them to refine their marketing strategies based on the average ROI generated, demonstrating how effectively AI tools drive revenue compared to traditional methods.
Establishing a conceptual framework for measuring ROI in AI advertising involves analyzing various data points that impact overall performance. Retail businesses that leverage AI technologies often see lower customer acquisition costs due to more targeted campaigns, which can lead to higher conversion rates. By focusing on the specific needs and behaviors of their audience, these companies create opportunities for improved profitability and sustainable growth over time.
Engagement Metrics Relevant to AI Ads
Engagement metrics are vital for evaluating the impact of AI-generated ads in comparison to traditional marketing strategies. Metrics such as click-through rates, interaction time, and conversion rates demonstrate how effectively digital marketing campaigns resonate with target audiences. By employing predictive analytics, marketers can identify patterns in user behavior, enhancing the relevance of their ads and leading to improved engagement levels.
To ensure ethical practices in advertising, organizations must focus on transparency regarding data usage. This approach builds trust with consumers while driving positive engagement outcomes. Additionally, optimizing for organic search results can significantly boost visibility, making it essential for advertisers to monitor engagement metrics closely:
- Click-through rates indicate initial ad effectiveness.
- Interaction time reflects user interest in content.
- Conversion rates show overall campaign success.
- Predictive analytics help tailor content to audience needs.
- Ethics in data usage fosters trust and engagement.
Click-Through Rates for AI vs. Traditional Ads
Click-through rates (CTR) serve as a critical metric for assessing the effectiveness of AI-generated ads compared to traditional marketing strategies. AI-driven approaches leverage data analytics and advanced algorithms as a sensor to understand audience behavior, enabling campaigns that resonate more powerfully and optimize revenue potential. In contrast, traditional methods often rely on guessing and generalized assumptions about consumer interests, which can lead to lower engagement levels and missed opportunities for conversion.
Furthermore, AI-generated ads frequently achieve higher click-through rates due to their ability to target specific demographics and interests, resulting in tailored messaging that speaks to the audience. This precision allows advertisers to incorporate influencer marketing strategies effectively, boosting visibility and driving traffic. As a result, organizations utilizing AI for ad creation can see a significant enhancement in both clicks and revenue generation compared to those relying solely on traditional advertising techniques.
Customer Acquisition Costs for Both Strategies
Customer acquisition costs (CAC) represent a critical performance indicator in evaluating the effectiveness of both AI-generated ads and traditional marketing strategies. As the climate of marketing and sales evolves, understanding how these costs differ can significantly impact decisions. AI-driven campaigns utilize advanced analytics to create tailored advertisements that directly address the interests of target audiences, often resulting in lower CAC due to more efficient spending on customer outreach.
Conversely, traditional marketing strategies may struggle with higher customer acquisition costs because they often rely on broader and less targeted approaches. In today’s landscape, advertisers who optimize their social media marketing efforts through AI technology can enhance return on investment while maintaining lower CAC. This strategic focus allows for the precise allocation of resources, directly influencing the overall effectiveness of marketing initiatives.
- AI-generated ads employ data analytics for targeted campaigns.
- Lower customer acquisition costs indicate effective use of resources.
- Traditional strategies may lead to higher acquisition costs due to their generalized outreach.
- Optimizing social media marketing enhances customer engagement and reduces costs.
Comparing Customer Lifetime Value Across Approaches
When comparing customer lifetime value (CLV) across AI-generated ads and traditional marketing strategies, efficiency becomes a crucial factor. AI-driven campaigns often utilize advanced analytics to predict consumer behavior, significantly reducing the churn rate by delivering personalized content that resonates with the target audience. For example, a retail brand employing AI tools can identify potential drop-off points and implement strategies to retain customers, thereby increasing their overall CLV and demonstrating a more scalable approach than traditional methods.
Moreover, the integration of technologies such as virtual reality in marketing efforts can enhance customer engagement, leading to higher lifetime value. Scientists in the marketing field note that immersive experiences not only captivate potential buyers but also foster long-term loyalty. By comparing the effectiveness of AI-driven ads that utilize these innovative approaches with traditional ads, organizations can better understand how to allocate their resources effectively to maximize customer lifetime value and ultimately drive their marketing success.
As marketers embrace the precision of AI, traditional methods still hold their ground. Next, explore the unique metrics that reveal the true impact of classic marketing strategies.
Unique Metrics for Traditional Marketing Strategies
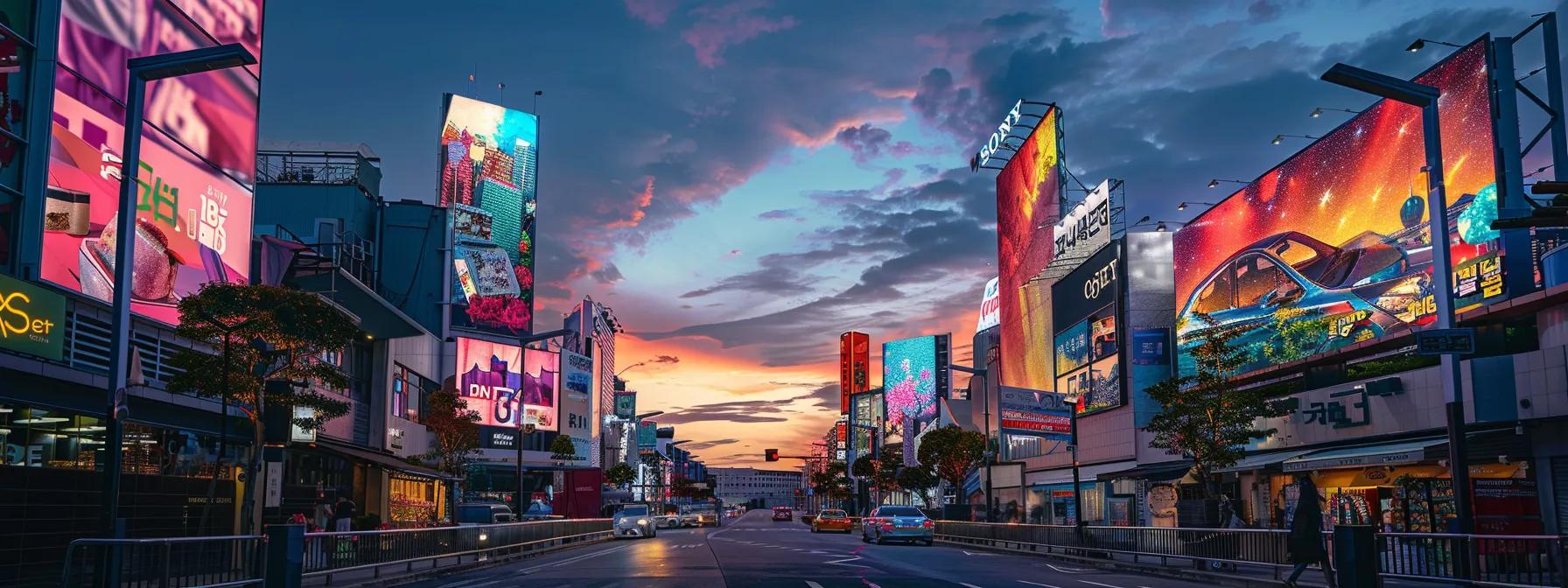
Traditional marketing strategies employ specific metrics for success measurement. Brand awareness and recall measurements gauge how well consumers recognize and remember a brand. Additionally, traditional media reach and frequency metrics help assess audience engagement levels. Offline conversion tracking presents challenges, especially regarding the customer journey, making precise predictions difficult in this context.
These metrics are vital for understanding a campaign‘s personality and long-term sustainability, as they guide advertisers on improving outreach efforts based on insights gathered from IP address tracking and other data sources.
Brand Awareness and Recall Measurements
Brand awareness and recall measurements are crucial for traditional marketing strategies, as they assess how well a campaign resonates with a target audience. Effective social media campaigns often rely on these metrics to gauge the extent of consumer recognition and memory of a brand. Marketers can utilize audience measurement techniques to fine-tune content creation efforts, ensuring that the materials produced effectively capture the attention of potential customers and communicate the intended message.
Evaluating brand awareness involves tracking various factors, such as recognition rates and recall percentages, to interpret the impact of advertising efforts. By leveraging intelligence derived from consumer responses, organizations can adjust their campaigns accordingly, enhancing their outreach and engagement levels. This data-driven approach allows businesses to align their marketing objectives with audience expectations, ultimately leading to more successful advertising outcomes.
Traditional Media Reach and Frequency Metrics
Traditional media reach and frequency metrics play a vital role in assessing the effectiveness of marketing campaigns. By measuring the total audience exposed to an advertisement, advertisers can establish a benchmark for evaluating the campaign‘s overall reach. This ensures that brands maintain credibility by delivering their message consistently across various platforms. For instance, in mobile marketing, understanding how often a target audience sees an advertisement can significantly influence the effectiveness of a campaign, leading to improved attention and engagement.
Moreover, frequency metrics assist businesses in managing their cost per lead more effectively. By analyzing the interaction rates of their ads, marketers can determine the optimal exposure necessary to convert potential customers without oversaturating them. This data-driven approach enables organizations to fine-tune their advertising strategies, ensuring that each campaign reaches its audience adequately, fostering long-term consumer relationships.
- Understanding traditional media reach is crucial for accurate benchmarking.
- Frequency metrics help manage engagement and saturation levels.
- Effective mobile marketing enhances audience attention and recognition.
- Controlling cost per lead contributes to overall campaign efficiency.
- Credibility is maintained through consistent messaging across platforms.
Offline Conversion Tracking Challenges
Offline conversion tracking poses significant challenges for traditional marketing strategies. The difficulty lies in accurately measuring customer responses to advertisements that do not have direct digital feedback mechanisms. For instance, when customers engage with printed media, it becomes nearly impossible to track their journey from seeing an ad to making a purchase, resulting in a gap in data collection and analysis. This limitation hinders marketers’ ability to refine their copywriting techniques and improve their overall campaign effectiveness based on solid data science practices.
Furthermore, inconsistent data collection methods may lead to inaccurate interpretations of campaign success, ultimately affecting customer service efforts. When businesses rely on general assumptions without robust tracking systems in place, they miss valuable insights that could enhance the customer experience. To address these challenges, organizations can implement integrated solutions that connect offline efforts with online metrics, providing a more complete view of customer behavior and facilitating informed strategic adjustments moving forward:
- Accurate measurement difficulties in offline environments.
- The impact of data gaps on campaign optimization.
- Ways to bridge online and offline marketing efforts.
Traditional marketing strategies provide a foundation built on metrics, but the landscape is changing. Real-time data and analytics now drive decisions, reshaping how advertisers connect with their audience.
Real-Time Data and Analytics in AI Advertising
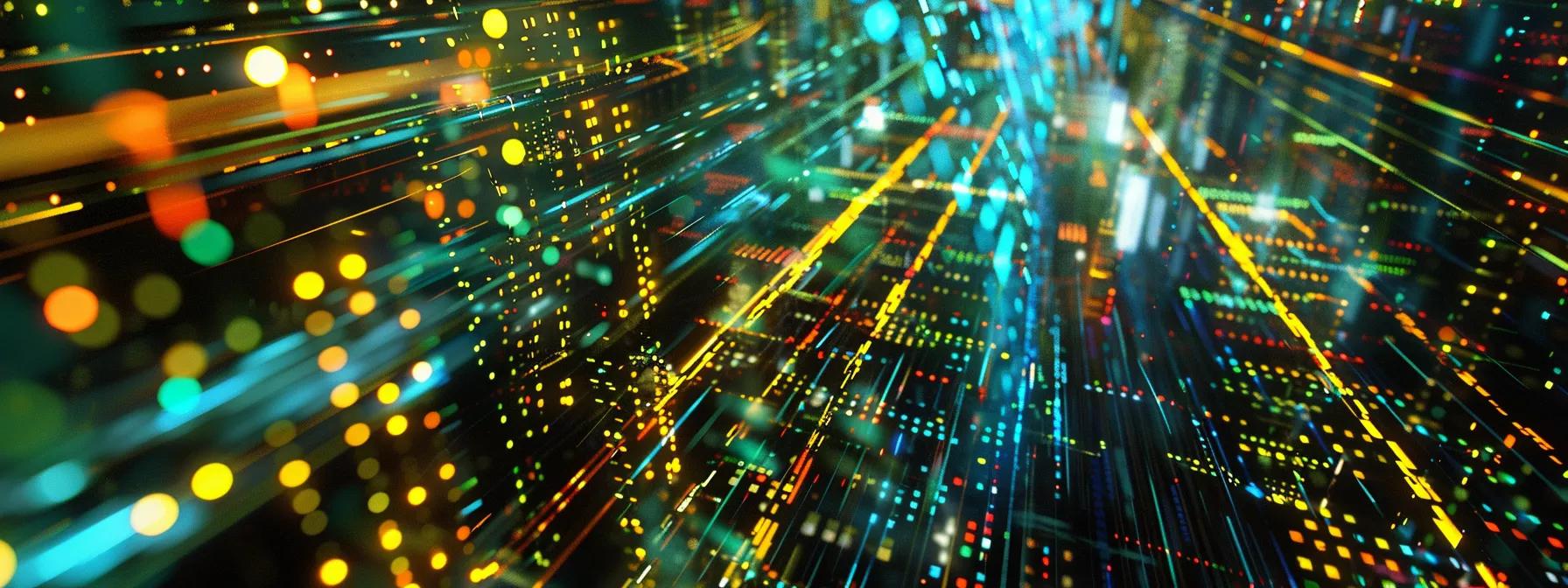
Machine learning plays a vital role in establishing performance metrics for AI-driven advertising, enhancing methodology with real-time data. Automation of reporting processes optimizes resource allocation, ensuring swift access to critical insights. Furthermore, leveraging predictive analytics allows marketers to test hypotheses and improve campaign outcomes, supporting informed decision-making and fostering engagement.
The Role of Machine Learning in Performance Metrics
Machine learning significantly enhances performance metrics in advertising by enabling marketers to analyze consumer data in real time. This capability allows businesses to gain a competitive advantage, as they can quickly adapt their marketing campaigns based on current consumer behavior and preferences. For instance, organizations can fine-tune their strategies to improve customer satisfaction, directly leading to increased advertising revenue through more effective engagement with their target audience.
Moreover, the integration of machine learning into advertising practices facilitates comprehensive research on the factors influencing campaign success. By examining data patterns and trends, marketers can develop insights that drive more targeted campaigns, reducing wasted resources and improving overall performance. This ensures that advertising efforts resonate with potential customers, addressing their needs and enhancing the chances of successful conversions in a fast-paced market environment.
Automating Reporting for AI-Driven Campaigns
Automating reporting for AI-driven campaigns streamlines the assessment process, allowing marketers to obtain insights quickly and efficiently. By harnessing advanced analytics, organizations can capture real-time data across various marketing channels, enhancing the strategies employed by a chief executive officer for improved decision-making. For instance, an AI chatbot can facilitate instant feedback on email marketing campaigns, providing essential metrics that directly influence future content development and audience engagement.
This integration not only saves time but also fosters a culture of data-driven decision-making within the organization. By automating the reporting process, businesses can focus on adapting their marketing strategies instead of getting lost in manual data analysis. Consequently, teams are empowered to improve performance based on accurate, actionable insights, leading to higher conversion rates and better alignment with customer needs:
- Real-time data collection enhances quick decision-making.
- AI chatbots provide immediate feedback on campaign performance.
- Automation cultivates a culture of data-driven practices.
- Streamlined reporting allows for focus on strategy improvement.
- Improved insights lead to higher engagement and conversion rates.
Leveraging Predictive Analytics for Improved Outcomes
Leveraging predictive analytics in digital marketing allows advertisers to create more targeted campaigns by analyzing historical data and consumer behavior patterns. For instance, organizations can utilize neural networks to process large datasets and uncover trends that inform outdoor advertising strategies. This approach ensures that marketing efforts are not only data-driven but also statistically significant, enhancing the likelihood of successful engagement with the intended audience.
As advertisers integrate predictive models into their campaigns, they can optimize various elements, such as ad placement and content effectiveness, ultimately improving conversion rates. The ability to adjust nearly in real-time based on predictive insights allows for more efficient resource allocation, guiding businesses to invest in high-performing strategies while minimizing wasted efforts. By implementing these techniques, marketers position themselves to stay ahead of competition and fully capitalize on opportunities within the market.
Real-time data shapes strategies in AI advertising. Now, let’s look at how success is measured through real examples, revealing what truly works.
Case Studies: Success Measurement in Action
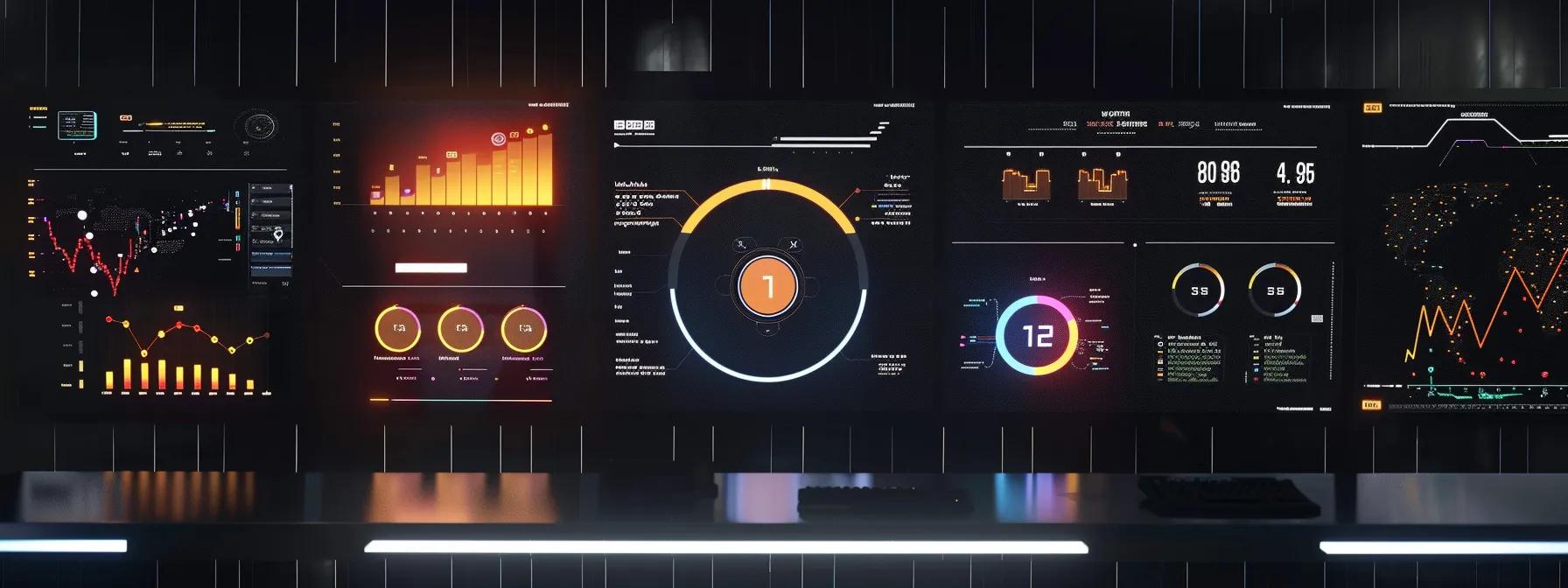
Case Studies: Success Measurement in Action
This section explores notable examples of high-performing AI advertising campaigns that have leveraged web analytics to boost user engagement and drive brand loyalty. It also examines traditional marketing campaigns that set industry benchmarks, focusing on reputation and their impact on consumer behavior. By comparing these approaches, valuable lessons emerge, highlighting effective strategies for maximizing advertising success.
Examples of High-Performing AI Advertising Campaigns
One notable example of a high-performing AI advertising campaign is the initiative by a leading retail brand that employed advanced algorithms to optimize its content marketing strategy. By analyzing gender-based purchasing behaviors, the brand tailored its advertising content to resonate with different audience segments. This approach not only showcased creativity in ad design but also reduced variance in customer engagement and significantly increased conversion rates, highlighting the effectiveness of data-driven insights in contemporary marketing.
Another exemplary AI campaign involved a global travel company that utilized machine learning to refine its targeting policy. By examining historical engagement data, the company customized its ads to align with seasonal trends and consumer preferences, thereby enhancing user experience and loyalty. The ability to dynamically adjust content based on real-time analytics contributed to a measurable uptick in bookings and customer satisfaction, illustrating how AI can effectively address marketer challenges and drive success in digital advertising.
Traditional Marketing Campaigns That Set Benchmarks
Traditional marketing campaigns have historically set benchmarks for success by utilizing various advertising assets across multiple touchpoints. For example, Coca-Cola’s “Share a Coke” campaign effectively personalized their bottles with consumer names, creating a direct connection that enhanced brand engagement. This strategy not only improved profit margins but reduced the risk associated with launching new products by leveraging existing brand loyalty and recognition.
Another example is Procter & Gamble’s “Thank You, Mom” campaign, which aligned with viewers’ emotional touchpoints during major sports events. This advertising campaign demonstrated the value of connecting with consumers on a personal level, resulting in increased brand affinity and profits. By studying these successful traditional approaches, marketers can gather essential insights for measuring campaign effectiveness and risk management that are still relevant in today’s marketing environment.
Lessons Learned From Comparing Both Approaches
Analyzing the performance metrics of AI-generated ads in comparison to traditional marketing strategies reveals critical insights into retention rates. For example, a cosmetics brand that adopted generative artificial intelligence witnessed a significant increase in customer loyalty through personalized marketing campaigns. By leveraging data-driven insights from computer science, this brand effectively targeted customer preferences, which resulted in a notable improvement in repeat purchase behavior, thereby enhancing overall business profitability.
The contrast between these two approaches also emphasizes the importance of agility in adapting marketing strategies. Companies that incorporate machine learning in their advertising efforts can quickly modify campaigns based on audience feedback and engagement analytics. In contrast, traditional methods often struggle to address real-time shifts in consumer behavior, causing potential losses. This reinforces the idea that brands across various industries, including those in accounting and cosmetics, can harness advanced technologies to refine their messaging and strengthen their market position.
The stories of success reveal what truly works in marketing. Now, it is time to shift focus and see how these lessons evolve as strategies change.
Adapting Success Metrics for Evolving Marketing Strategies
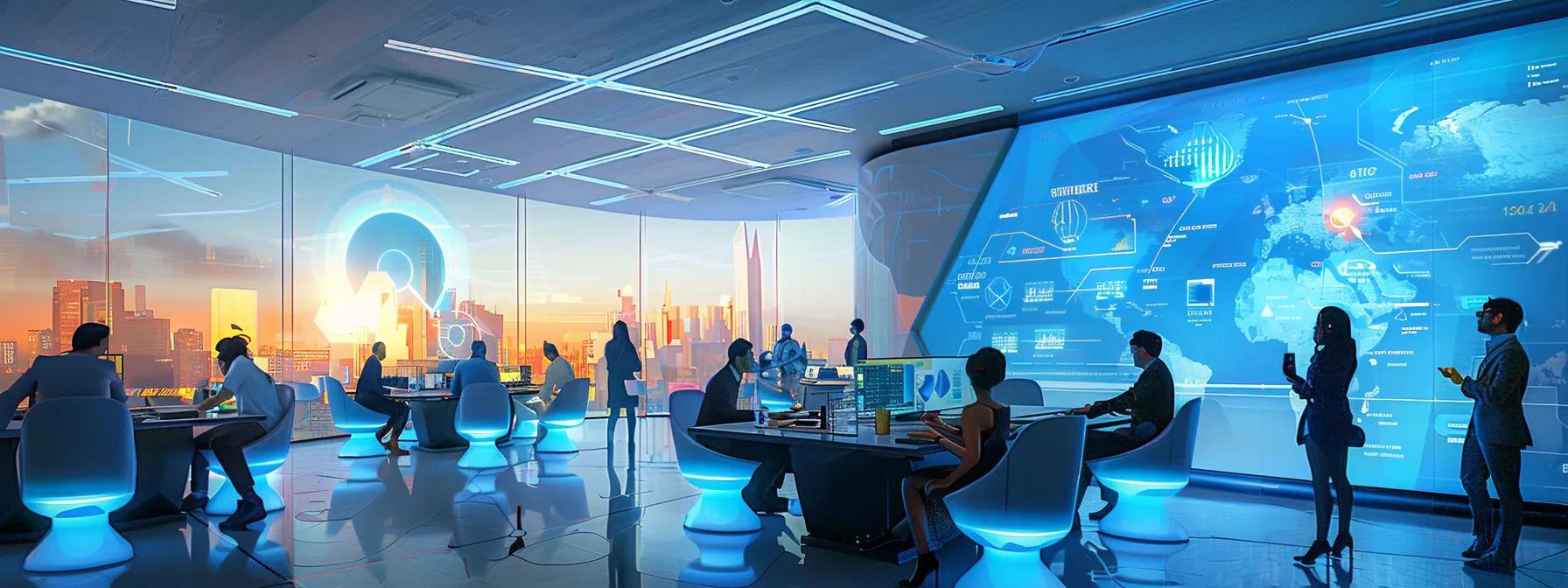
As advertising measurement continues to evolve, understanding future trends becomes vital for marketers. This section focuses on aligning marketing strategies with changing consumer behavior while integrating AI insights for enhanced campaign success. Insights into expense management, open access to data, and an improved customer experience will be explored, providing practical guidance for effective measurement and strategy adaptation.
Future Trends in Advertising Measurement
Future trends in advertising measurement will increasingly focus on the integration of advanced data analysis techniques to enhance return on investment. As marketers seek to optimize their content strategy, the ability to analyze real-time data will empower them to tailor campaigns, making them more relevant to consumers. This shift toward data-driven decision-making will not only boost campaign effectiveness but also elevate brand awareness and customer engagement by ensuring that advertising meets the evolving expectations of the audience.
Organizations will also need to prioritize the customer experience in their measurements, utilizing insights gained from data analysis to refine their advertising approaches continually. The success of campaigns will rely heavily on developing a responsive framework that not only tracks brand awareness but also adjusts creative strategies based on consumer feedback and behavior. This adaptable performance measurement will ensure that businesses remain competitive and effectively connect with their target customers:
- Increased reliance on data analysis to inform marketing decisions.
- Focus on return on investment as a primary metric for success.
- Heightened emphasis on brand awareness as part of overall engagement strategy.
- Continuous refinement of content strategy based on customer insights.
- Prioritization of customer experience to enhance advertising effectiveness.
Aligning Marketing Strategies With Changing Consumer Behavior
Aligning marketing strategies with changing consumer behavior is increasingly necessary for brands aiming to maintain relevance. Machine learning technologies enable advertisers to analyze vast datasets, adapting strategies based on real-time consumer interactions. By leveraging insights derived from platforms like arxiv, marketers can discern evolving trends and preferences, refining their online advertising efforts to enhance customer lifetime value.
As consumer behavior shifts, the importance of visibility in marketing cannot be overstated. Brands must engage with audiences through personalized content that resonates with their specific interests and needs. This proactive alignment not only enhances engagement but also fosters loyalty, ensuring that marketing strategies evolve in tandem with consumer expectations and behaviors:
- Machine learning facilitates real-time analysis of consumer behavior.
- Insights from platforms like arxiv guide marketing adjustments.
- Visibility is crucial for maintaining audience engagement.
- Personalized content increases customer lifetime value.
- Proactive alignment enhances brand loyalty and relevance.
Integrating AI Insights for Enhanced Campaign Success
Integrating AI insights into digital marketing strategies offers significant advantages for enhancing campaign success. By leveraging data analytics, organizations can better understand their potential customers and adjust their messaging accordingly. This approach allows for more accurate targeting, ultimately improving lead generation and reducing the cost per action. As an example, retailers using AI-driven insights can optimize their advertising spend by determining which channels yield the highest returns, thus improving profitability.
The value of incorporating AI insights also lies in the ability to track performance metrics in real time, facilitating immediate adjustments to strategies based on consumer behavior. By continuously analyzing data, businesses can refine their ad placements and content to resonate more effectively with their audience. This adaptability not only enhances customer engagement but also supports long-term loyalty by ensuring that advertising efforts align with the evolving preferences of potential buyers:
- Understanding the importance of accurate targeting for potential customers.
- Leveraging AI for effective lead generation.
- Reducing cost per action through data-driven decisions.
- Optimizing advertising spend to improve profitability.
- Tracking performance in real-time for immediate strategy adjustments.
Conclusion
Comparing key metrics for AI-generated ads and traditional marketing strategies reveals vital differences that drive campaign effectiveness. AI approaches enable real-time data analysis, leading to improved targeting and lower customer acquisition costs, while traditional methods often rely on broader, less adaptive strategies. Understanding these distinctions allows marketers to leverage targeted insights for enhanced engagement and higher conversion rates. Embracing a data-driven mindset is essential for modern advertisers aiming to maximize their marketing impact in an evolving landscape.