Are AI-generated ads truly creative? This question is increasingly relevant as businesses seek innovative ways to enhance their content creation strategies. The blog post will examine the creative potential of AI advertising techniques, compare traditional methods to AI-generated ugc ads, and investigate audience reactions to these new formats. Readers will gain insights into AI’s limitations and capabilities, helping them make informed decisions about incorporating AI into their marketing strategies. By addressing common concerns about personalization and customer experience, this article aims to clarify the role of AI in modern advertising.
Key Takeaways
- AI-generated ads enhance efficiency and targeting through data-driven creativity and adaptability
- Emotional connections in advertising remain vital despite AI’s rapid content generation capabilities
- Transparency in AI processes is crucial for building consumer trust and addressing ethical concerns
- User feedback is essential for refining AI ad effectiveness and ensuring relevant messaging
- Brands must balance AI advancements with human creativity to create emotionally engaging advertisements
Assessing the Creative Potential of AI Advertising Techniques
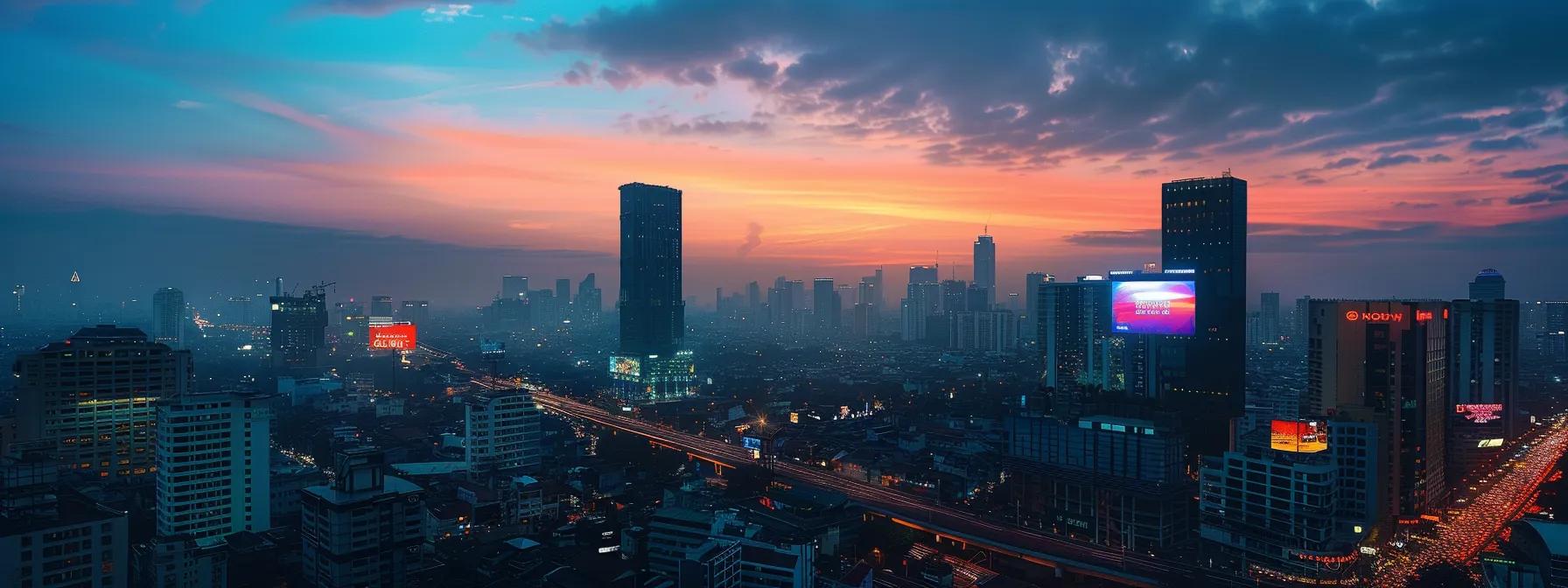
This section delves into the creative capacity of AI in advertising by examining how algorithms generate content, including ugc ads, and identifying key features that drive creativity in ad campaigns. It will present case studies highlighting successful AI-generated advertisements such as ugc ads, analyze the influence of data on ad creativity, and explore the balance between AI and human innovation. Limitations faced by AI in ad creation will also be investigated, emphasizing the broader ecosystem and architecture supporting user experience and audience segmentation.
Understand How AI Algorithms Generate Advertising Content
AI algorithms generate advertising content by analyzing vast datasets to identify trends and consumer preferences. For instance, platforms like Amazon Ads utilize machine learning techniques to create tailored ad copy that resonates with specific target audiences. By leveraging insights from user behavior and engagement, these algorithms can produce targeted and relevant meta ads that align with the specific policies of social media marketing channels.
The effectiveness of AI in copywriting lies in its ability to produce variations of ad content quickly and efficiently, while still maintaining relevancy to the intended audience. This capability allows advertisers to test multiple versions of their ads, fine-tuning them based on performance metrics and user feedback. Such data-driven approaches enhance the creative process, providing marketers with actionable insights to refine their strategies and improve engagement.
Identify Key Features Driving Creativity in AI Ad Campaigns
Key features that drive creativity in AI ad campaigns include innovative algorithms that operate similarly to a chatbot, analyzing consumer behavior to understand preferences and trends. For instance, AI can create engaging video clips by employing animation techniques that reflect user interests, ensuring that the final product is both appealing and relevant to the target audience. This ability to personalize descriptions and visuals enhances creativity, making ads more impactful in online advertising environments.
Furthermore, AI tools benefit from continuous learning, allowing them to refine their outputs based on real-time data and user interactions. This iterative process enables advertisers to make informed decisions quickly, keeping their campaigns current and aligned with market demands. By integrating these features, AI not only complements human creativity but also empowers marketers to implement more effective strategies in their online advertising efforts:
- Utilization of chatbot-like algorithms for trend analysis
- Engaging animation technology to enhance visual appeal
- Personalization of ad descriptions based on user behavior
- Continuous learning from real-time data for campaign refinement
Examine Case Studies of Successful AI-generated Advertisements
One notable experiment in AI-generated advertising is the campaign developed by Lexus, which utilized a neural network to create tailored video ads. By leveraging extensive data analysis of consumer preferences and behaviors, this campaign successfully showcased vehicles in dynamic and eye-catching formats. Such approaches highlight how AI can intelligently select typefaces and visual elements that resonate with target audiences, demonstrating a creative application of technology in advertising.
Another compelling example comes from the clothing retailer H&M, which employed AI to design advertisements that adjusted in real-time based on viewer reactions. This initiative showcased the concept of consent, as it incorporated viewers’ interactions to guide the development of subsequent ads. Using data analysis, the campaign effectively personalized visuals and messaging, proving that AI can enhance creativity while responding to audience preferences in a meaningful way.
Analyze the Role of Data in Shaping Ad Creativity
Data plays a crucial role in shaping ad creativity, driving marketing strategies that effectively engage target audiences. Marketing teams utilize insights derived from consumer interactions and preferences, ensuring that AI-generated ads are not only relevant but also resonate with viewers. By employing techniques such as prompt engineering, marketers can guide AI systems in producing tailored content that minimizes the risk of plagiarism while maximizing the originality and appeal of advertisements.
The landscape of AI advertising is constantly evolving, and leveraging data can significantly enhance creative outcomes. Effective use of analytics allows marketing teams to refine their campaigns in real-time, making adjustments based on audience feedback and performance metrics. This data-driven approach empowers advertisers to craft innovative ad experiences that reflect current trends and consumer desires, resulting in more impactful marketing efforts.
Explore the Balance Between AI and Human Creativity in Ads
The interplay between generative artificial intelligence and human creativity in advertising creates unique opportunities and challenges within digital marketing. Algorithms can efficiently analyze extensive data collection to generate tailored ad content, yet they may lack the nuanced understanding that human creators bring to the table. This dynamic encourages advertisers to foster a collaborative environment where AI tools complement human insights, leading to more compelling and relatable ads that resonate with audiences.
Moreover, the conversation about the balance between AI and human creativity often centers around innovation and audience engagement. While AI can iterate quickly on designs and messaging based on past performance data, the emotional connection and storytelling that humans provide remain essential to crafting truly impactful ads. Advertisers need to recognize that while algorithms offer substantial analytical power, it is the creativity and intuition of human marketers that ultimately drive meaningful connections with consumers.
Investigate Limitations Faced by AI in Ad Creation
One key limitation faced by AI in ad creation is the inherent bias present in algorithms. These biases can stem from the datasets used to train AI systems. For instance, if historical data contains imbalances, the generated social media ads may inadvertently reinforce stereotypes or fail to capture the diversity of potential consumers. This results in missed opportunities for engagement, as ads that do not resonate with a broader audience struggle to gain attention and ultimately impact the overall success ratio of marketing campaigns.
Furthermore, AI-generated ads often lack the emotional depth that human creators bring to their work. While algorithms excel at generating content based on trends and data analytics, they fall short in understanding cultural nuances and emotional connections that drive consumer behavior. This limitation can affect the effectiveness of social media ads, where genuine engagement and relatability are crucial for standing out. To address these challenges, marketers must find ways to complement AI outputs with human creativity, ensuring that their ads not only adhere to principles of search engine optimization but also connect with audiences on a personal level.
AI ads offer new ways to connect, but how do they stack up against traditional methods? A closer look reveals the strengths and weaknesses of each approach, illuminating the path forward for advertisers.
Comparing Traditional Advertising to AI Generated Ads
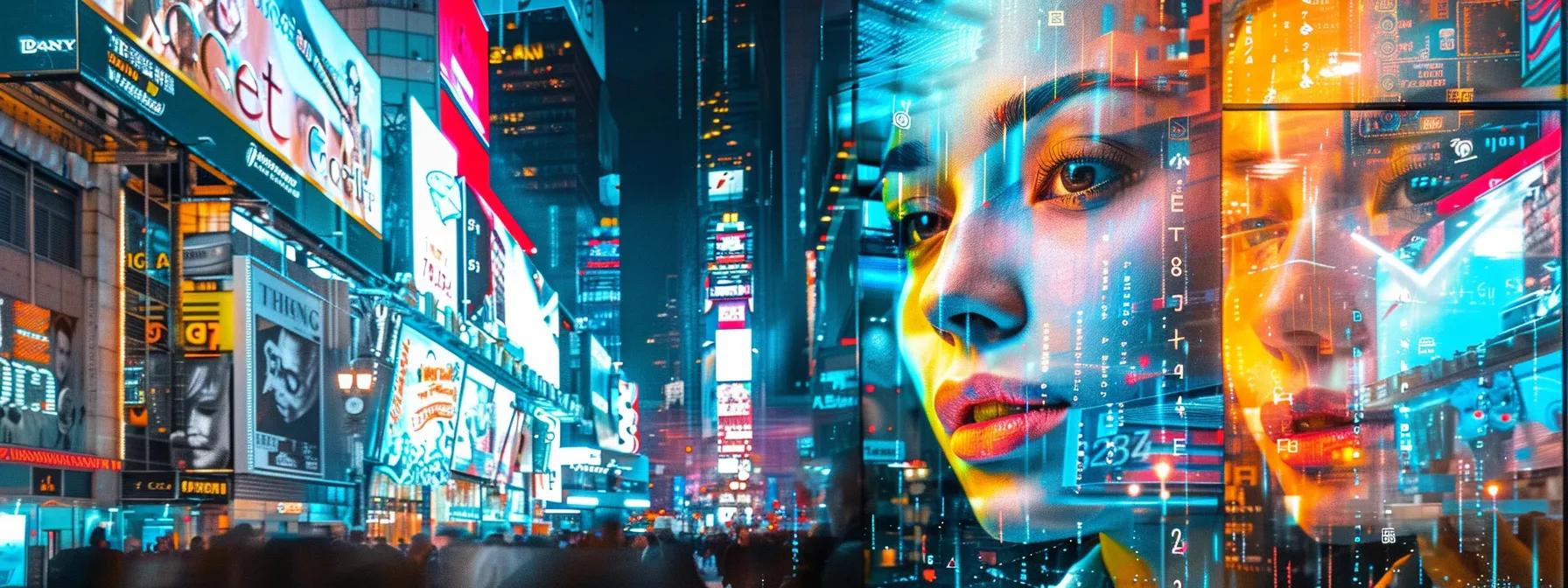
This section will detail the main differences between AI-generated and traditional advertising methods, examining the effectiveness of AI ads compared to conventional strategies. It will explore the challenges of integrating AI into existing advertising systems and identify industries most affected by this technology. Examples of brands benefiting from AI will be presented alongside audience perceptions of AI versus traditional advertising, offering practical insights into their relative strengths.
List the Main Differences Between AI and Traditional Ad Methods
AI-generated ads and traditional advertising methods differ significantly in their communication strategies and execution. Traditional advertising relies heavily on human creativity and intuition to craft messages that resonate with audiences, often stemming from a thorough understanding of cultural nuances. In contrast, AI utilizes deep learning and data analysis to produce ad content, making decisions based on historical data and consumer behavior insights. This shift can enhance campaign performance by rapidly testing various ad variations against real-time metrics, enabling advertisers to pivot strategies more quickly than with conventional methods.
Another critical distinction lies in the potential for revenue generation. While traditional ads may take longer to gauge effectiveness and optimize performance, AI-generated ads can analyze vast datasets to improve performance almost instantaneously. With the ability to produce numerous ad variations efficiently, AI technology facilitates targeted advertising that can lead to better engagement and increased revenue. As a result, brands can leverage AI’s capabilities to drive more effective campaigns and stay ahead in a competitive landscape:
- AI employs deep learning for data-driven decision-making.
- Human creativity in traditional advertising fosters emotional connections.
- Campaign performance is optimized more rapidly with AI.
- Revenue generation can be improved through targeted AI strategies.
Evaluate Effectiveness of AI Ads Versus Conventional Strategies
The effectiveness of AI-generated ads in comparison to traditional strategies is evident in several aspects, particularly in video production and content editing. With the integration of intelligence into advertising, AI systems can quickly analyze vast amounts of data to optimize copy and design, resulting in campaigns that resonate better with target audiences. This rapid adaptation capabilities not only enhance audience engagement but also lead to increased conversion rates, making AI a compelling choice for advertisers seeking effective solutions.
Discuss Challenges of Integrating AI in Existing Advertising Systems
Integrating AI into existing advertising systems presents notable challenges, particularly the risk of algorithmic bias. Such biases can result in ads that may unintentionally favor certain demographics or misrepresent others, leading to ethical concerns and potential backlash in the public sphere. For instance, if an AI system trained on biased data generates an ad that depicts a narrow view of diversity, it could prompt a lawsuit, damaging a brand‘s reputation and customer trust.
Moreover, the shift from traditional graphic design methods to AI-generated content requires a reevaluation of the roles within creative teams. Established roles, such as composers of visual elements, may need to adapt or redefine their functions to align with AI capabilities. This transition can create resistance among team members who may feel displaced by technology, emphasizing the necessity for organizations to foster a collaborative culture that embraces both human creativity and AI efficiency in their advertising strategies.
Highlight Industries Most Affected by AI Advertising
The retail and e-commerce sectors are among the industries that have experienced a significant transformation due to AI advertising tools. With the rise of video content creation refined by AI methodologies, brands can now generate compelling product images that capture consumer attention more effectively. This capability allows retailers to tailor their advertising strategies based on current trends and preferences, enhancing the relevance of their campaigns in a rapidly changing market.
The technology sector is also deeply affected by AI-generated ads, particularly in how brands manage their intellectual property. As companies leverage AI for content creation, they must navigate the complexities of ownership and originality, ensuring that their ads comply with copyright laws while maintaining a unique brand voice. By addressing these challenges, businesses can maximize the advantages of AI in advertising, leading to more engaging campaigns that resonate well with their target audiences.
Present Examples of Brands Benefiting From AI Over Traditional Ads
Brands such as Radiology have harnessed AI advertising tools to drive their marketing strategies effectively. By utilizing data-driven insights, they create tailored advertisements that resonate with their target audience, leading to increased engagement and conversion rates. In this manner, AI-generated content allows Radiology to streamline their campaigns while addressing the specific needs of consumers in the healthcare sector.
Another example can be seen in the application of deepfake technology by leading brands for personalized marketing experiences. Companies leveraging this innovative approach can modify advertisements based on viewer preferences, enhancing user interaction and brand recognition. This adaptability not only highlights the learning curve involved in adopting new technologies but also underscores the competitive edge that AI offers over traditional advertising methods:
- Radiology utilizes AI-driven insights for targeted ads.
- Brands employ deepfake technology for personalized advertising.
- AI demonstrates a learning curve that benefits marketing strategies.
- Customizable content enhances user interaction and brand recognition.
Consider Audience Perceptions of AI Versus Traditional Ads
Audience perceptions of AI-generated ads often hinge on their effectiveness in delivering emotional engagement and achieving a strong return on investment. While AI can produce targeted ads quickly and efficiently, many consumers still value the emotional connection created by traditional advertising methods. Advertisers need to recognize that genuine storytelling and the ability to evoke emotion remain significant drivers of consumer response, which can lead to more sustainable engagement and brand loyalty.
Concerns about copyright infringement also arise in discussions about AI ads, as audiences may question the originality and authenticity of AI-generated content. This skepticism can impact how consumers perceive innovations in advertising, especially in areas like image editing. Advertisers must ensure that their AI tools are used responsibly, adhering to legal standards while creating engaging experiences that resonate with their target audience, fostering trust and enhancing overall campaign effectiveness.
Traditional advertising often relied on guesswork. Now, AI brings a method to the madness, learning how to create ads that speak directly to the audience.
Understanding AI's Learning Process in Ad Creation
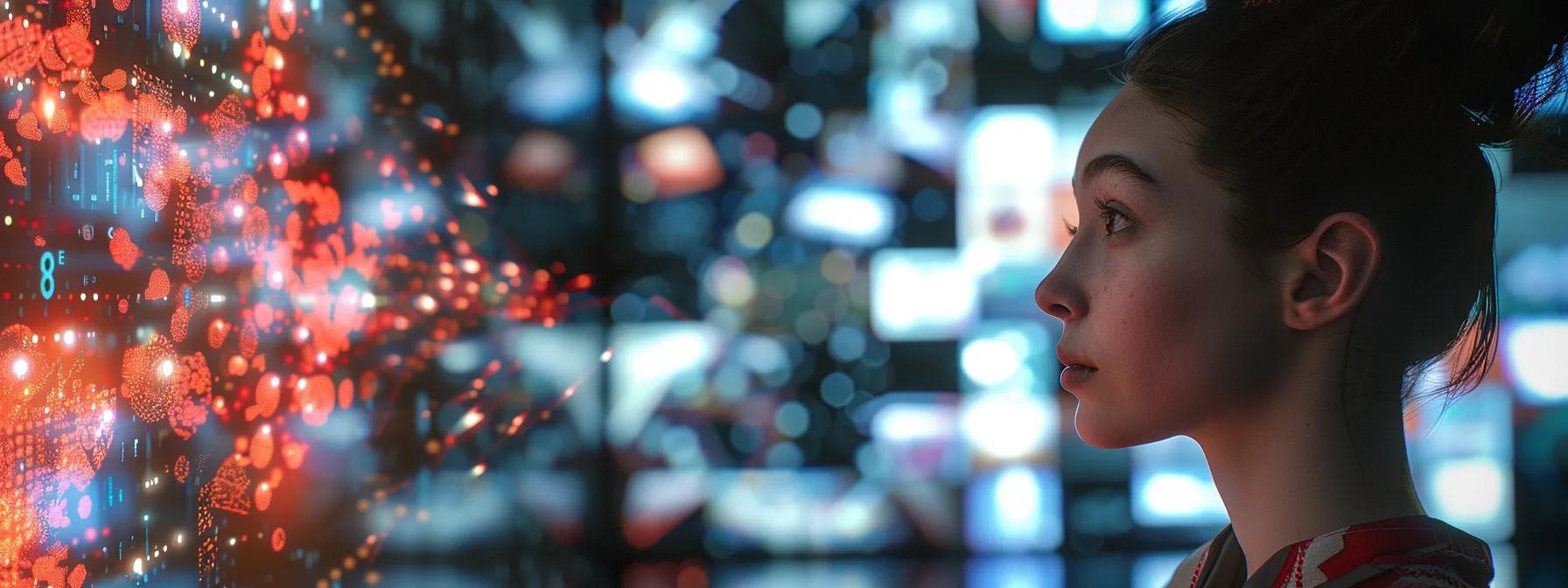
The learning process in AI-generated advertising relies heavily on machine learning techniques, which analyze user data to create effective native ads. User feedback is integral, as analytics inform models that enhance ad performance. This section will cover the mechanisms behind AI training processes, how AI adapts to market trends, and the ongoing evolution within advertising landscapes, providing insights into the risk and potential of AI in advertising.
Explain the Machine Learning Techniques Used in AI Ads
Machine learning techniques play a critical role in the creation of AI-generated ads, with large language models being at the forefront of this process. These models analyze vast amounts of data, including brand interactions and consumer behavior, to identify patterns and trends that inform ad content. By utilizing natural language processing, AI systems can generate relevant and engaging language that resonates with specific audiences, thereby enhancing the effectiveness of advertising strategies.
An example of this technique in action can be seen in how brands capitalize on consumer feedback to refine ad targeting. Through the analysis of user behavior, AI adapts its messaging to align with evolving preferences and interests within the universe of consumer tastes. This dynamic interaction ensures that ads remain relevant and appealing, addressing the real-time needs of the audience while leveraging the capabilities provided by machine learning technology:
- Large language models analyze consumer behavior.
- AI-generated ads use natural language processing for relevance.
- Real-time adaptation enhances ad effectiveness.
Discuss the Importance of User Data for Learning Models
User data plays a pivotal role in shaping the learning models utilized by AI in ad creation, particularly in enhancing automation and improving efficiency across advertising campaigns. By collecting insights from user interactions, AI systems can accurately identify patterns that inform decision-making, enabling the development of ads that resonate with the target audience. For instance, in fields like medical imaging, user data can be used to refine how advertisements communicate complex information, resulting in content that is both informative and engaging.
Effective regulation of AI models requires the incorporation of robust user data to ensure that the content generated meets ethical standards and aligns with consumer expectations. Critical thinking must drive the analysis of this data, guiding marketers to make informed decisions about ad strategies. This systematic approach leads to more relevant advertising experiences, addressing user needs effectively while fostering trust and increasing engagement.
- User data enhances automation and efficiency in AI ad creation.
- Insights from user interactions inform ad development.
- Incorporation of user data is crucial for ethical regulation.
- Critical thinking guides effective analysis and decision-making.
- Relevant ads drive higher user engagement and trust.
Outline the Feedback Mechanisms That Improve AI Ad Performance
Feedback mechanisms play a pivotal role in enhancing the performance of AI-generated ads by continuously refining how these systems respond to customer behaviors and preferences. For instance, using a drag and drop interface, marketers can easily adjust ad elements based on real-time analytics and audience interactions. This technique allows AI to utilize feedback akin to the diffusion of ideas in journalism, rapidly incorporating trends that resonate within a specific genre, thereby improving engagement levels.
Moreover, as customer responses generate data, AI systems adapt their content creation strategies, learning which visuals and messages yield the highest impact. By evaluating various performance indicators, AI can identify and implement successful features from prior campaigns, ensuring that ads evolve with shifting market dynamics. This iterative learning process empowers advertisers to produce more relevant and appealing content, addressing the ongoing needs of their target audience while capitalizing on the capabilities of AI technology.
Investigate the Training Processes Behind AI Advertising Tools
The training process behind AI advertising tools involves complex methodologies that utilize machine learning and computer vision to create relevant ad content. By leveraging vast datasets, these systems learn to identify patterns and consumer preferences, which inform design elements and messaging strategies. For example, a user-friendly interface allows marketers to interact with AI tools, guiding the algorithms in generating ads that resonate with specific audiences, increasing engagement and effectiveness.
This dynamic training process incorporates various concepts from music production, where the iterative adjustment of elements leads to refined outputs. AI tools continuously adapt to feedback and performance metrics, enhancing their ability to generate compelling advertisements. Through this ongoing learning, advertisers can confidently develop campaigns that align with market trends while addressing the needs and interests of consumers:
- Integration of machine learning for pattern recognition.
- Application of computer vision to enhance visual elements.
- Utilization of user-friendly interfaces for marketer interaction.
- Incorporation of feedback and performance data for continuous improvement.
Analyze How AI Adapts to Changing Market Trends in Ads
AI adapts to changing market trends in advertising campaigns by continuously analyzing data related to consumer behavior and preferences. By harnessing real-time analytics, AI systems can modify campaign elements that resonate with the target audience, ensuring relevance amidst evolving trends. This dynamic approach not only enhances the effectiveness of advertisements but also addresses potential misinformation that may arise from outdated strategies.
The ability of AI to engage in brainstorming processes enables marketers to swiftly implement adjustments in their campaigns, fostering a creative and responsive environment. AI-generated content can incorporate trending topics and popular themes, aligning with the interests of consumers while optimizing engagement. As a result, advertisers can confidently navigate the complexities of the market, developing compelling ads that resonate with their audiences and drive overall success.
Review the Ongoing Evolution of AI in Advertising Landscapes
The evolution of AI in the advertising landscape is marked by significant advancements in content marketing strategies driven by machine learning. As algorithms become more sophisticated, they can process vast amounts of data to inform pricing models and optimize campaign performance. This continuous refinement allows advertisers to create targeted content that resonates with their audiences, leading to enhanced engagement and conversion rates.
Furthermore, the integration of AI tools has transformed how marketers approach research and data analysis. By leveraging real-time insights, brands can quickly adapt their advertising campaigns to emerging trends and consumer preferences. This responsiveness not only improves the effectiveness of ads but also empowers marketers to make informed decisions, fostering a more agile advertising environment:
- Advancements in machine learning enhance content marketing strategies.
- Data processing informs pricing models and optimizes campaigns.
- Real-time insights allow quick adaptation to market trends.
- Data analysis fosters informed decision-making within marketing teams.
AI has shifted how ads are made, but the tools behind this change are just as crucial. Next, the focus turns to the must-have AI tools transforming advertising into something fresh and exciting.
Identifying Popular AI Tools for Advertising Innovations
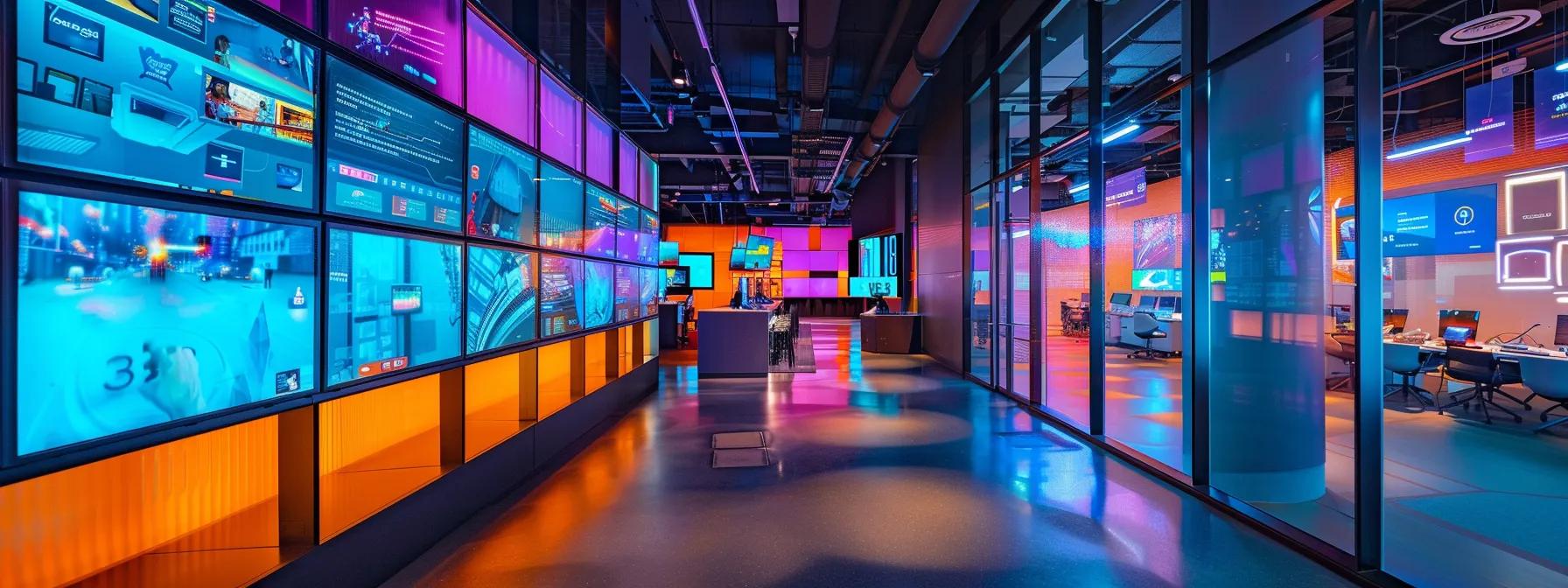
This section identifies leading AI tools commonly used in ad creation, comparing the features of popular platforms like autoencoders that enhance productivity. It examines effective case studies demonstrating the impact of these tools in real-world advertising, reviews user experiences with various applications, and highlights emerging technologies that are reshaping ad creativity. The overview concludes by considering future trends in AI advertising technologies.
List Leading AI Tools Commonly Used in Ad Creation
Several AI tools have gained prominence in the realm of ad creation, significantly transforming how brands develop and execute marketing strategies. Tools such as Adobe Sensei utilize machine learning to enhance creative processes by automating image editing and optimizing ad placements, enabling advertisers to focus on higher-level strategy and engagement. Similarly, platforms like Copy.ai generate compelling ad copy through natural language processing, ensuring that messages resonate well with targeted audiences while minimizing the burden on creative teams.
Another noteworthy tool is Canopy Labs, which harnesses consumer data to create personalized advertisements that appeal to specific market demographics. By analyzing user interactions, this platform allows marketers to refine their ad strategies based on real-time insights and continuously evolving preferences. These advancements illustrate how AI tools not only enhance productivity but also contribute to more effective advertising tactics, addressing the challenges faced by brands in capturing audience attention in a competitive landscape.
Compare Features of Popular AI Advertising Platforms
Popular AI advertising platforms, such as Adobe Sensei and Copy.ai, each offer distinct features designed to enhance the ad creation process. Adobe Sensei utilizes machine learning to automate image editing and optimize ad placements, streamlining workflows for marketers. In contrast, Copy.ai excels in natural language processing, generating effective ad copy that resonates with targeted audiences while reducing the workload for creative teams.
Another noteworthy platform, Canopy Labs, focuses on personalized advertising by leveraging consumer data to create tailored campaigns. By analyzing user interactions, this tool empowers marketers to refine their strategies based on real-time insights. As such, the integration of these AI technologies not only improves productivity but also addresses the evolving needs of brands aiming to capture more audience attention in an increasingly competitive advertising landscape:
- Adobe Sensei automates image editing and ad placements.
- Copy.ai specializes in generating effective ad copy.
- Canopy Labs creates personalized advertisements using user data.
Examine Case Studies Showcasing Tool Effectiveness in Ads
One compelling example of AI tool effectiveness in advertising can be observed in the campaign by KFC, which utilized an AI-driven platform to generate ad content that aligned with customer preferences. By analyzing consumer data, KFC was able to create dynamic advertisements tailored to specific audiences, resulting in a noticeable increase in engagement rates. This case illustrates how AI technologies can empower brands to respond effectively to consumer interests, ultimately improving campaign performance.
Another notable case is the success of promotional campaigns executed by the automotive brand Toyota using AI-generated video ads. By leveraging sophisticated algorithms, Toyota produced targeted video content that addressed individual viewer preferences, leading to higher conversion rates. Such examples demonstrate how integrating AI tools can enhance creativity and effectiveness in advertising by providing insights that drive tailored content creation, thus meeting the needs of diverse audiences.
Review User Experiences With Various AI Advertising Tools
User experiences with various AI advertising tools highlight both the strengths and limitations of these technologies. Many marketers appreciate the efficiency and speed with which AI can generate ad content, allowing them to experiment with different messaging and visuals instantaneously. For instance, platforms like Copy.ai have streamlined the copywriting process, providing users with compelling text options without the lengthy revisions often required with traditional methods.
However, some users express concerns regarding the creativity of AI-generated ads, noting that while these tools can produce relevant content, they may lack the emotional depth of human-created materials. This sentiment is particularly evident in industries where storytelling and emotional connections are crucial for engagement. Marketers are encouraged to balance AI efficiency with their creative insights to ensure that ads resonate meaningfully with their target audiences.
Highlight Emerging Tools That Are Reshaping Ad Creativity
Emerging tools such as Synthesia and Runway are reshaping ad creativity by providing advanced solutions for video production and content generation. Synthesia employs AI to create lifelike avatars that can deliver personalized messages, enabling brands to engage their audiences in a more interactive manner. Meanwhile, Runway utilizes machine learning for video editing, allowing marketers to quickly produce visually compelling content tailored to current trends and consumer preferences.
These innovations mark a significant shift in how advertisers approach campaign development. By leveraging tools that enhance automation and personalization, businesses can streamline their creative processes while maintaining high standards of relevance and engagement. Marketers can capitalize on these capabilities to develop advertising strategies that resonate effectively with diverse audiences and adapt to the rapidly evolving market landscape:
- Synthesia creates lifelike avatars for personalized messaging.
- Runway utilizes machine learning for efficient video editing.
- Advancements facilitate streamlined creative processes.
- Tools enhance automation and personalization in advertising.
Consider the Future Trends in AI Advertising Technologies
As AI advertising technologies evolve, future trends are likely to emphasize greater personalization and interactivity. With advancements in machine learning and data analytics, advertisers can harness user data more effectively to create campaigns that resonate deeply with target audiences. This level of customization has the potential to enhance user engagement, ensuring ads are not only relevant but also tailored to individual preferences.
Moreover, the integration of augmented reality (AR) and virtual reality (VR) in AI advertising is set to transform how consumers experience advertisements. By creating immersive environments, brands can provide users with unforgettable interactions that drive emotional engagement. Such innovations will challenge marketers to blend AI’s data-driven precision with creative storytelling, ultimately pushing the boundaries of what constitutes creativity in advertising.
The tools are in place, ready to shape the future of advertising. Next, the focus shifts to how audiences respond when these new ads meet their eyes.
Evaluating Audience Reactions to AI-Generated Ads
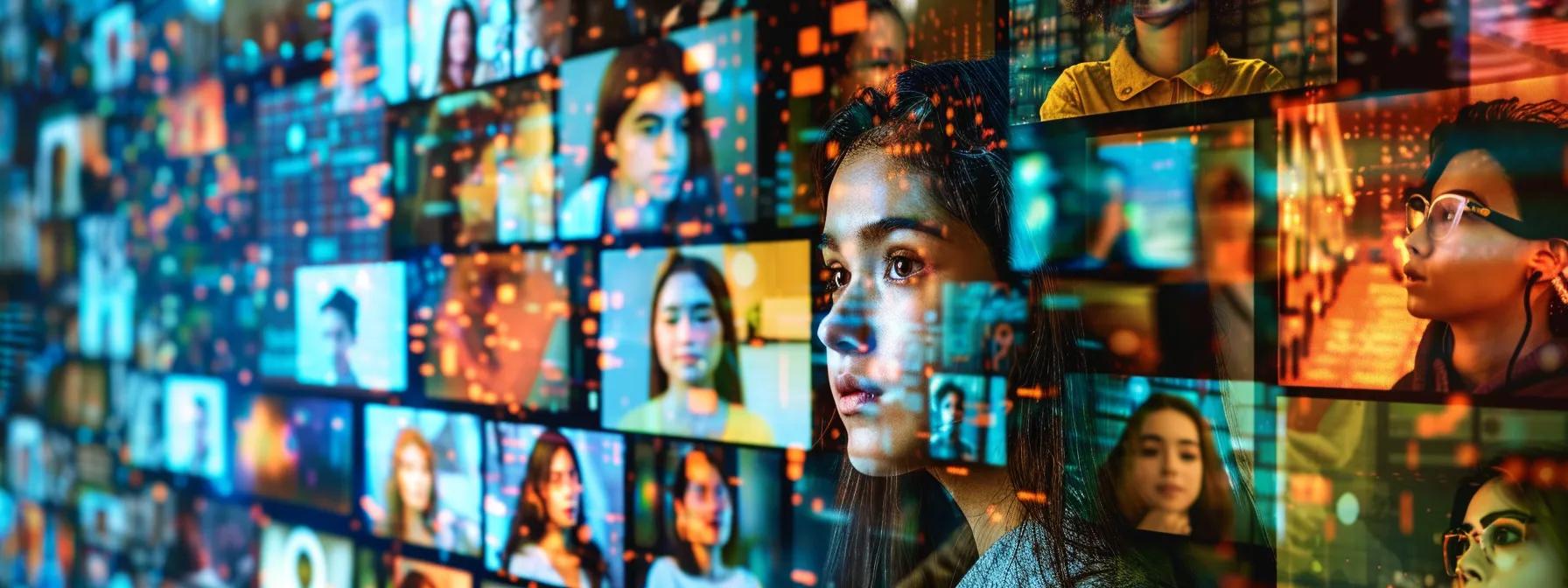
Assessing audience perceptions of AI-generated ads involves examining emotional responses and demographic variances in reactions to AI-created content. Research studies provide insights into how different groups perceive these advertisements and highlight strategies to enhance engagement. Additionally, it is essential to explore potential biases affecting reception and review feedback mechanisms that can refine AI ad effectiveness.
Assess How Audiences Perceive AI-created Advertising
Audiences often exhibit mixed perceptions regarding AI-generated advertising, reflecting both curiosity and skepticism about its effectiveness. While some individuals appreciate the speed and customization AI brings to ad creation, others express concerns about the emotional depth and creativity of these advertisements. Research indicates that audiences may find AI-generated ads less relatable compared to traditionally crafted campaigns that rely on human storytelling and emotional engagement.
Therefore, understanding audience reactions is crucial for optimizing AI ad strategies. Marketers can enhance engagement by integrating feedback mechanisms that assess how different demographics respond to AI-created content. By continuously refining their approaches based on audience insights, advertisers can bridge the gap between AI capabilities and consumer expectations, ensuring that the ads resonate more effectively with targeted audiences:
- Audience perceptions vary, showing curiosity towards AI capabilities.
- Concerns exist about emotional depth in AI-generated ads.
- Feedback mechanisms can improve AI ad effectiveness.
- Refining ad strategies is vital for better engagement.
Analyze Studies on Emotional Responses to AI Ads
Research indicates that audience responses to AI-generated ads frequently reveal a disparity in emotional engagement compared to traditional advertisements. Studies have shown that while AI can produce visually appealing content rapidly, many viewers perceive these ads as lacking the emotional nuance and storytelling depth that human creators provide. This difference in emotional resonance can hinder the effectiveness of AI-generated advertising, as genuine connections often lead to higher consumer engagement and brand loyalty.
Furthermore, experimental studies have explored how demographic factors influence perceptions of AI-generated ads. Younger audiences, who are more accustomed to interacting with technology, may be more accepting of AI in advertising than older demographics, who could be skeptical about its creativity. These variations underscore the importance of understanding audience sentiments and preferences when implementing AI in advertising strategies, as a more informed approach can significantly enhance emotional impact and campaign success.
Discuss Demographic Differences in Reactions to AI Content
Demographic factors significantly influence how audiences react to AI-generated advertisements. Younger consumers, who are often more familiar with technology, tend to exhibit a greater acceptance of AI in marketing. They may appreciate the rapid personalization and relevance of AI-produced content, finding it appealing due to its alignment with their digital experiences. In contrast, older demographics may harbor skepticism regarding the emotional depth of AI-generated ads, perceiving them as less authentic compared to more traditional approaches.
This divergence in reactions underscores the importance of tailoring advertising strategies to different age groups. Marketers can enhance engagement by emphasizing emotional storytelling in ads aimed at older audiences, ensuring that the content resonates on a personal level. Conversely, for younger viewers, highlighting the innovative and interactive aspects of AI-generated content can effectively capture their interest. Understanding these demographic differences allows advertisers to optimize their strategies and create compelling campaigns that connect with diverse audience segments.
Present Strategies for Improving Audience Engagement With AI Ads
To enhance audience engagement with AI-generated ads, brands must prioritize personalization and relevance in their messaging. By analyzing user data and understanding consumer preferences, advertisers can tailor ad content to fit the unique interests of their target demographic. For example, implementing segmentation techniques allows marketers to create customized experiences that resonate on a personal level, thus increasing the likelihood of viewer interaction and brand loyalty.
Furthermore, integrating storytelling techniques can significantly elevate the effectiveness of AI ads. By combining compelling narratives with data-driven content, brands can create ads that evoke emotions and connect with viewers more deeply. This approach not only addresses the need for a human touch in advertising but also ensures that the content remains engaging and memorable. Ultimately, focusing on these strategies can bridge the gap between technological capabilities and consumer expectations, leading to successful advertising outcomes.
Explore Potential Biases in AI-generated Ad Reception
Potential biases in AI-generated ad reception often stem from the datasets used for training algorithms. If these datasets are incomplete or reflect historical imbalances, the resulting ad content may inadvertently reinforce stereotypes or cater to a narrow audience. This can limit engagement and alienate potential consumers, highlighting the importance of diverse and representative datasets to ensure AI outputs resonate broadly.
Moreover, audience perception of AI-generated ads can vary significantly based on cultural and demographic factors. For instance, younger audiences may embrace technological innovations, while older demographics could be skeptical of the emotional depth in AI-created advertisements. Understanding these biases allows marketers to adapt their strategies and create ads that genuinely connect with diverse segments, leveraging AI’s capabilities while addressing the inherent limitations of the technology.
Review Feedback Mechanisms for Enhancing AI Ad Effectiveness
Feedback mechanisms play a crucial role in enhancing the effectiveness of AI-generated advertisements by enabling continuous improvement based on audience responses. By incorporating real-time analytics, marketers can assess how different demographics interact with ads, allowing for timely adjustments that align with viewer preferences. This strategic approach not only increases engagement but also fosters a deeper connection between the brand and the audience, ultimately enhancing the overall effectiveness of the advertising campaign.
In practice, effective feedback loops involve gathering data on key performance indicators and consumer sentiment, which inform subsequent ad iterations. For instance, if an AI-generated video ad receives lower engagement rates in specific demographic segments, marketers can analyze viewer feedback to tweak the ad’s messaging or visuals. This data-driven refinement process ensures that AI ads remain relevant and appealing to target audiences, addressing any shortcomings in creativity and achieving better results within the competitive advertising landscape.
Some ads spark interest, while others raise questions. As audience reactions unfold, it becomes essential to examine the ethical landscape shaping these new advertisements.
Investigating Ethical Considerations in AI Advertising
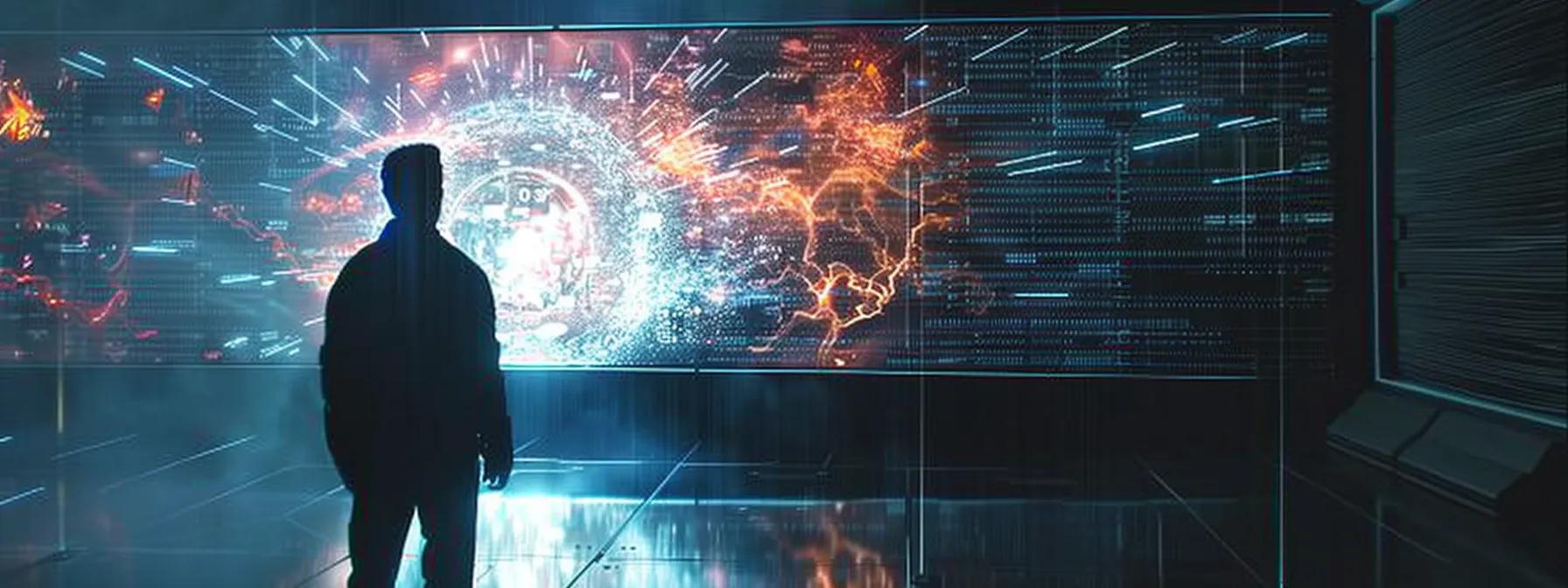
This subsection examines the ethical dilemmas surrounding AI in advertising, focusing on transparency issues in AI ad creation, brand responsibilities when using technology, and consumer privacy concerns. It also evaluates regulatory challenges in AI advertising practices and proposes best practices for ethical approaches. Each topic highlights the necessary considerations for ensuring responsible and effective AI advertising strategies.
Outline Ethical Dilemmas Surrounding AI in Advertising
AI in advertising raises several ethical dilemmas, particularly related to consumer privacy and data usage. Advertisers often rely on extensive user data to inform AI-generated content, raising concerns about how this information is collected and used. The transparency of algorithms that dictate which ads are shown to consumers is also under scrutiny, as hidden biases in these systems can impact audience segmentation and engagement, potentially leading to misrepresentation of diverse consumer groups.
Another significant ethical consideration is the potential for AI-generated content to propagate stereotypes or inadvertently reinforce societal biases. Advertisers must navigate the delicate balance between leveraging AI for creative solutions while ensuring that the resulting ads are respectful and inclusive. By actively seeking to create diverse datasets and implementing ethical guidelines, marketers can work to mitigate these dilemmas and foster a more responsible approach to AI advertising, ultimately enhancing trust and engagement with their audiences.
Discuss Transparency Issues in AI Ad Creation
Transparency issues in AI ad creation pose significant challenges for both advertisers and consumers. As algorithms dictate which advertisements reach specific audiences, there is often a lack of clarity regarding how these decisions are made. This lack of transparency can lead to mistrust among consumers, who may feel targeted by ads that do not reflect their diverse experiences or preferences.
Moreover, the opacity surrounding AI-generated content raises concerns about accountability in marketing practices. When brands rely on AI tools that operate without clear explanations, it becomes difficult to address potential biases embedded in the algorithms. This situation underscores the importance for advertisers to actively seek transparent processes in AI usage, ensuring that their strategies uphold ethical standards and reflect the values of inclusivity and diversity.
Evaluate the Responsibility of Brands Using AI Technology
Brands utilizing AI technology in advertising hold significant responsibility in ensuring that their strategies reflect ethical standards. This involves the mindful use of consumer data, which must be collected and implemented transparently to prevent breaches of privacy. By adopting clear guidelines, brands can foster trust with their audiences, mitigating potential backlash against perceived abuses of technology.
Furthermore, it is crucial for brands to actively review their AI-generated content for biases and stereotypes. Advertisers should prioritize inclusivity and representation in their campaigns, recognizing the impact their messages can have on diverse consumer groups. Engaging in regular audits of AI outputs can help uphold ethical advertising practices while enhancing brand reputation and consumer loyalty:
- Responsibility of brands in ethical data use.
- Transparency in consumer data collection processes.
- Active review of AI-generated content for biases.
- Importance of inclusivity in advertising messages.
- Regular audits for maintaining ethical standards.
Examine Consumer Privacy Concerns With AI Ads
Consumer privacy concerns surrounding AI-generated ads arise primarily from the extensive data collection practices necessary for targeted advertising. As brands utilize sophisticated algorithms to analyze user behavior and preferences, individuals may feel uneasy about how their personal information is being used. The lack of transparency in these processes can lead to mistrust, making it essential for marketers to implement clear data protection measures and communicate them effectively to their audience.
Moreover, AI-generated ads can inadvertently lead to a chilling effect, where consumers may alter their online behavior due to fears of surveillance and data misuse. This apprehension can diminish the effectiveness of advertising campaigns, as the emotional connection to a brand is often weakened by distrust. Advertisers are encouraged to adopt ethical practices that prioritize consumer privacy, thus fostering a more positive relationship and enhancing engagement in the advertising environment.
Highlight Regulatory Challenges in AI Advertising Practices
The regulatory landscape surrounding AI-generated advertisements poses significant challenges for brands and marketers. Current regulations may not fully address the complexities associated with the use of AI, leading to potential issues related to data privacy, algorithmic bias, and transparency in advertising practices. Without clear guidelines, companies might inadvertently produce misleading content or fail to mitigate the ethical implications of their AI systems.
Additionally, as AI technologies continue to evolve, regulatory bodies struggle to keep pace with rapid advancements and innovative advertising techniques. This discrepancy can lead to uneven enforcement of advertising standards, resulting in some brands facing scrutiny while others exploit regulatory gray areas. Consequently, marketers must remain vigilant in adapting their strategies to comply with existing regulations while advocating for updated frameworks that address the unique challenges presented by AI in advertising:
- Data privacy concerns with AI-generated ads.
- Lack of comprehensive regulations related to AI technology.
- Algorithmic bias and its impact on advertising effectiveness.
- Need for transparency in AI advertising processes.
- Challenges in adapting to evolving regulatory landscapes.
Propose Best Practices for Ethical AI Advertising Approaches
Implementing best practices for ethical AI advertising involves ensuring transparency in data usage and algorithmic operations. Brands should clearly communicate how consumer data is collected, processed, and utilized to create ad content, fostering trust among audiences. Regular reviews and audits of AI outputs can help identify biases and stereotypes, leading to more inclusive and responsible advertising strategies that resonate positively with diverse demographics.
Additionally, engaging consumers in the development process enhances ethical standards in AI-generated ads. By soliciting feedback from target audiences on ad effectiveness and emotional engagement, marketers can refine their strategies and improve overall resonance. This focus on consumer insights not only mitigates potential issues related to algorithmic bias but also cultivates a deeper connection between brands and their audiences, ultimately driving successful advertising outcomes.
Conclusion
AI-generated ads represent a significant advancement in advertising technology, showcasing impressive capabilities in tailoring content to consumer preferences. However, their limitations, such as the potential for bias and the lack of emotional depth, highlight the need for a balanced approach that combines AI efficiency with human creativity. Advertisers must prioritize transparency and inclusivity to foster audience trust and engagement, ensuring their strategies resonate effectively across diverse demographics. Recognizing both the strengths and weaknesses of AI in advertising empowers marketers to create more impactful campaigns that leverage technology responsibly and innovatively.