In the fast-paced world of online advertising, many marketers struggle to effectively reach their target audience. Did you know that machine learning can significantly enhance your marketing strategy by improving targeting and personalization? This article will explore how machine learning, particularly techniques like gradient boosting and reinforcement learning, revolutionizes programmatic advertising. We’ll discuss optimizing ad bidding strategies and overcoming implementation challenges. By understanding these concepts, you’ll be better equipped to address common pain points and elevate your advertising efforts.
Key Takeaways
- Machine learning enhances data analysis for more effective targeted advertising strategies
- Personalization improves customer engagement and drives higher conversion rates in advertising campaigns
- Automated bidding processes optimize ad placements and maximize return on investment
- Addressing data privacy is essential for building trust in programmatic advertising
- Continuous improvement and training are crucial for successful machine learning implementation in advertising
Introduction to Machine Learning in Programmatic Advertising
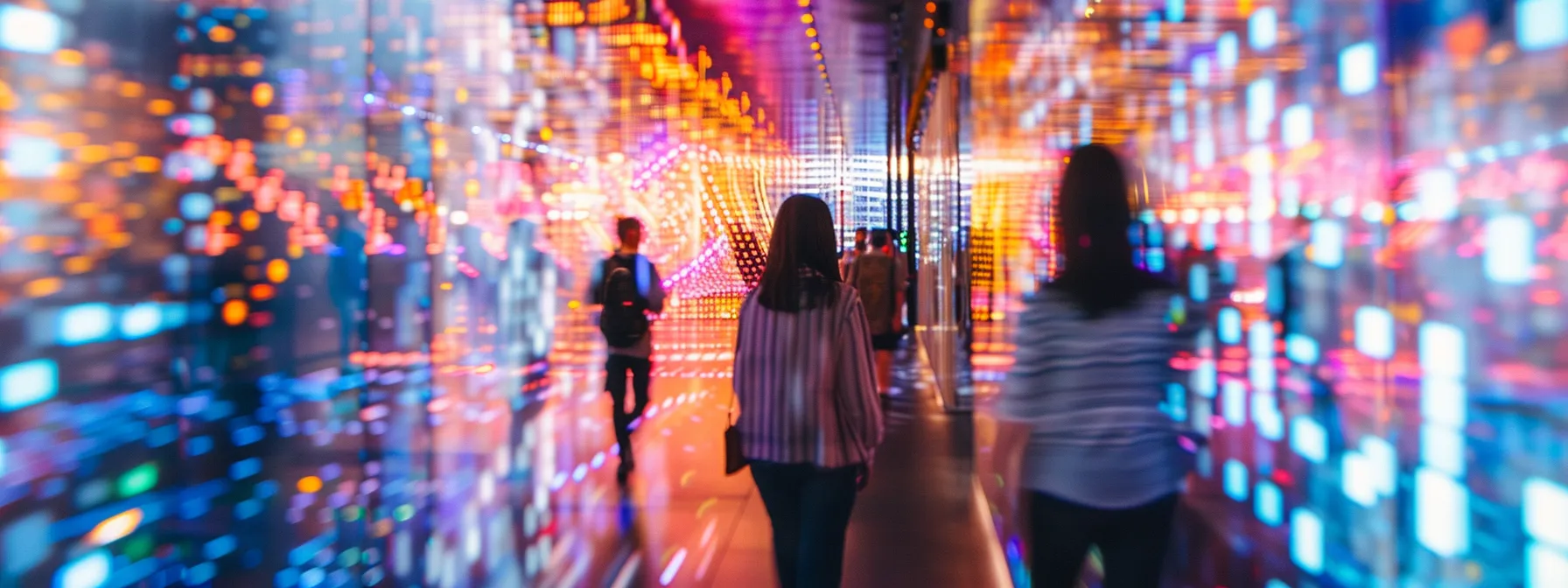
Machine learning is transforming programmatic advertising by enhancing data analysis and data science capabilities. This integration allows organizations to better understand consumer emotions and behaviors, shaping the advertising landscape. In the following sections, I will explore how machine learning integrates with advertising technology and discuss the evolution of advertising through these advancements.
Defining Machine Learning and Programmatic Advertising
Machine learning refers to the use of algorithms and statistical models that enable computers to perform tasks without explicit instructions, relying instead on patterns and inference. In the context of programmatic advertising, this technology analyzes vast amounts of data to understand consumer behavior, allowing for more effective targeted advertising. By leveraging these data models, advertisers can enhance their return on investment through intelligent ad placements that resonate with the right audience at the right time.
How Machine Learning Integrates With Advertising Technology
Machine learning seamlessly integrates with advertising technology by enhancing analytics and research capabilities, allowing advertisers to gain deeper insights into consumer behavior. For instance, in the retail sector, machine learning algorithms can analyze customer engagement data from various channels, including chatbots, to tailor advertising strategies that resonate with specific audiences. This targeted approach not only improves ad effectiveness but also fosters a more personalized shopping experience, ultimately driving higher conversion rates.
The Evolution of Advertising Through Machine Learning
The evolution of advertising through machine learning has been significant, particularly with the rise of unsupervised learning techniques that allow brands to analyze consumer data without predefined labels. This approach enhances audience segmentation by identifying patterns and trends that may not be immediately apparent, enabling advertisers to tailor their strategies more effectively. By focusing on feature engineering, I can extract valuable insights from complex datasets, ultimately improving campaign performance and driving better engagement with target audiences.
Machine learning reshapes how we understand our audience. Now, let’s explore how it sharpens targeting and personalization, making every ad count.
Enhancing Targeting and Personalization
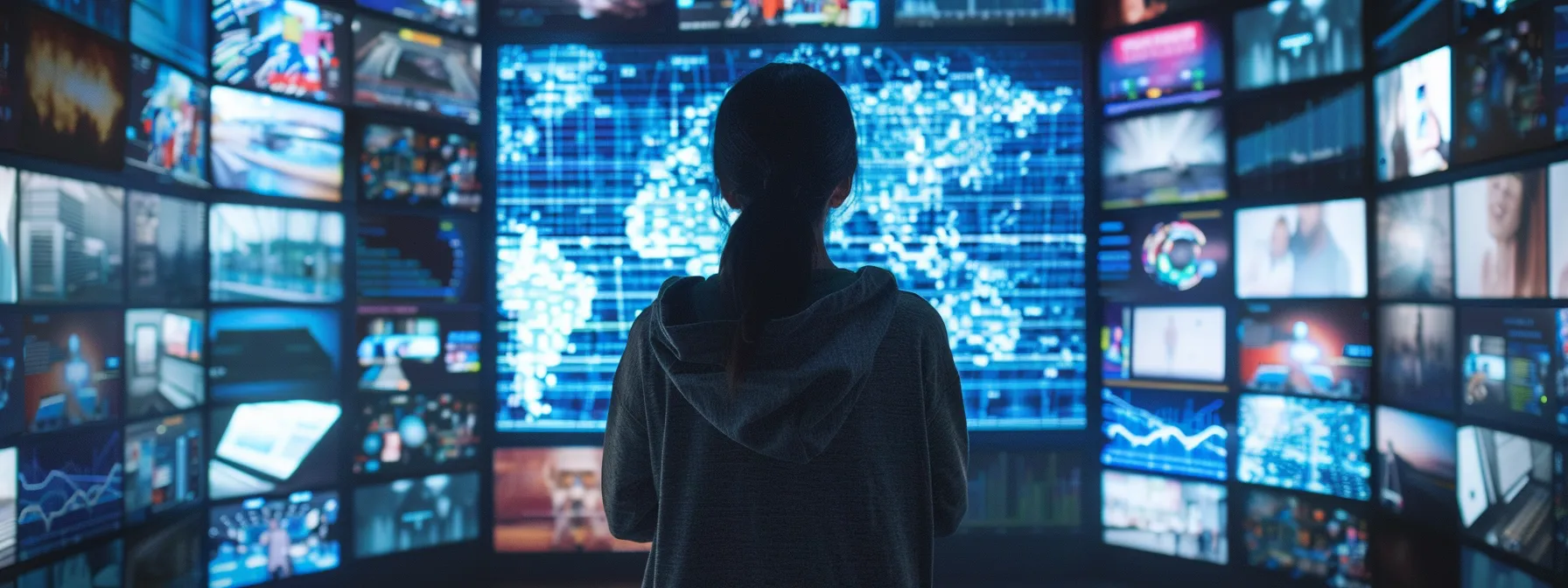
Enhancing Targeting and Personalization
Leveraging user data is essential for precise ad targeting in programmatic advertising. By utilizing natural language processing and marketing automation, I can create tailored advertising campaigns that resonate with consumers. Personalizing ad content not only improves engagement but also helps in identifying lookalike audiences, ultimately driving better results for my advertising efforts.
Leveraging User Data for Precise Ad Targeting
In the realm of programmatic advertising, leveraging user data is crucial for achieving precise ad targeting. By utilizing machine learning algorithms, I can analyze vast datasets to determine the probability of user engagement, ensuring that my ads are relevant to the audience. This data-driven approach not only enhances the effectiveness of my campaigns but also optimizes the adtech ecosystem, allowing for a more personalized experience that resonates with consumers.
- Understanding user behavior through data analysis.
- Utilizing machine learning for improved targeting accuracy.
- Enhancing relevance in advertising campaigns.
- Optimizing the adtech ecosystem for better performance.
Personalizing Ad Content With Machine Learning
Personalizing ad content with machine learning allows me to create highly relevant advertisements that resonate with individual consumers. By utilizing decision tree algorithms, I can analyze user data to understand preferences and behaviors, enabling me to tailor messages that align with their interests. This system not only enhances engagement but also integrates robotic process automation to streamline the ad delivery process, ensuring that the right content reaches the right audience at the optimal time.
Improving Customer Engagement Through Personalization
Improving customer engagement through personalization is a key focus in my programmatic advertising strategy. By utilizing algorithms and predictive analytics, I can analyze user behavior and preferences, allowing me to tailor ads that resonate with my target audience. Incorporating computer vision technology further enhances this process, enabling me to deliver visually appealing content that captures attention and boosts customer satisfaction.
With precise targeting, your ads reach the right eyes. Now, let’s explore how smart bidding can make every dollar count.
Optimizing Ad Bidding Strategies With Machine Learning
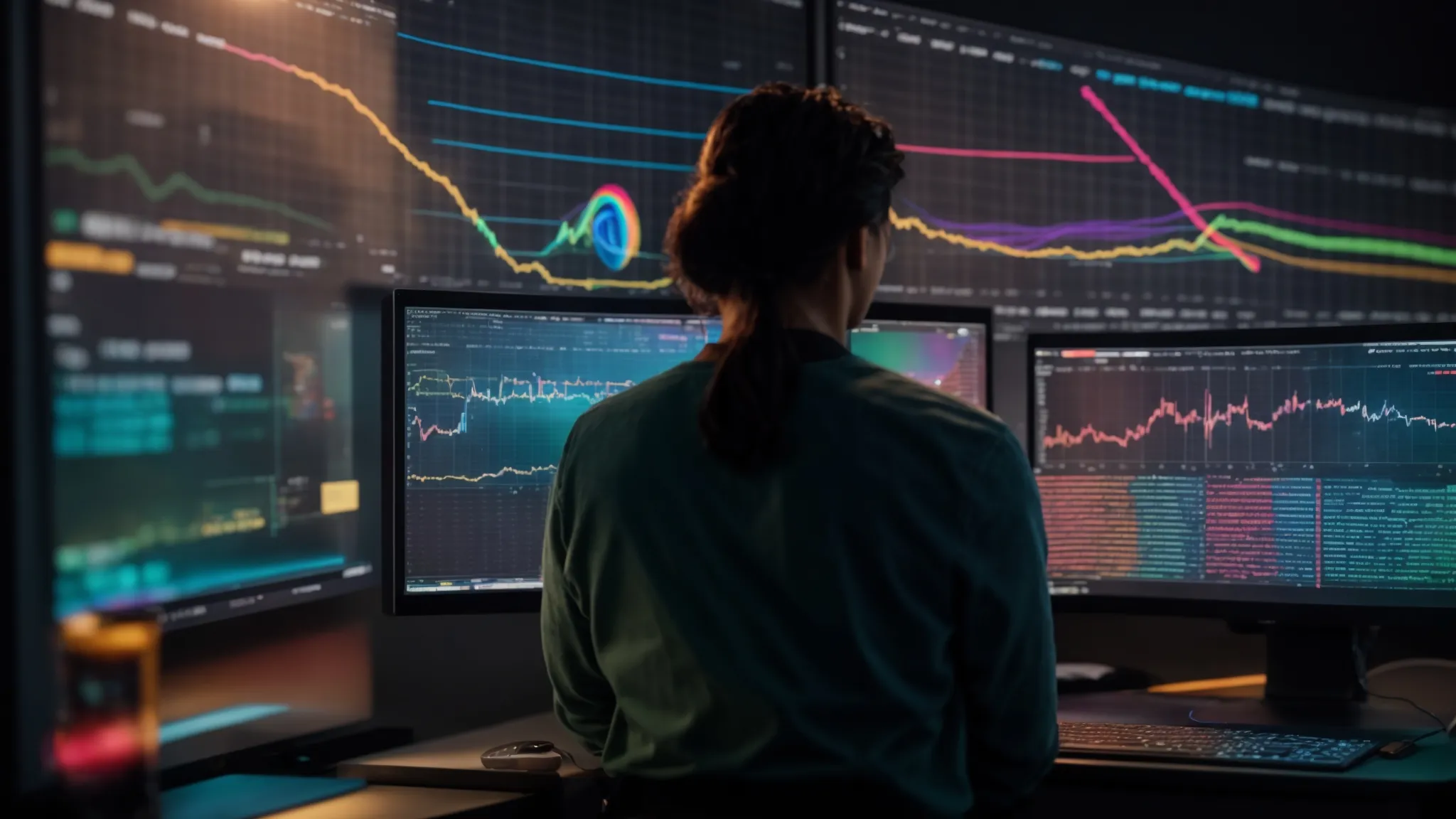
Automated real-time bidding processes are transforming how I manage advertising campaigns, allowing for more efficient media buying. By leveraging predictive analytics in bid optimization, I can make data-driven decisions that maximize ROI. Efficient bidding algorithms enhance my infrastructure, ensuring that my ads reach the right audience through search engine platforms at the right time.
Automated Real-Time Bidding Processes
Automated real-time bidding processes are a game-changer in programmatic advertising, allowing me to make swift, data-driven decisions that enhance campaign performance. By employing supervised learning and reinforcement learning techniques, I can optimize my bidding strategies based on historical data and user behavior, ensuring that my ads are placed effectively. Additionally, incorporating sentiment analysis helps me gauge audience reactions, allowing for adjustments that mitigate bias and improve overall ad relevance.
- Utilizing supervised learning for data-driven bidding decisions.
- Implementing reinforcement learning to optimize strategies.
- Incorporating sentiment analysis to gauge audience reactions.
- Mitigating bias to enhance ad relevance.
Predictive Analytics in Bid Optimization
Predictive analytics plays a crucial role in optimizing bid strategies within programmatic advertising. By analyzing historical data and user behavior, I can anticipate auction outcomes and adjust my bids accordingly to capture audience attention effectively. This data-driven approach not only enhances the user experience but also streamlines content creation, ensuring that the right ads reach the right users at the right time.
- Utilizing historical data for informed bidding decisions.
- Anticipating auction outcomes to capture audience attention.
- Enhancing user experience through targeted ad placements.
- Streamlining content creation for better engagement.
Maximizing ROI With Efficient Bidding Algorithms
Maximizing ROI with efficient bidding algorithms is essential in programmatic advertising, where every touchpoint matters. By employing deep learning techniques and logistic regression models, I can analyze vast datasets to identify the most effective bidding strategies tailored to my audience’s behavior. This data-driven approach not only enhances my martech capabilities but also ensures that my ads are placed at the right time and in front of the right users, ultimately driving better returns on my advertising investments:
- Utilizing deep learning for advanced data analysis.
- Implementing logistic regression to refine bidding strategies.
- Enhancing martech tools for improved campaign performance.
- Focusing on touchpoints to maximize audience engagement.
Even as we refine our ad bidding strategies, challenges lurk in the shadows. Understanding these obstacles is key to harnessing the true power of machine learning.
Overcoming Challenges in Machine Learning Implementation
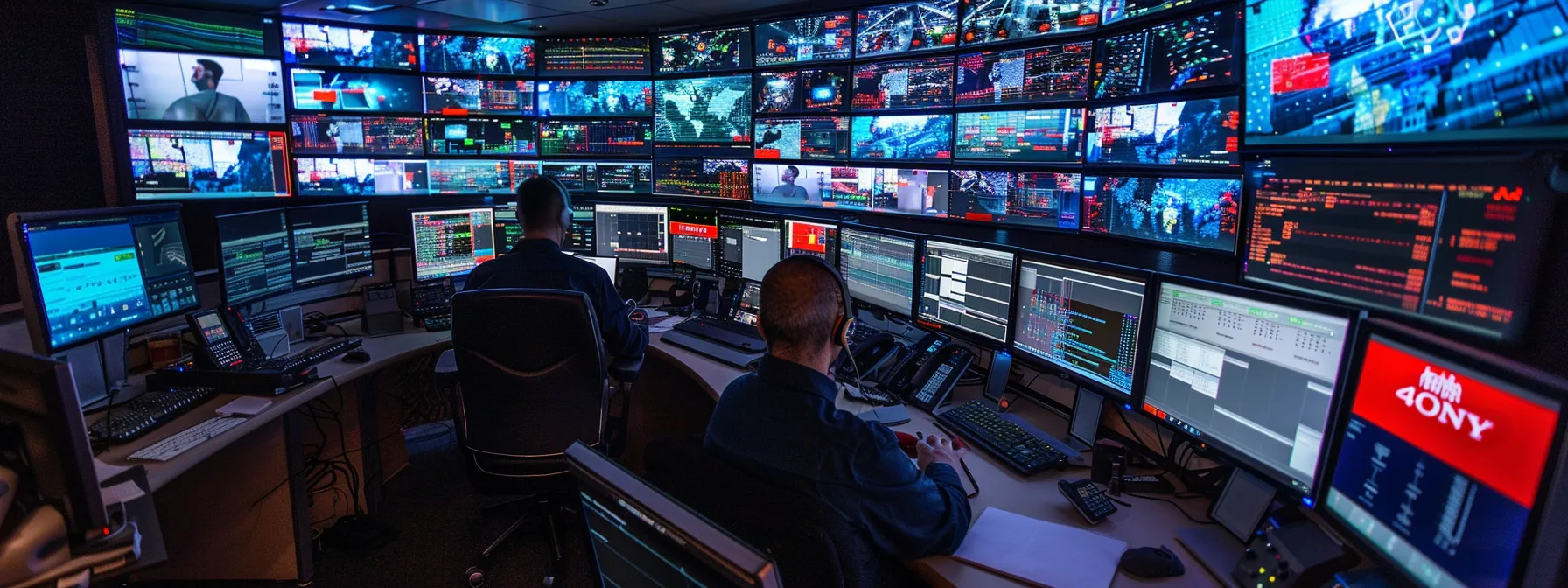
Addressing data privacy and security concerns is crucial as I integrate machine learning into existing systems. I must ensure transparency and accountability in algorithms while managing unstructured data effectively. In the following sections, I will discuss how training models can enhance customer lifetime value, the demand for speech recognition technology, and the strategies to overcome these challenges in programmatic advertising.
Addressing Data Privacy and Security Concerns
As I integrate machine learning into programmatic advertising, addressing data privacy and security concerns becomes paramount. With the increasing reliance on social media and user data, I must ensure that my workflow complies with regulations while maintaining a positive customer experience. By leveraging statistics to analyze data responsibly, I can build trust with my audience, ensuring that my advertising strategies are both effective and ethical.
Integrating Machine Learning Into Existing Systems
Integrating machine learning into existing systems can be a complex process, but it is essential for enhancing personalization in programmatic advertising. For instance, I often utilize random forest algorithms to analyze customer data, which helps me create more effective contextual advertising strategies. By implementing these advanced techniques within mobile apps, I can deliver tailored content that resonates with users, ultimately improving engagement and driving conversions.
- Utilizing random forest algorithms for data analysis.
- Enhancing personalization in programmatic advertising.
- Implementing machine learning in mobile apps.
- Improving contextual advertising strategies.
Ensuring Transparency and Accountability in Algorithms
Ensuring transparency and accountability in algorithms is essential for building trust in programmatic advertising, especially when handling personal data. I focus on implementing clear guidelines that govern how algorithms make predictions, which not only enhances productivity but also safeguards user privacy. By openly sharing how UGC Ads are targeted and the rationale behind ad placements, I can demonstrate a commitment to ethical practices that ultimately drive revenue while fostering a positive relationship with consumers.
The challenges faced in machine learning are just the beginning. As we look ahead, programmatic advertising promises to change the game, offering new ways to connect and engage.
The Future of Programmatic Advertising

Emerging trends in machine learning are reshaping programmatic advertising, particularly through the development of advanced recommender systems that enhance customer retention. I will explore how AI advancements, including artificial neural networks, are revolutionizing ad technology and improving email marketing strategies. Additionally, I will discuss how these innovations prepare us for the next generation of advertising solutions, focusing on effective copywriting and targeted outreach.
Emerging Trends in Machine Learning and Advertising
As I look toward the future of programmatic advertising, emerging trends in machine learning are set to redefine how we approach search ads and product promotion. By leveraging advanced algorithms, I can analyze user preferences more effectively, allowing for the creation of tailored assets that resonate with specific audiences. This targeted approach not only enhances engagement but also plays a crucial role in reducing churn rates, ensuring that my advertising strategies remain relevant and effective in a competitive landscape.
The Impact of AI Advancements on Ad Technology
The impact of AI advancements on ad technology is profound, particularly in enhancing search engine optimization and improving landing page effectiveness. By utilizing platforms like Criteo, I can leverage machine learning algorithms to analyze user behavior and optimize ad frequency, ensuring that my content marketing strategies are both targeted and efficient. This approach not only increases engagement but also drives conversions, as I can deliver personalized content that resonates with my audience’s preferences and needs:
- Utilizing AI for enhanced search engine optimization.
- Improving landing page effectiveness through data analysis.
- Leveraging Criteo for optimized ad frequency.
- Driving conversions with targeted content marketing strategies.
Preparing for the Next Generation of Advertising Solutions
As I prepare for the next generation of advertising solutions, I recognize the importance of integrating advanced machine learning architectures into our existing database systems. This integration not only enhances customer service by providing real-time insights but also mitigates risks associated with ad placements and pricing strategies. By leveraging data-driven approaches, I can optimize our advertising efforts, ensuring that we deliver relevant content to the right audience while maintaining cost efficiency.
The landscape of advertising is changing fast, and those who adapt will thrive. Next, we’ll explore how to weave machine learning into your strategy for better results.
Implementing Machine Learning in Your Advertising Strategy

Implementing Machine Learning in Your Advertising Strategy
To effectively implement machine learning in my advertising strategy, I start by assessing my business needs and objectives. This helps me identify the right machine learning tools that align with my goals. Training my team for technological adoption is crucial, as is measuring success and fostering continuous improvement. Each of these steps ensures that I maximize the benefits of machine learning in programmatic advertising.
Assessing Your Business Needs and Objectives
To effectively implement machine learning in my advertising strategy, I begin by thoroughly assessing my business needs and objectives. This process involves identifying specific goals, such as improving ad targeting or increasing conversion rates, which helps me determine the most suitable machine learning tools and techniques. By aligning these tools with my objectives, I can ensure that my programmatic advertising efforts are not only efficient but also tailored to meet the unique demands of my audience.
Choosing the Right Machine Learning Tools
Choosing the right machine learning tools is essential for optimizing my programmatic advertising strategy. I focus on tools that offer robust data analysis capabilities and integrate seamlessly with my existing systems. For instance, platforms like TensorFlow and Scikit-learn provide the flexibility to build custom models tailored to my specific advertising needs, enabling me to enhance targeting accuracy and improve campaign performance.
Training Your Team for Technological Adoption
Training my team for technological adoption is a critical step in successfully implementing machine learning in our advertising strategy. I focus on providing comprehensive training sessions that cover the fundamentals of machine learning, data analysis, and the specific tools we will be using. By fostering a culture of continuous learning and encouraging team members to engage with new technologies, I ensure that everyone is equipped to leverage machine learning effectively, ultimately enhancing our programmatic advertising efforts.
- Provide comprehensive training on machine learning fundamentals.
- Focus on data analysis and specific tools for implementation.
- Encourage a culture of continuous learning within the team.
- Equip team members to leverage machine learning effectively.
Measuring Success and Continuous Improvement
Measuring success and fostering continuous improvement are critical components of my machine learning strategy in programmatic advertising. I regularly analyze key performance indicators (KPIs) such as click-through rates, conversion rates, and return on ad spend to assess the effectiveness of my campaigns. By leveraging machine learning algorithms, I can identify trends and patterns in the data, allowing me to make informed adjustments that enhance campaign performance and drive better results:
- Regularly analyze key performance indicators (KPIs).
- Utilize machine learning algorithms to identify trends.
- Make informed adjustments to enhance campaign performance.
- Drive better results through continuous improvement.
Conclusion
Machine learning is fundamentally transforming programmatic advertising by enabling precise targeting and personalization, which enhances consumer engagement and drives higher conversion rates. By leveraging advanced algorithms, advertisers can analyze vast datasets to optimize bidding strategies and improve campaign performance, ultimately maximizing return on investment. Addressing challenges such as data privacy and integration ensures that these technologies are implemented ethically and effectively. As we move forward, embracing machine learning will be essential for staying competitive in the ever-evolving digital advertising landscape.